StableMate: a statistical method to select stable predictors in omics data
- PMID: 39345755
- PMCID: PMC11437361
- DOI: 10.1093/nargab/lqae130
StableMate: a statistical method to select stable predictors in omics data
Abstract
Identifying statistical associations between biological variables is crucial to understanding molecular mechanisms. Most association studies are based on correlation or linear regression analyses, but the identified associations often lack reproducibility and interpretability due to the complexity and variability of omics datasets, making it difficult to translate associations into meaningful biological hypotheses. We developed StableMate, a regression framework, to address these challenges through a process of variable selection across heterogeneous datasets. Given datasets from different environments, such as experimental batches, StableMate selects environment-agnostic (stable) and environment-specific predictors in predicting the response of interest. Stable predictors represent robust functional dependencies with the response, and can be used to build regression models that make generalizable predictions in unseen environments. We applied StableMate to (i) RNA sequencing data of breast cancer to discover genes that consistently predict estrogen receptor expression across disease status; (ii) metagenomics data to identify microbial signatures that show persistent association with colon cancer across study cohorts; and (iii) single-cell RNA sequencing data of glioblastoma to discern signature genes associated with the development of pro-tumour microglia regardless of cell location. Our case studies demonstrate that StableMate is adaptable to regression and classification analyses and achieves comprehensive characterization of biological systems for different omics data types.
© The Author(s) 2024. Published by Oxford University Press on behalf of NAR Genomics and Bioinformatics.
Figures
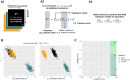
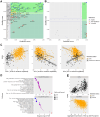
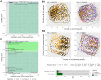
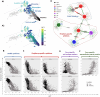
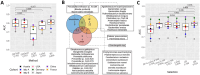
Similar articles
-
Translational Metabolomics of Head Injury: Exploring Dysfunctional Cerebral Metabolism with Ex Vivo NMR Spectroscopy-Based Metabolite Quantification.In: Kobeissy FH, editor. Brain Neurotrauma: Molecular, Neuropsychological, and Rehabilitation Aspects. Boca Raton (FL): CRC Press/Taylor & Francis; 2015. Chapter 25. In: Kobeissy FH, editor. Brain Neurotrauma: Molecular, Neuropsychological, and Rehabilitation Aspects. Boca Raton (FL): CRC Press/Taylor & Francis; 2015. Chapter 25. PMID: 26269925 Free Books & Documents. Review.
-
A radiomics approach to assess tumour-infiltrating CD8 cells and response to anti-PD-1 or anti-PD-L1 immunotherapy: an imaging biomarker, retrospective multicohort study.Lancet Oncol. 2018 Sep;19(9):1180-1191. doi: 10.1016/S1470-2045(18)30413-3. Epub 2018 Aug 14. Lancet Oncol. 2018. PMID: 30120041
-
Multi-omics facilitated variable selection in Cox-regression model for cancer prognosis prediction.Methods. 2017 Jul 15;124:100-107. doi: 10.1016/j.ymeth.2017.06.010. Epub 2017 Jun 13. Methods. 2017. PMID: 28627406
-
Comparison of five supervised feature selection algorithms leading to top features and gene signatures from multi-omics data in cancer.BMC Bioinformatics. 2022 Apr 28;23(Suppl 3):153. doi: 10.1186/s12859-022-04678-y. BMC Bioinformatics. 2022. PMID: 35484501 Free PMC article.
-
Using Predictive Models to Improve Care for Patients Hospitalized with COVID-19 [Internet].Washington (DC): Patient-Centered Outcomes Research Institute (PCORI); 2023 Jan. Washington (DC): Patient-Centered Outcomes Research Institute (PCORI); 2023 Jan. PMID: 38976624 Free Books & Documents. Review.
References
-
- Moerman T., Aibar Santos S., Bravo González-Blas C., Simm J., Moreau Y., Aerts J., Aerts S. GRNBoost2 and Arboreto: efficient and scalable inference of gene regulatory networks. Bioinformatics. 2019; 35:2159–2161. - PubMed
LinkOut - more resources
Full Text Sources