Preventing illegal seafood trade using machine-learning assisted microbiome analysis
- PMID: 39256748
- PMCID: PMC11389296
- DOI: 10.1186/s12915-024-02005-w
Preventing illegal seafood trade using machine-learning assisted microbiome analysis
Abstract
Background: Seafood is increasingly traded worldwide, but its supply chain is particularly prone to frauds. To increase consumer confidence, prevent illegal trade, and provide independent validation for eco-labelling, accurate tools for seafood traceability are needed. Here we show that the use of microbiome profiling (MP) coupled with machine learning (ML) allows precise tracing the origin of Manila clams harvested in areas separated by small geographic distances. The study was designed to represent a real-world scenario. Clams were collected in different seasons across the most important production area in Europe (lagoons along the northern Adriatic coast) to cover the known seasonal variation in microbiome composition for the species. DNA extracted from samples underwent the same depuration process as commercial products (i.e. at least 12 h in open flow systems).
Results: Machine learning-based analysis of microbiome profiles was carried out using two completely independent sets of data (collected at the same locations but in different years), one for training the algorithm, and the other for testing its accuracy and assessing the temporal stability signal. Briefly, gills (GI) and digestive gland (DG) of clams were collected in summer and winter over two different years (i.e. from 2018 to 2020) in one banned area and four farming sites. 16S DNA metabarcoding was performed on clam tissues and the obtained amplicon sequence variants (ASVs) table was used as input for ML MP. The best-predicting performances were obtained using the combined information of GI and DG (consensus analysis), showing a Cohen K-score > 0.95 when the target was the classification of samples collected from the banned area and those harvested at farming sites. Classification of the four different farming areas showed slightly lower accuracy with a 0.76 score.
Conclusions: We show here that MP coupled with ML is an effective tool to trace the origin of shellfish products. The tool is extremely robust against seasonal and inter-annual variability, as well as product depuration, and is ready for implementation in routine assessment to prevent the trade of illegally harvested or mislabeled shellfish.
Keywords: Food traceability; Illegal unreported unregulated (IUU) fishing; Machine learning; Manila clam; Microbiota 16S; North Adriatic sea.
© 2024. The Author(s).
Conflict of interest statement
The authors declare that they have no competing interests.
Figures
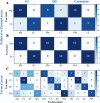
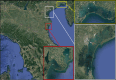
Similar articles
-
Tracing seafood at high spatial resolution using NGS-generated data and machine learning: Comparing microbiome versus SNPs.Food Chem. 2019 Jul 15;286:413-420. doi: 10.1016/j.foodchem.2019.02.037. Epub 2019 Feb 15. Food Chem. 2019. PMID: 30827626
-
Isotope Geochemistry for Seafood Traceability and Authentication: The Northern Adriatic Manila Clams Case Study.Foods. 2022 Oct 1;11(19):3054. doi: 10.3390/foods11193054. Foods. 2022. PMID: 36230130 Free PMC article.
-
Erratum: Eyestalk Ablation to Increase Ovarian Maturation in Mud Crabs.J Vis Exp. 2023 May 26;(195). doi: 10.3791/6561. J Vis Exp. 2023. PMID: 37235796
-
Shellfish and residual chemical contaminants: hazards, monitoring, and health risk assessment along French coasts.Rev Environ Contam Toxicol. 2011;213:55-111. doi: 10.1007/978-1-4419-9860-6_3. Rev Environ Contam Toxicol. 2011. PMID: 21541848 Review.
-
How to Determine the Geographical Origin of Seafood?Crit Rev Food Sci Nutr. 2016;56(2):306-17. doi: 10.1080/10408398.2012.745478. Crit Rev Food Sci Nutr. 2016. PMID: 24834835 Review.
References
-
- Mamede R, Ricardo F, Santos A, Díaz S, Santos SAO, Bispo R, et al. Revealing the illegal harvesting of Manila clams (Ruditapes philippinarum) using fatty acid profiles of the adductor muscle. Food Control. 2020;118:107368.10.1016/j.foodcont.2020.107368 - DOI
-
- Martini A, Aguiari L, Capoccioni F, Martinoli M, Napolitano R, Pirlo G, et al. Is Manila clam farming environmentally sustainable? A Life Cycle Assessment (LCA) approach applied to an Italian Ruditapes philippinarum hatchery. Sustainability. 2023;15(4):3237.10.3390/su15043237 - DOI
-
- Humphreys J, Harris MRC, Herbert RJH, Farrell P, Jensen A, Cragg SM. Introduction, dispersal and naturalization of the Manila clam Ruditapes philippinarum in British estuaries, 1980–2010. J Mar Biol Assoc UK. 2015;95:1163–72.10.1017/S0025315415000132 - DOI
-
- Turolla E, Castaldelli G, Fano EA, Tamburini E. Life cycle assessment (LCA) proves that Manila clam farming (Ruditapes philippinarum) is a fully sustainable aquaculture practice and a carbon sink. Sustainability. 2020;12(13):5252.10.3390/su12135252 - DOI
MeSH terms
LinkOut - more resources
Full Text Sources