Systemic dysregulation and molecular insights into poor influenza vaccine response in the aging population
- PMID: 39331702
- PMCID: PMC11430404
- DOI: 10.1126/sciadv.adq7006
Systemic dysregulation and molecular insights into poor influenza vaccine response in the aging population
Abstract
Vaccination-induced protection against influenza is greatly diminished and increasingly heterogeneous with age. We investigated longitudinally (up to five time points) a cohort of 234 vaccinated >65-year-old vaccinees with adjuvanted vaccine FluAd across two independent seasons. System-level analyses of multiomics datasets measuring six modalities and serological data revealed that poor responders lacked time-dependent changes in response to vaccination as observed in responders, suggestive of systemic dysregulation in poor responders. Multiomics integration revealed key molecules and their likely role in vaccination response. High prevaccination plasma interleukin-15 (IL-15) concentrations negatively associated with antibody production, further supported by experimental validation in mice revealing an IL-15-driven natural killer cell axis explaining the suppressive role in vaccine-induced antibody production as observed in poor responders. We propose a subset of long-chain fatty acids as modulators of persistent inflammation in poor responders. Our findings provide a potential link between low-grade chronic inflammation and poor vaccination response and open avenues for possible pharmacological interventions to enhance vaccine responses.
Figures
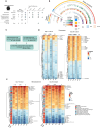
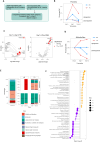
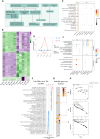

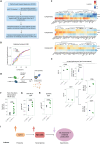
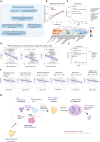
Similar articles
-
Age-Related Changes in the Natural Killer Cell Response to Seasonal Influenza Vaccination Are Not Influenced by a Synbiotic: a Randomised Controlled Trial.Front Immunol. 2018 Mar 22;9:591. doi: 10.3389/fimmu.2018.00591. eCollection 2018. Front Immunol. 2018. PMID: 29662493 Free PMC article. Clinical Trial.
-
Plasmablast antibody repertoires in elderly influenza vaccine responders exhibit restricted diversity but increased breadth of binding across influenza strains.Clin Immunol. 2018 Aug;193:70-79. doi: 10.1016/j.clim.2018.01.011. Epub 2018 Feb 2. Clin Immunol. 2018. PMID: 29410330 Free PMC article.
-
Seasonal Variability and Shared Molecular Signatures of Inactivated Influenza Vaccination in Young and Older Adults.J Immunol. 2020 Mar 15;204(6):1661-1673. doi: 10.4049/jimmunol.1900922. Epub 2020 Feb 14. J Immunol. 2020. PMID: 32060136 Free PMC article.
-
[The role of anti-influenza vaccination in elderly persons].Przegl Epidemiol. 2002;56 Suppl 1:91-9. Przegl Epidemiol. 2002. PMID: 12194236 Review. Polish.
-
Influenza vaccination in elderly people.Exp Gerontol. 1999 Jun;34(3):445-52. doi: 10.1016/s0531-5565(99)00007-8. Exp Gerontol. 1999. PMID: 10433399 Review.
References
-
- Osterholm M. T., Kelley N. S., Sommer A., Belongia E. A., Efficacy and effectiveness of influenza vaccines: A systematic review and meta-analysis. Lancet Infect. Dis. 12, 36–44 (2012). - PubMed
-
- Ferdinands J. M., Thompson M. G., Blanton L., Spencer S., Grant L., Fry A. M., Does influenza vaccination attenuate the severity of breakthrough infections? A narrative review and recommendations for further research. Vaccine 39, 3678–3695 (2021). - PubMed
-
- Jefferson T., Rivetti D., Rivetti A., Rudin M., Di Pietrantonj C., Demicheli V., Efficacy and effectiveness of influenza vaccines in elderly people: A systematic review. Lancet 366, 1165–1174 (2005). - PubMed
MeSH terms
Substances
LinkOut - more resources
Full Text Sources
Medical