A biophysical approach to transcription factor binding site discovery
- PMID: 14597652
- PMCID: PMC403756
- DOI: 10.1101/gr.1271603
A biophysical approach to transcription factor binding site discovery
Abstract
Identification of transcription factor binding sites within regulatory segments of genomic DNA is an important step toward understanding of the regulatory circuits that control expression of genes. Here, we describe a novel bioinformatics method that bases classification of potential binding sites explicitly on the estimate of sequence-specific binding energy of a given transcription factor. The method also estimates the chemical potential of the factor that defines the threshold of binding. In contrast with the widely used information-theoretic weight matrix method, the new approach correctly describes saturation in the transcription factor/DNA binding probability. This results in a significant improvement in the number of expected false positives, particularly in the ubiquitous case of low-specificity factors. In the strong binding limit, the algorithm is related to the "support vector machine" approach to pattern recognition. The new method is used to identify likely genomic binding sites for the E. coli transcription factors collected in the DPInteract database. In addition, for CRP (a global regulatory factor), the likely regulatory modality (i.e., repressor or activator) of predicted binding sites is determined.
Figures
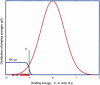
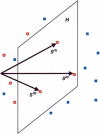
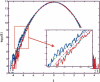
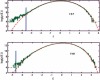
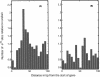
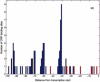
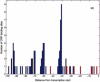
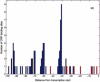
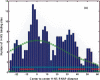
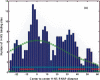
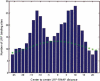
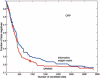
Similar articles
-
Integrating genomic data to predict transcription factor binding.Genome Inform. 2005;16(1):83-94. Genome Inform. 2005. PMID: 16362910
-
MAPPER: a search engine for the computational identification of putative transcription factor binding sites in multiple genomes.BMC Bioinformatics. 2005 Mar 30;6:79. doi: 10.1186/1471-2105-6-79. BMC Bioinformatics. 2005. PMID: 15799782 Free PMC article.
-
A motif co-occurrence approach for genome-wide prediction of transcription-factor-binding sites in Escherichia coli.Genome Res. 2004 Feb;14(2):201-8. doi: 10.1101/gr.1448004. Genome Res. 2004. PMID: 14762058 Free PMC article.
-
[Computational predictions of transcription factor binding sites].Tanpakushitsu Kakusan Koso. 2004 Dec;49(17 Suppl):2877-81. Tanpakushitsu Kakusan Koso. 2004. PMID: 15669270 Review. Japanese. No abstract available.
-
An overview of the computational analyses and discovery of transcription factor binding sites.Methods Mol Biol. 2010;674:1-22. doi: 10.1007/978-1-60761-854-6_1. Methods Mol Biol. 2010. PMID: 20827582 Review.
Cited by
-
A structure-based Multiple-Instance Learning approach to predicting in vitro transcription factor-DNA interaction.BMC Genomics. 2015;16 Suppl 4(Suppl 4):S3. doi: 10.1186/1471-2164-16-S4-S3. Epub 2015 Apr 21. BMC Genomics. 2015. PMID: 25917392 Free PMC article.
-
Improved predictions of transcription factor binding sites using physicochemical features of DNA.Nucleic Acids Res. 2012 Dec;40(22):e175. doi: 10.1093/nar/gks771. Epub 2012 Aug 25. Nucleic Acids Res. 2012. PMID: 22923524 Free PMC article.
-
Modeling DNA-binding of Escherichia coli sigma70 exhibits a characteristic energy landscape around strong promoters.Nucleic Acids Res. 2007;35(20):7003-10. doi: 10.1093/nar/gkm720. Epub 2007 Oct 16. Nucleic Acids Res. 2007. PMID: 17940097 Free PMC article.
-
Interacting models of cooperative gene regulation.Proc Natl Acad Sci U S A. 2004 Nov 16;101(46):16234-9. doi: 10.1073/pnas.0407365101. Epub 2004 Nov 8. Proc Natl Acad Sci U S A. 2004. PMID: 15534222 Free PMC article.
-
Novel computational analysis of protein binding array data identifies direct targets of Nkx2.2 in the pancreas.BMC Bioinformatics. 2011 Feb 25;12:62. doi: 10.1186/1471-2105-12-62. BMC Bioinformatics. 2011. PMID: 21352540 Free PMC article.
References
-
- Berg, O.G. and von Hippel, P.H. 1987. Selection of DNA binding sites by regulatory proteins: Statistical-mechanical theory and application to operators and promoters. J. Mol. Biol. 193: 723-750. - PubMed
-
- Blattner, F.R., Plunkett III, G., Bloch, C.A., Perna, N.T., Burland, V., Riley, M., Collado-Vides, J., Glasner, J.D., Rode, C.K., Mayhew, G.F., et al. 1997. The complete genome sequence of E. coli K-12. Science 277: 1453-1462. - PubMed
-
- Busby, S. and Ebright, R. 1999. Transcription activation by catabolite activator protein (CAP). J. Mol. Biol. 293: 199-213. - PubMed
-
- Cristianini, N. and Shawe-Taylor, J. 2001. An introduction to support vector machines. Cambridge University Press, Cambridge, UK.
WEB SITE REFERENCES
-
- http://arep.med.harvard.edu/dpinteract/; DPInteract.
-
- http://www.ecocyc.org; EcoCyc database.
Publication types
MeSH terms
Substances
Grants and funding
LinkOut - more resources
Full Text Sources
Other Literature Sources
Molecular Biology Databases
Research Materials
Miscellaneous