Machine learning approaches identify immunologic signatures of total and intact HIV DNA during long-term antiretroviral therapy
- PMID: 39250423
- PMCID: PMC11383529
- DOI: 10.7554/eLife.94899
Machine learning approaches identify immunologic signatures of total and intact HIV DNA during long-term antiretroviral therapy
Abstract
Understanding the interplay between the HIV reservoir and the host immune system may yield insights into HIV persistence during antiretroviral therapy (ART) and inform strategies for a cure. Here, we applied machine learning (ML) approaches to cross-sectional high-parameter HIV reservoir and immunology data in order to characterize host-reservoir associations and generate new hypotheses about HIV reservoir biology. High-dimensional immunophenotyping, quantification of HIV-specific T cell responses, and measurement of genetically intact and total HIV proviral DNA frequencies were performed on peripheral blood samples from 115 people with HIV (PWH) on long-term ART. Analysis demonstrated that both intact and total proviral DNA frequencies were positively correlated with T cell activation and exhaustion. Years of ART and select bifunctional HIV-specific CD4 T cell responses were negatively correlated with the percentage of intact proviruses. A leave-one-covariate-out inference approach identified specific HIV reservoir and clinical-demographic parameters, such as age and biological sex, that were particularly important in predicting immunophenotypes. Overall, immune parameters were more strongly associated with total HIV proviral frequencies than intact proviral frequencies. Uniquely, however, expression of the IL-7 receptor alpha chain (CD127) on CD4 T cells was more strongly correlated with the intact reservoir. Unsupervised dimension reduction analysis identified two main clusters of PWH with distinct immune and reservoir characteristics. Using reservoir correlates identified in these initial analyses, decision tree methods were employed to visualize relationships among multiple immune and clinical-demographic parameters and the HIV reservoir. Finally, using random splits of our data as training-test sets, ML algorithms predicted with approximately 70% accuracy whether a given participant had qualitatively high or low levels of total or intact HIV DNA . The techniques described here may be useful for assessing global patterns within the increasingly high-dimensional data used in HIV reservoir and other studies of complex biology.
Keywords: HIV; human; immune cells; infectious disease; machine learning; microbiology.
© 2024, Semenova et al.
Conflict of interest statement
LS, YW, SF, NA, AC, DM, NG, DM, CR, EB No competing interests declared
Figures
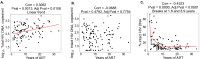
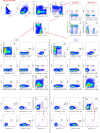
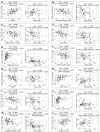
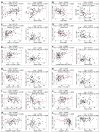
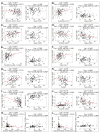
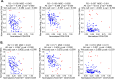
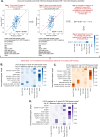
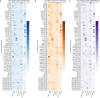
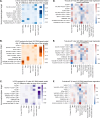
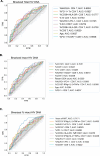
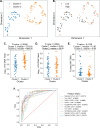
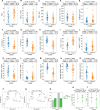
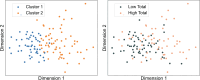
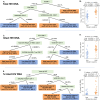
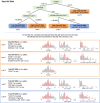
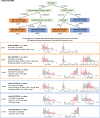
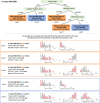
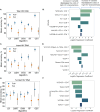
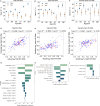
Update of
-
Machine learning approaches identify immunologic signatures of total and intact HIV DNA during long-term antiretroviral therapy.bioRxiv [Preprint]. 2024 Jun 25:2023.11.16.567386. doi: 10.1101/2023.11.16.567386. bioRxiv. 2024. Update in: Elife. 2024 Sep 09;13:RP94899. doi: 10.7554/eLife.94899 PMID: 38014340 Free PMC article. Updated. Preprint.
