Use of extracellular vesicle microRNA profiles in patients with acute myeloid leukemia for the identification of novel biomarkers
- PMID: 39178208
- PMCID: PMC11343415
- DOI: 10.1371/journal.pone.0306962
Use of extracellular vesicle microRNA profiles in patients with acute myeloid leukemia for the identification of novel biomarkers
Abstract
Objectives: This study aimed to establish clinically significant microRNA (miRNA) sets using extracellular vesicles (EVs) from bone marrow (BM) aspirates of patients with acute myelogenous leukemia (AML), and to identify the genes that interact with these EV-derived miRNAs in AML.
Materials and methods: BM aspirates were collected from 32 patients with AML at the time of AML diagnosis. EVs were isolated using size-exclusion chromatography. A total of 965 EV-derived miRNAs were identified in all the samples.
Results: We analyzed the expression levels of these EV-derived miRNAs of the favorable (n = 10) and non-favorable (n = 22) risk groups; we identified 32 differentially expressed EV-derived miRNAs in the non-favorable risk group. The correlation of these miRNAs with risk stratification and patient survival was analyzed using the information of patients with AML from The Cancer Genome Atlas (TCGA) database. Of the miRNAs with downregulated expression in the non-favorable risk group, hsa-miR-181b and hsa-miR-143 were correlated with non-favorable risk and short overall survival. Regarding the miRNAs with upregulated expression in the non-favorable risk group, hsa-miR-188 and hsa-miR-501 were correlated with non-favorable risk and could predict poor survival. Through EV-derived miRNAs-mRNA network analysis using TCGA database, we identified 21 mRNAs that could be potential poor prognosis biomarkers.
Conclusions: Overall, our findings revealed that EV-derived miRNAs can serve as biomarkers for risk stratification and prognosis in AML. In addition, these EV-derived miRNA-based bioinformatic analyses could help efficiently identify mRNAs with biomarker potential, similar to the previous cell-based approach.
Copyright: © 2024 Kang et al. This is an open access article distributed under the terms of the Creative Commons Attribution License, which permits unrestricted use, distribution, and reproduction in any medium, provided the original author and source are credited.
Conflict of interest statement
The authors have declared that no competing interests exist.
Figures
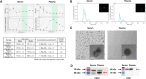
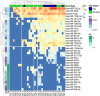
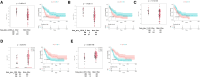
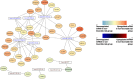
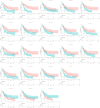
Similar articles
-
DICER1 gene and miRNA dysregulation in mesenchymal stem cells of patients with myelodysplastic syndrome and acute myeloblastic leukemia.Leuk Res. 2017 Dec;63:62-71. doi: 10.1016/j.leukres.2017.10.006. Epub 2017 Oct 31. Leuk Res. 2017. PMID: 29102598
-
High serum extracellular vesicle miR-10b expression predicts poor prognosis in patients with acute myeloid leukemia.Cancer Biomark. 2020;27(1):1-9. doi: 10.3233/CBM-190211. Cancer Biomark. 2020. PMID: 31594209
-
Differentially expressed miRNA profiles of serum derived extracellular vesicles from patients with acute ischemic stroke.Brain Res. 2024 Dec 15;1845:149171. doi: 10.1016/j.brainres.2024.149171. Epub 2024 Aug 19. Brain Res. 2024. PMID: 39168264
-
MicroRNAs as New Biomarkers for Diagnosis and Prognosis, and as Potential Therapeutic Targets in Acute Myeloid Leukemia.Int J Mol Sci. 2018 Feb 3;19(2):460. doi: 10.3390/ijms19020460. Int J Mol Sci. 2018. PMID: 29401684 Free PMC article. Review.
-
Extracellular Vesicle miRNAs in Diagnostics of Gastric Cancer.Biochemistry (Mosc). 2024 Jul;89(7):1211-1238. doi: 10.1134/S0006297924070058. Biochemistry (Mosc). 2024. PMID: 39218020 Review.
References
MeSH terms
Substances
Grants and funding
LinkOut - more resources
Full Text Sources
Medical