Gut Microbiome and Hepatic Transcriptomic Determinants of HCC Development in Mice with Metabolic Dysfunction-Associated Steatohepatitis
- PMID: 39372712
- PMCID: PMC11456366
- DOI: 10.2147/JHC.S485532
Gut Microbiome and Hepatic Transcriptomic Determinants of HCC Development in Mice with Metabolic Dysfunction-Associated Steatohepatitis
Abstract
Purpose: Hepatocellular carcinoma (HCC) related to metabolic dysfunction-associated steatotic liver disease (MASLD) is often diagnosed at a late stage, and its incidence is increasing. Predictive biomarkers are therefore needed to identify individuals at high risk of HCC. We aimed to characterize the gut microbiome and hepatic transcriptome associated with HCC development in female mice with hepatocyte-deletion of Pten (HepPten -). These mice present with large variations in HCC development, making them a powerful model for biomarker discovery.
Methods & results: Sequencing of stool 16S and hepatic RNA was performed on a first set of mice. Among all liver histology parameters measured, the strongest association with microbiome composition changes was with the number of tumors detected at necropsy, followed by inflammation. The gut microbiome of mice with more than 2 tumors was enriched with Lachnospiraceae UCG and depleted of Palleniella intestinalis and Odoribacter. In contrast, hepatic transcriptomic changes were most strongly associated with tumor burden, followed by liver fibrosis. The 840 differentially expressed genes correlating with tumor burden were enriched in leukocyte extravasation and interleukin 10 receptor A (IL10RA) pathways. In addition, the abundance of Spp1-high epithelial cells is correlated with tumor burden. Association between tumor number and depletion of Palleniella intestinalis, and between tumor burden and circulating levels of C-X-C motif chemokine ligand 13 (CXCL13) and stem cell factor (SCF), was further validated in an independent set of mice.
Conclusion: We identified microbiome components contributing to liver carcinogenesis by inducing inflammation, and changes in hepatic gene expression and hepatic cells distribution that contribute to tumor growth. Such information can be highly valuable for the development of new prevention strategies as well as of new biomarkers for risk modeling in HCC.
Keywords: MASLD; cancer risk biomarkers; liver cancer; microbiome.
© 2024 Dolapchiev et al.
Conflict of interest statement
The authors have declared that no conflict of interests exists in this work.
Figures
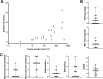
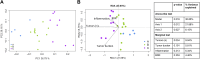
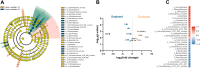
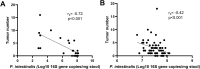
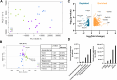
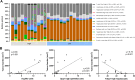
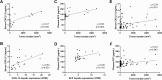
Similar articles
-
Pathology and Pathogenesis of Metabolic Dysfunction-Associated Steatotic Liver Disease-Associated Hepatic Tumors.Biomedicines. 2023 Oct 12;11(10):2761. doi: 10.3390/biomedicines11102761. Biomedicines. 2023. PMID: 37893134 Free PMC article. Review.
-
RASSF4 Attenuates Metabolic Dysfunction-Associated Steatotic Liver Disease Progression via Hippo Signaling and Suppresses Hepatocarcinogenesis.Cell Mol Gastroenterol Hepatol. 2024;18(2):101348. doi: 10.1016/j.jcmgh.2024.04.005. Epub 2024 Apr 30. Cell Mol Gastroenterol Hepatol. 2024. PMID: 38697356 Free PMC article.
-
Probiotic Mixture Ameliorates a Diet-Induced MASLD/MASH Murine Model through the Regulation of Hepatic Lipid Metabolism and the Gut Microbiome.J Agric Food Chem. 2024 Apr 17;72(15):8536-8549. doi: 10.1021/acs.jafc.3c08910. Epub 2024 Apr 4. J Agric Food Chem. 2024. PMID: 38575146
-
Histidine-rich glycoprotein in metabolic dysfunction-associated steatohepatitis-related disease progression and liver carcinogenesis.Front Immunol. 2024 Feb 26;15:1342404. doi: 10.3389/fimmu.2024.1342404. eCollection 2024. Front Immunol. 2024. PMID: 38469298 Free PMC article.
-
Association between Metabolic-Dysfunction-Associated Steatotic Liver Disease and Hepatic Cancer: Current Concepts and Future Challenges.J Clin Med. 2024 May 27;13(11):3132. doi: 10.3390/jcm13113132. J Clin Med. 2024. PMID: 38892843 Free PMC article. Review.
References
-
- Siegel RL, Miller KD, Jemal A. Cancer statistics, 2019. Ca a Cancer J Clinicians. 2019;69(1):7–34. - PubMed
Grants and funding
LinkOut - more resources
Full Text Sources
Research Materials
Miscellaneous