Similar articles
-
Machine learning approaches identify immunologic signatures of total and intact HIV DNA during long-term antiretroviral therapy.bioRxiv [Preprint]. 2024 Jun 25:2023.11.16.567386. doi: 10.1101/2023.11.16.567386. bioRxiv. 2024. Update in: Elife. 2024 Sep 09;13:RP94899. doi: 10.7554/eLife.94899 PMID: 38014340 Free PMC article. Updated. Preprint.
-
Genetic Diversity, Compartmentalization, and Age of HIV Proviruses Persisting in CD4+ T Cell Subsets during Long-Term Combination Antiretroviral Therapy.J Virol. 2020 Feb 14;94(5):e01786-19. doi: 10.1128/JVI.01786-19. Print 2020 Feb 14. J Virol. 2020. PMID: 31776273 Free PMC article.
-
Sequence Evaluation and Comparative Analysis of Novel Assays for Intact Proviral HIV-1 DNA.J Virol. 2021 Feb 24;95(6):e01986-20. doi: 10.1128/JVI.01986-20. Print 2021 Feb 24. J Virol. 2021. PMID: 33361426 Free PMC article.
-
New Approaches to Multi-Parametric HIV-1 Genetics Using Multiple Displacement Amplification: Determining the What, How, and Where of the HIV-1 Reservoir.Viruses. 2021 Dec 10;13(12):2475. doi: 10.3390/v13122475. Viruses. 2021. PMID: 34960744 Free PMC article. Review.
-
HIV-1 Reservoir Persistence and Decay: Implications for Cure Strategies.Curr HIV/AIDS Rep. 2022 Jun;19(3):194-206. doi: 10.1007/s11904-022-00604-2. Epub 2022 Apr 11. Curr HIV/AIDS Rep. 2022. PMID: 35404007 Free PMC article. Review.
References
-
- Astorga-Gamaza A, Perea D, Sanchez-Gaona N, Calvet-Mirabent M, Gallego-Cortés A, Grau-Expósito J, Sanchez-Cerrillo I, Rey J, Castellví J, Curran A, Burgos J, Navarro J, Suanzes P, Falcó V, Genescà M, Martín-Gayo E, Buzon MJ. KLRG1 expression on natural killer cells is associated with HIV persistence, and its targeting promotes the reduction of the viral reservoir. Cell Reports. Medicine. 2023;4:101202. doi: 10.1016/j.xcrm.2023.101202. - DOI - PMC - PubMed
-
- Banga R, Procopio FA, Noto A, Pollakis G, Cavassini M, Ohmiti K, Corpataux J-M, de Leval L, Pantaleo G, Perreau M. PD-1(+) and follicular helper T cells are responsible for persistent HIV-1 transcription in treated aviremic individuals. Nature Medicine. 2016;22:754–761. doi: 10.1038/nm.4113. - DOI - PubMed
-
- Bastard J-P, Soulié C, Fellahi S, Haïm-Boukobza S, Simon A, Katlama C, Calvez V, Marcelin A-G, Capeau J. Circulating interleukin-6 levels correlate with residual hiv viraemia and markers of immune dysfunction in treatment-controlled HIV-infected patients. Antiviral Therapy. 2012;17:915–919. doi: 10.3851/IMP2093. - DOI - PubMed
-
- Bernal S, Puertas MC, Morón-López S, Cranston RD, Urrea V, Dalmau J, Salgado M, Gálvez C, Erkizia I, McGowan I, Scherrer D, Revollo B, Sirera G, Santos JR, Clotet B, Paredes R, Martinez-Picado J. Impact of obefazimod on viral persistence, inflammation, and immune activation in people with human immunodeficiency virus on suppressive antiretroviral therapy. The Journal of Infectious Diseases. 2023;228:1280–1291. doi: 10.1093/infdis/jiad251. - DOI - PMC - PubMed
MeSH terms
Substances
Grants and funding
LinkOut - more resources
Full Text Sources
Medical
Research Materials