This is a preprint.
How the Structure of Signaling Regulation Evolves: Insights from an Evolutionary Model
- PMID: 39484560
- PMCID: PMC11526956
- DOI: 10.1101/2024.10.23.619883
How the Structure of Signaling Regulation Evolves: Insights from an Evolutionary Model
Abstract
To remain responsive to environmental changes, signaling pathways attenuate their activity with negative feedback loops (NFLs), where proteins produced upon stimulation downregulate the response. NFLs function both upstream of signaling to reduce input and downstream to reduce output. Unlike upstream NFLs, downstream NFLs directly regulate gene expression without the involvement of intermediate proteins. Thus, we hypothesized that downstream NFLs evolve under more stringent selection than upstream NFLs. Indeed, genes encoding downstream NFLs exhibit a slower evolutionary rate than upstream genes. Such differences in selective pressures could result in the robust evolution of downstream NFLs while making the evolution of upstream NFLs more sensitive to changes in signaling proteins and stimuli. Here, we test these assumptions within the context of immune signaling. Our minimal model of immune signaling predicts robust evolution of downstream NFLs to changes in model parameters. This is consistent with their critical role in regulating signaling and the conservative rate of evolution. Furthermore, we show that the number of signaling steps needed to activate a downstream NFL is influenced by the cost of signaling. Our model predicts that upstream NFLs are more likely to evolve under a shorter half-life of signaling proteins, absence of host-pathogen co-evolution, and a high infection rate. Although it has been proposed that NFLs evolve to reduce the cost of signaling, we show that a high cost does not necessarily predict the evolution of upstream NFLs. The insights from our model have broad implications for understanding the evolution of regulatory mechanisms across signaling pathways.
Keywords: Negative feedback; downstream regulation; multi-level regulation; upstream regulation.
Figures
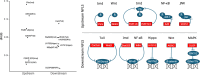
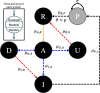
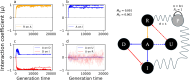
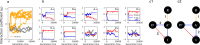
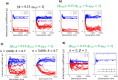
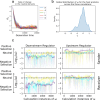
Similar articles
-
The relationship between the hierarchical position of proteins in the human signal transduction network and their rate of evolution.BMC Evol Biol. 2012 Sep 28;12:192. doi: 10.1186/1471-2148-12-192. BMC Evol Biol. 2012. PMID: 23020283 Free PMC article.
-
Attention to Physical Activity-Equivalent Calorie Information on Nutrition Facts Labels: An Eye-Tracking Investigation.J Nutr Educ Behav. 2017 Jan;49(1):35-42.e1. doi: 10.1016/j.jneb.2016.10.001. Epub 2016 Nov 16. J Nutr Educ Behav. 2017. PMID: 27865642
-
Evolutionary patterns of Toll-like receptor signaling pathway genes in the Suidae.BMC Evol Biol. 2016 Feb 9;16:33. doi: 10.1186/s12862-016-0602-7. BMC Evol Biol. 2016. PMID: 26860534 Free PMC article.
-
Regulation and Role of Store-Operated Ca2+ Entry in Cellular Proliferation.In: Kozak JA, Putney JW Jr, editors. Calcium Entry Channels in Non-Excitable Cells. Boca Raton (FL): CRC Press/Taylor & Francis; 2018. Chapter 12. In: Kozak JA, Putney JW Jr, editors. Calcium Entry Channels in Non-Excitable Cells. Boca Raton (FL): CRC Press/Taylor & Francis; 2018. Chapter 12. PMID: 30299656 Free Books & Documents. Review.
-
Store-Independent Orai Channels Regulated by STIM.In: Kozak JA, Putney JW Jr, editors. Calcium Entry Channels in Non-Excitable Cells. Boca Raton (FL): CRC Press/Taylor & Francis; 2018. Chapter 11. In: Kozak JA, Putney JW Jr, editors. Calcium Entry Channels in Non-Excitable Cells. Boca Raton (FL): CRC Press/Taylor & Francis; 2018. Chapter 11. PMID: 30299650 Free Books & Documents. Review.
References
-
- Aggarwal K., & Silverman N. (2008). Positive and negative regulation of the Drosophila immune response. BMB Reports, 41(4), 267–277. - PubMed
-
- Asgari D., & Tate A. T. (2024). Positioning of negative feedback loops within immune signaling pathways influences host fitness through noise in AMP expression. In bioRxiv (p. 2024.02.22.581613). 10.1101/2024.02.22.581613 - DOI
-
- Beg A. A., Sha W. C., Bronson R. T., & Baltimore D. (1995). Constitutive NF-kappa B activation, enhanced granulopoiesis, and neonatal lethality in I kappa B alpha-deficient mice. Genes & Development, 9(22), 2736–2746. - PubMed
Publication types
Grants and funding
LinkOut - more resources
Full Text Sources