Application of independent component analysis to microarrays
- PMID: 14611662
- PMCID: PMC329130
- DOI: 10.1186/gb-2003-4-11-r76
Application of independent component analysis to microarrays
Abstract
We apply linear and nonlinear independent component analysis (ICA) to project microarray data into statistically independent components that correspond to putative biological processes, and to cluster genes according to over- or under-expression in each component. We test the statistical significance of enrichment of gene annotations within clusters. ICA outperforms other leading methods, such as principal component analysis, k-means clustering and the Plaid model, in constructing functionally coherent clusters on microarray datasets from Saccharomyces cerevisiae, Caenorhabditis elegans and human.
Figures
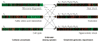
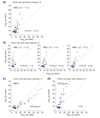
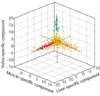
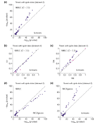
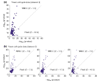
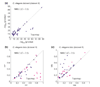
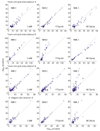
Similar articles
-
Decomposing gene expression into cellular processes.Pac Symp Biocomput. 2003:89-100. doi: 10.1142/9789812776303_0009. Pac Symp Biocomput. 2003. PMID: 12603020
-
Influence of microarrays experiments missing values on the stability of gene groups by hierarchical clustering.BMC Bioinformatics. 2004 Aug 23;5:114. doi: 10.1186/1471-2105-5-114. BMC Bioinformatics. 2004. PMID: 15324460 Free PMC article.
-
Possibilistic approach for biclustering microarray data.Comput Biol Med. 2007 Oct;37(10):1426-36. doi: 10.1016/j.compbiomed.2007.01.005. Epub 2007 Mar 8. Comput Biol Med. 2007. PMID: 17346690
-
Discovering patterns in microarray data.Mol Diagn. 2000 Dec;5(4):349-57. doi: 10.1007/BF03262096. Mol Diagn. 2000. PMID: 11172499 Review.
-
Clustering methods for microarray gene expression data.OMICS. 2006 Winter;10(4):507-31. doi: 10.1089/omi.2006.10.507. OMICS. 2006. PMID: 17233561 Review.
Cited by
-
K1 and K15 of Kaposi's Sarcoma-Associated Herpesvirus Are Partial Functional Homologues of Latent Membrane Protein 2A of Epstein-Barr Virus.J Virol. 2015 Jul;89(14):7248-61. doi: 10.1128/JVI.00839-15. Epub 2015 May 6. J Virol. 2015. PMID: 25948739 Free PMC article.
-
Integrating genome-wide genetic variations and monocyte expression data reveals trans-regulated gene modules in humans.PLoS Genet. 2011 Dec;7(12):e1002367. doi: 10.1371/journal.pgen.1002367. Epub 2011 Dec 1. PLoS Genet. 2011. PMID: 22144904 Free PMC article.
-
robustica: customizable robust independent component analysis.BMC Bioinformatics. 2022 Dec 5;23(1):519. doi: 10.1186/s12859-022-05043-9. BMC Bioinformatics. 2022. PMID: 36471244 Free PMC article.
-
Independent Component Analysis for Unraveling the Complexity of Cancer Omics Datasets.Int J Mol Sci. 2019 Sep 7;20(18):4414. doi: 10.3390/ijms20184414. Int J Mol Sci. 2019. PMID: 31500324 Free PMC article. Review.
-
Trimming of mammalian transcriptional networks using network component analysis.BMC Bioinformatics. 2010 Oct 13;11:511. doi: 10.1186/1471-2105-11-511. BMC Bioinformatics. 2010. PMID: 20942926 Free PMC article.
References
-
- Butte A. The use and analysis of microarray data. Nat Rev Drug Discov. 2002;1:951–960. - PubMed
-
- Golub TR, Slonim DK, Tamayo P, Huard C, Gaasenbeek M, Mesirov JP, Coller H, Loh ML, Downing JR, Caligiuri MA, et al. Molecular classification of cancer: class discovery and class prediction by gene expression monitoring. Science. 1999;286:531–537. - PubMed
-
- Mukherjee S, Tamayo P, Mesirov JP, Slonim D, Verri A, Poggio T. Technical Report No 182, AI Memo 1676. MIT, Cambridge: Massachusetts Institute of Technology; 1999. Support vector machine classification of microarray data.
Publication types
MeSH terms
LinkOut - more resources
Full Text Sources
Other Literature Sources
Molecular Biology Databases
Miscellaneous