Brain tumor classification using fine-tuned transfer learning models on magnetic resonance imaging (MRI) images
- PMID: 39381813
- PMCID: PMC11459499
- DOI: 10.1177/20552076241286140
Brain tumor classification using fine-tuned transfer learning models on magnetic resonance imaging (MRI) images
Abstract
Objective: Brain tumors are a leading global cause of mortality, often leading to reduced life expectancy and challenging recovery. Early detection significantly improves survival rates. This paper introduces an efficient deep learning model to expedite brain tumor detection through timely and accurate identification using magnetic resonance imaging images.
Methods: Our approach leverages deep transfer learning with six transfer learning algorithms: VGG16, ResNet50, MobileNetV2, DenseNet201, EfficientNetB3, and InceptionV3. We optimize data preprocessing, upsample data through augmentation, and train the models using two optimizers: Adam and AdaMax. We perform three experiments with binary and multi-class datasets, fine-tuning parameters to reduce overfitting. Model effectiveness is analyzed using various performance scores with and without cross-validation.
Results: With smaller datasets, the models achieve 100% accuracy in both training and testing without cross-validation. After applying cross-validation, the framework records an outstanding accuracy of 99.96% with a receiver operating characteristic of 100% on average across five tests. For larger datasets, accuracy ranges from 96.34% to 98.20% across different models. The methodology also demonstrates a small computation time, contributing to its reliability and speed.
Conclusion: The study establishes a new standard for brain tumor classification, surpassing existing methods in accuracy and efficiency. Our deep learning approach, incorporating advanced transfer learning algorithms and optimized data processing, provides a robust and rapid solution for brain tumor detection.
Keywords: AdaMax optimizer.; Adam; Brain tumor detection; convolutional neural network; deep learning; magnetic resonance imaging; transfer learning.
© The Author(s) 2024.
Figures
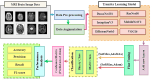
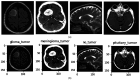
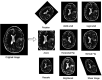
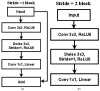
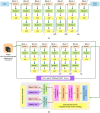
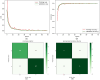
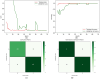
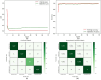
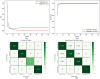
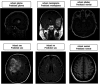
Similar articles
-
BreastNet18: A High Accuracy Fine-Tuned VGG16 Model Evaluated Using Ablation Study for Diagnosing Breast Cancer from Enhanced Mammography Images.Biology (Basel). 2021 Dec 17;10(12):1347. doi: 10.3390/biology10121347. Biology (Basel). 2021. PMID: 34943262 Free PMC article.
-
FT-FEDTL: A fine-tuned feature-extracted deep transfer learning model for multi-class microwave-based brain tumor classification.Comput Biol Med. 2024 Dec;183:109316. doi: 10.1016/j.compbiomed.2024.109316. Epub 2024 Nov 2. Comput Biol Med. 2024. PMID: 39489108
-
Enhancing parasitic organism detection in microscopy images through deep learning and fine-tuned optimizer.Sci Rep. 2024 Mar 8;14(1):5753. doi: 10.1038/s41598-024-56323-8. Sci Rep. 2024. PMID: 38459096 Free PMC article.
-
Automated Monkeypox Skin Lesion Detection Using Deep Learning and Transfer Learning Techniques.Int J Environ Res Public Health. 2023 Mar 1;20(5):4422. doi: 10.3390/ijerph20054422. Int J Environ Res Public Health. 2023. PMID: 36901430 Free PMC article. Review.
-
Development and integration of VGG and dense transfer-learning systems supported with diverse lung images for discovery of the Coronavirus identity.Inform Med Unlocked. 2022;32:101004. doi: 10.1016/j.imu.2022.101004. Epub 2022 Jul 8. Inform Med Unlocked. 2022. PMID: 35822170 Free PMC article. Review.
Cited by
-
Parkinson's disease detection from EEG signal employing autoencoder and RBFNN-based hybrid deep learning framework utilizing power spectral density.Digit Health. 2024 Nov 12;10:20552076241297355. doi: 10.1177/20552076241297355. eCollection 2024 Jan-Dec. Digit Health. 2024. PMID: 39539721 Free PMC article.
References
-
- Park KS. Nervous system. In: Humans and electricity: Understanding body electricity and applications. Korea: Springer Cham, 2023, pp.27–51.
-
- Talukder MA, Islam MM, Uddin MA, et al.. An efficient deep learning model to categorize brain tumor using reconstruction and fine-tuning. Expert Syst Appl 2023; 230: 120534.
-
- Evans-Martin F. The nervous system. New York: Infobase Holdings, Inc., 2022.
-
- Legler JM, Ries LAG, Smith MA, et al.. Brain and other central nervous system cancers: recent trends in incidence and mortality. J Nat Cancer Inst 1999; 91: 1382–1390. - PubMed
-
- Packer RJ, Gurney JG, Punyko JA, et al.. Long-term neurologic and neurosensory sequelae in adult survivors of a childhood brain tumor: childhood cancer survivor study. J Clin Oncol 2003; 21: 3255–3261. - PubMed
LinkOut - more resources
Full Text Sources