Graph masked self-distillation learning for prediction of mutation impact on protein-protein interactions
- PMID: 39462102
- PMCID: PMC11513059
- DOI: 10.1038/s42003-024-07066-9
Graph masked self-distillation learning for prediction of mutation impact on protein-protein interactions
Abstract
Assessing mutation impact on the binding affinity change (ΔΔG) of protein-protein interactions (PPIs) plays a crucial role in unraveling structural-functional intricacies of proteins and developing innovative protein designs. In this study, we present a deep learning framework, PIANO, for improved prediction of ΔΔG in PPIs. The PIANO framework leverages a graph masked self-distillation scheme for protein structural geometric representation pre-training, which effectively captures the structural context representations surrounding mutation sites, and makes predictions using a multi-branch network consisting of multiple encoders for amino acids, atoms, and protein sequences. Extensive experiments demonstrated its superior prediction performance and the capability of pre-trained encoder in capturing meaningful representations. Compared to previous methods, PIANO can be widely applied on both holo complex structures and apo monomer structures. Moreover, we illustrated the practical applicability of PIANO in highlighting pathogenic mutations and crucial proteins, and distinguishing de novo mutations in disease cases and controls in PPI systems. Overall, PIANO offers a powerful deep learning tool, which may provide valuable insights into the study of drug design, therapeutic intervention, and protein engineering.
© 2024. The Author(s).
Conflict of interest statement
The authors declare no competing interests.
Figures
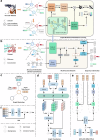
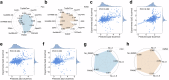
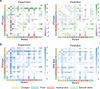
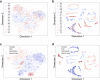
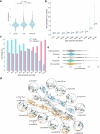
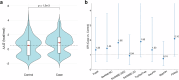
Similar articles
-
Multimodal deep representation learning for protein interaction identification and protein family classification.BMC Bioinformatics. 2019 Dec 2;20(Suppl 16):531. doi: 10.1186/s12859-019-3084-y. BMC Bioinformatics. 2019. PMID: 31787089 Free PMC article.
-
DL-PPI: a method on prediction of sequenced protein-protein interaction based on deep learning.BMC Bioinformatics. 2023 Dec 14;24(1):473. doi: 10.1186/s12859-023-05594-5. BMC Bioinformatics. 2023. PMID: 38097937 Free PMC article.
-
Prediction of mutation-induced protein stability changes based on the geometric representations learned by a self-supervised method.BMC Bioinformatics. 2024 Aug 28;25(1):282. doi: 10.1186/s12859-024-05876-6. BMC Bioinformatics. 2024. PMID: 39198740 Free PMC article.
-
Revolutionizing protein-protein interaction prediction with deep learning.Curr Opin Struct Biol. 2024 Apr;85:102775. doi: 10.1016/j.sbi.2024.102775. Epub 2024 Feb 7. Curr Opin Struct Biol. 2024. PMID: 38330793 Review.
-
Computational tools to predict context-specific protein complexes.Curr Opin Struct Biol. 2024 Oct;88:102883. doi: 10.1016/j.sbi.2024.102883. Epub 2024 Jul 9. Curr Opin Struct Biol. 2024. PMID: 38986166 Review.
References
-
- David, A., Razali, R., Wass, M. N. & Sternberg, M. J. Protein-protein interaction sites are hot spots for disease-associated nonsynonymous SNPs. Hum. Mutat.33, 359–363 (2012). - PubMed
-
- Chuderland, D. & Seger, R. Protein-protein interactions in the regulation of the extracellular signal-regulated kinase. Mol. Biotechnol.29, 57–74 (2005). - PubMed
-
- Cheng, J. et al. Accurate proteome-wide missense variant effect prediction with AlphaMissense. Science381, eadg7492 (2023). - PubMed
-
- Rabbani, G., Baig, M. H., Ahmad, K. & Choi, I. Protein-protein Interactions and their Role in Various Diseases and their Prediction Techniques. Curr. Protein Pept. Sci.19, 948–957 (2018). - PubMed
MeSH terms
Substances
Grants and funding
LinkOut - more resources
Full Text Sources