Prospects for inferring very large phylogenies by using the neighbor-joining method
- PMID: 15258291
- PMCID: PMC491989
- DOI: 10.1073/pnas.0404206101
Prospects for inferring very large phylogenies by using the neighbor-joining method
Abstract
Current efforts to reconstruct the tree of life and histories of multigene families demand the inference of phylogenies consisting of thousands of gene sequences. However, for such large data sets even a moderate exploration of the tree space needed to identify the optimal tree is virtually impossible. For these cases the neighbor-joining (NJ) method is frequently used because of its demonstrated accuracy for smaller data sets and its computational speed. As data sets grow, however, the fraction of the tree space examined by the NJ algorithm becomes minuscule. Here, we report the results of our computer simulation for examining the accuracy of NJ trees for inferring very large phylogenies. First we present a likelihood method for the simultaneous estimation of all pairwise distances by using biologically realistic models of nucleotide substitution. Use of this method corrects up to 60% of NJ tree errors. Our simulation results show that the accuracy of NJ trees decline only by approximately 5% when the number of sequences used increases from 32 to 4,096 (128 times) even in the presence of extensive variation in the evolutionary rate among lineages or significant biases in the nucleotide composition and transition/transversion ratio. Our results encourage the use of complex models of nucleotide substitution for estimating evolutionary distances and hint at bright prospects for the application of the NJ and related methods in inferring large phylogenies.
Figures
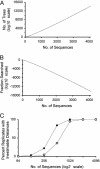
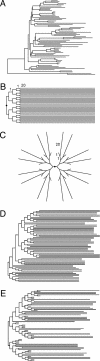
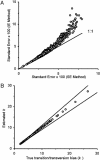
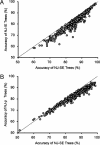
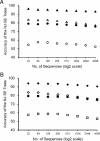
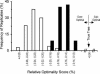
Similar articles
-
ML or NJ-MCL? A comparison between two robust phylogenetic methods.Comput Biol Chem. 2009 Oct;33(5):373-8. doi: 10.1016/j.compbiolchem.2009.07.007. Epub 2009 Jul 19. Comput Biol Chem. 2009. PMID: 19679513
-
Inferring species phylogenies from multiple genes: concatenated sequence tree versus consensus gene tree.J Exp Zool B Mol Dev Evol. 2005 Jan 15;304(1):64-74. doi: 10.1002/jez.b.21026. J Exp Zool B Mol Dev Evol. 2005. PMID: 15593277
-
Evaluating the relationship between evolutionary divergence and phylogenetic accuracy in AFLP data sets.Mol Biol Evol. 2010 May;27(5):988-1000. doi: 10.1093/molbev/msp315. Epub 2009 Dec 21. Mol Biol Evol. 2010. PMID: 20026482
-
Neighbor-joining revealed.Mol Biol Evol. 2006 Nov;23(11):1997-2000. doi: 10.1093/molbev/msl072. Epub 2006 Jul 28. Mol Biol Evol. 2006. PMID: 16877499 Review.
-
Phylogenetic analysis in molecular evolutionary genetics.Annu Rev Genet. 1996;30:371-403. doi: 10.1146/annurev.genet.30.1.371. Annu Rev Genet. 1996. PMID: 8982459 Review.
Cited by
-
Evaluation of biofilm formation and antimicrobial susceptibility (drug resistance) of Candida albicans isolates.Braz J Microbiol. 2024 Nov 6. doi: 10.1007/s42770-024-01558-w. Online ahead of print. Braz J Microbiol. 2024. PMID: 39500825
-
Molecular Evolution of Human Norovirus GII.2 Clusters.Front Microbiol. 2021 Mar 22;12:655567. doi: 10.3389/fmicb.2021.655567. eCollection 2021. Front Microbiol. 2021. PMID: 33828543 Free PMC article.
-
Fungal Community Successions in Rhizosphere Sediment of Seagrasses Enhalus acoroides under PAHs Stress.Int J Mol Sci. 2015 Jun 18;16(6):14039-55. doi: 10.3390/ijms160614039. Int J Mol Sci. 2015. PMID: 26096007 Free PMC article.
-
Epidemiology and genetic diversity of SARS-CoV-2 lineages circulating in Africa.medRxiv [Preprint]. 2021 May 19:2021.05.17.21257341. doi: 10.1101/2021.05.17.21257341. medRxiv. 2021. Update in: iScience. 2022 Mar 18;25(3):103880. doi: 10.1016/j.isci.2022.103880 PMID: 34031660 Free PMC article. Updated. Preprint.
-
Spatial variations of bacterial community and its relationship with water chemistry in Sanya Bay, South China Sea as determined by DGGE fingerprinting and multivariate analysis.Ecotoxicology. 2015 Oct;24(7-8):1486-97. doi: 10.1007/s10646-015-1492-y. Epub 2015 May 27. Ecotoxicology. 2015. PMID: 26013101
References
Publication types
MeSH terms
LinkOut - more resources
Full Text Sources
Other Literature Sources
Research Materials