Distinguishing regulatory DNA from neutral sites
- PMID: 12529307
- PMCID: PMC430974
- DOI: 10.1101/gr.817703
Distinguishing regulatory DNA from neutral sites
Abstract
We explore several computational approaches to analyzing interspecies genomic sequence alignments, aiming to distinguish regulatory regions from neutrally evolving DNA. Human-mouse genomic alignments were collected for three sets of human regions: (1) experimentally defined gene regulatory regions, (2) well-characterized exons (coding sequences, as a positive control), and (3) interspersed repeats thought to have inserted before the human-mouse split (a good model for neutrally evolving DNA). Models that potentially could distinguish functional noncoding sequences from neutral DNA were evaluated on these three data sets, as well as bulk genome alignments. Our analyses show that discrimination based on frequencies of individual nucleotide pairs or gaps (i.e., of possible alignment columns) is only partially successful. In contrast, scoring procedures that include the alignment context, based on frequencies of short runs of alignment columns, dramatically improve separation between regulatory and neutral features. Such scoring functions should aid in the identification of putative regulatory regions throughout the human genome.
Figures
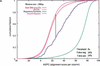
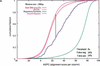
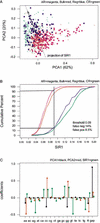
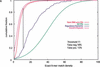
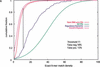
Similar articles
-
ESPERR: learning strong and weak signals in genomic sequence alignments to identify functional elements.Genome Res. 2006 Dec;16(12):1596-604. doi: 10.1101/gr.4537706. Epub 2006 Oct 19. Genome Res. 2006. PMID: 17053093 Free PMC article.
-
Regulatory potential scores from genome-wide three-way alignments of human, mouse, and rat.Genome Res. 2004 Apr;14(4):700-7. doi: 10.1101/gr.1976004. Genome Res. 2004. PMID: 15060013 Free PMC article.
-
Gene structure prediction by spliced alignment of genomic DNA with protein sequences: increased accuracy by differential splice site scoring.J Mol Biol. 2000 Apr 14;297(5):1075-85. doi: 10.1006/jmbi.2000.3641. J Mol Biol. 2000. PMID: 10764574
-
Cluster analysis and promoter modelling as bioinformatics tools for the identification of target genes from expression array data.Pharmacogenomics. 2001 Feb;2(1):25-36. doi: 10.1517/14622416.2.1.25. Pharmacogenomics. 2001. PMID: 11258194 Review.
-
Computation and analysis of genomic multi-sequence alignments.Annu Rev Genomics Hum Genet. 2007;8:193-213. doi: 10.1146/annurev.genom.8.080706.092300. Annu Rev Genomics Hum Genet. 2007. PMID: 17489682 Review.
Cited by
-
Sequence comparison of human and mouse genes reveals a homologous block structure in the promoter regions.Genome Res. 2004 Sep;14(9):1711-8. doi: 10.1101/gr.2435604. Genome Res. 2004. PMID: 15342556 Free PMC article.
-
Chromatin architecture and transcription factor binding regulate expression of erythrocyte membrane protein genes.Mol Cell Biol. 2009 Oct;29(20):5399-412. doi: 10.1128/MCB.00777-09. Epub 2009 Aug 17. Mol Cell Biol. 2009. PMID: 19687298 Free PMC article.
-
Local DNA topography correlates with functional noncoding regions of the human genome.Science. 2009 Apr 17;324(5925):389-92. doi: 10.1126/science.1169050. Epub 2009 Mar 12. Science. 2009. PMID: 19286520 Free PMC article.
-
Comprehensive association analysis of APOE regulatory region polymorphisms in Alzheimer disease.Neurogenetics. 2004 Dec;5(4):201-8. doi: 10.1007/s10048-004-0189-9. Epub 2004 Sep 29. Neurogenetics. 2004. PMID: 15455263
-
Integrating sequence, evolution and functional genomics in regulatory genomics.Genome Biol. 2009;10(1):202. doi: 10.1186/gb-2009-10-1-202. Epub 2009 Jan 30. Genome Biol. 2009. PMID: 19226437 Free PMC article. Review.
References
-
- Berman B.P., Nibu, Y., Pfeiffer, B.D., Tomancak, P., Celniker, S.E., Levine, M., Rubin, G.M., and Eisen, M.B. 2002. Exploiting transcription factor binding site clustering to identify cis-regulatory modules involved in pattern formation in the Drosophila gene. Proc. Natl. Acad. Sci. 99: 757-762. - PMC - PubMed
-
- Burge C. and Karlin, S. 1997. Prediction of complete gene structures in human genomic DNA. J. Mol. Biol. 268: 78-94. - PubMed
Publication types
MeSH terms
Substances
Grants and funding
LinkOut - more resources
Full Text Sources
Other Literature Sources