Machine learning developed a macrophage signature for predicting prognosis, immune infiltration and immunotherapy features in head and neck squamous cell carcinoma
- PMID: 39174693
- PMCID: PMC11341843
- DOI: 10.1038/s41598-024-70430-6
Machine learning developed a macrophage signature for predicting prognosis, immune infiltration and immunotherapy features in head and neck squamous cell carcinoma
Abstract
Macrophages played an important role in the progression and treatment of head and neck squamous cell carcinoma (HNSCC). We employed weighted gene co-expression network analysis (WGCNA) to identify macrophage-related genes (MRGs) and classify patients with HNSCC into two distinct subtypes. A macrophage-related risk signature (MRS) model, comprising nine genes: IGF2BP2, PPP1R14C, SLC7A5, KRT9, RAC2, NTN4, CTLA4, APOC1, and CYP27A1, was formulated by integrating 101 machine learning algorithm combinations. We observed lower overall survival (OS) in the high-risk group and the high-risk group showed elevated expression levels in most of the immune checkpoint and human leukocyte antigen (HLA) genes, suggesting a strong immune evasion capacity. Correspondingly, TIDE score positively correlated with risk score, implying that high-risk tumors may resist immunotherapy more effectively. At the single-cell level, we noted macrophages in the tumor microenvironment (TME) predominantly stalled in the G2/M phase, potentially hindering epithelial-mesenchymal transition and playing a crucial role in the inhibition of tumor progression. Finally, the proliferation and migration abilities of HNSCC cells significantly decreased after the expression of IGF2BP2 and SLC7A5 reduced. It also decreased migration ability of macrophages and facilitated their polarization towards the M1 direction. Our study constructed a novel MRS for HNSCC, which could serve as an indicator for predicting the prognosis, immune infiltration and immunotherapy for HNSCC patients.
Keywords: HNSCC; Immunotherapy; Macrophage; Prognostic model; Risk score.
© 2024. The Author(s).
Conflict of interest statement
The authors declare no competing interests.
Figures
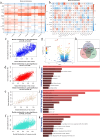
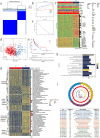
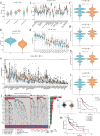
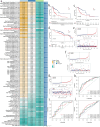
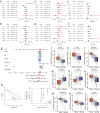
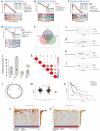
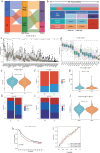
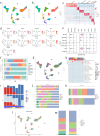
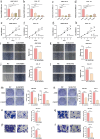
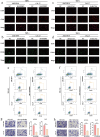
Similar articles
-
Characterization of macrophages in head and neck squamous cell carcinoma and development of MRG-based risk signature.Sci Rep. 2024 Apr 30;14(1):9914. doi: 10.1038/s41598-024-60516-6. Sci Rep. 2024. PMID: 38688945 Free PMC article.
-
A meta-validated immune infiltration-related gene model predicts prognosis and immunotherapy sensitivity in HNSCC.BMC Cancer. 2023 Jan 13;23(1):45. doi: 10.1186/s12885-023-10532-y. BMC Cancer. 2023. PMID: 36639648 Free PMC article.
-
Development of Chromatin Regulator-related Molecular Subtypes and a Signature to Predict Prognosis and Immunotherapeutic Response in Head and Neck Squamous Cell Carcinoma.Curr Cancer Drug Targets. 2024;24(8):804-819. doi: 10.2174/0115680096274798231121053634. Curr Cancer Drug Targets. 2024. PMID: 38310463 Free PMC article.
-
Comprehensive review regarding the association of E2Fs with the prognosis and immune infiltrates in human head and neck squamous cell carcinoma.Asian J Surg. 2024 May;47(5):2106-2121. doi: 10.1016/j.asjsur.2024.01.130. Epub 2024 Feb 5. Asian J Surg. 2024. PMID: 38320907 Review.
-
Macrophages: plastic participants in the diagnosis and treatment of head and neck squamous cell carcinoma.Front Immunol. 2024 Apr 8;15:1337129. doi: 10.3389/fimmu.2024.1337129. eCollection 2024. Front Immunol. 2024. PMID: 38650924 Free PMC article. Review.
Cited by
-
Artificial Intelligence in Head and Neck Cancer Diagnosis: A Comprehensive Review with Emphasis on Radiomics, Histopathological, and Molecular Applications.Cancers (Basel). 2024 Oct 27;16(21):3623. doi: 10.3390/cancers16213623. Cancers (Basel). 2024. PMID: 39518063 Free PMC article. Review.
References
-
- Global Burden of Disease Cancer Collaboration, et al. Global, Regional, and National Cancer Incidence, Mortality, Years of Life Lost, Years Lived With Disability, and Disability-Adjusted Life-years for 32 Cancer Groups, 1990 to 2015: A Systematic Analysis for the Global Burden of Disease Study. JAMA Oncol. 2017; 3(4):524–548. 10.1001/jamaoncol.2016.5688 - PMC - PubMed
MeSH terms
Substances
Grants and funding
LinkOut - more resources
Full Text Sources
Medical
Research Materials
Miscellaneous