mtPCDI: a machine learning-based prognostic model for prostate cancer recurrence
- PMID: 39296545
- PMCID: PMC11408181
- DOI: 10.3389/fgene.2024.1430565
mtPCDI: a machine learning-based prognostic model for prostate cancer recurrence
Abstract
Background: This research seeks to formulate a prognostic model for forecasting prostate cancer recurrence by examining the interaction between mitochondrial function and programmed cell death (PCD).
Methods: The research involved analyzing four gene expression datasets from The Cancer Genome Atlas (TCGA) and Gene Expression Omnibus (GEO) using univariate Cox regression. These analyses identified genes linked with mitochondrial function and PCD that correlate with recurrence prognosis. Various machine learning algorithms were then employed to construct an optimal predictive model.
Results: A key outcome was the creation of a mitochondrial-related programmed cell death index (mtPCDI), which effectively predicts the prognosis of prostate cancer patients. It was observed that individuals with lower mtPCDI exhibited higher immune activity, correlating with better recurrence outcomes.
Conclusion: The study demonstrates that mtPCDI can be used for personalized risk assessment and therapeutic decision-making, highlighting its clinical significance and providing insights into the biological processes affecting prostate cancer recurrence.
Keywords: machine learning; mitochondrial activity; programmed cell death; prostate cancer; targeted cancer therapy; tumor immune microenvironment.
Copyright © 2024 Cheng, Xu, Wang, Chen, Huang, Qian and Fan.
Conflict of interest statement
The authors declare that the research was conducted in the absence of any commercial or financial relationships that could be construed as a potential conflict of interest.
Figures
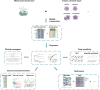
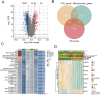
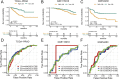
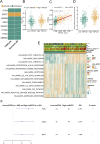
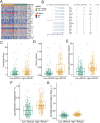
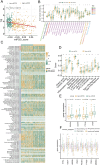
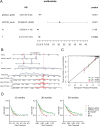
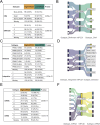
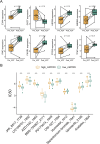
Similar articles
-
Integrated machine learning survival framework develops a prognostic model based on inter-crosstalk definition of mitochondrial function and cell death patterns in a large multicenter cohort for lower-grade glioma.J Transl Med. 2023 Sep 2;21(1):588. doi: 10.1186/s12967-023-04468-x. J Transl Med. 2023. PMID: 37660060 Free PMC article.
-
Integration of machine learning for developing a prognostic signature related to programmed cell death in colorectal cancer.Environ Toxicol. 2024 May;39(5):2908-2926. doi: 10.1002/tox.24157. Epub 2024 Feb 1. Environ Toxicol. 2024. PMID: 38299230
-
Molecular subtypes of lung adenocarcinoma patients for prognosis and therapeutic response prediction with machine learning on 13 programmed cell death patterns.J Cancer Res Clin Oncol. 2023 Oct;149(13):11351-11368. doi: 10.1007/s00432-023-05000-w. Epub 2023 Jun 28. J Cancer Res Clin Oncol. 2023. PMID: 37378675
-
Multiple programmed cell death patterns and immune landscapes in bladder cancer: Evidence based on machine learning and multi-cohorts.Environ Toxicol. 2024 Mar;39(3):1780-1801. doi: 10.1002/tox.24066. Epub 2023 Dec 8. Environ Toxicol. 2024. PMID: 38064272
-
A novel necroptosis-related gene index for predicting prognosis and a cold tumor immune microenvironment in stomach adenocarcinoma.Front Immunol. 2022 Oct 27;13:968165. doi: 10.3389/fimmu.2022.968165. eCollection 2022. Front Immunol. 2022. PMID: 36389725 Free PMC article. Review.
References
Grants and funding
LinkOut - more resources
Full Text Sources