An end-to-end deep learning method for mass spectrometry data analysis to reveal disease-specific metabolic profiles
- PMID: 39164279
- PMCID: PMC11335749
- DOI: 10.1038/s41467-024-51433-3
An end-to-end deep learning method for mass spectrometry data analysis to reveal disease-specific metabolic profiles
Abstract
Untargeted metabolomic analysis using mass spectrometry provides comprehensive metabolic profiling, but its medical application faces challenges of complex data processing, high inter-batch variability, and unidentified metabolites. Here, we present DeepMSProfiler, an explainable deep-learning-based method, enabling end-to-end analysis on raw metabolic signals with output of high accuracy and reliability. Using cross-hospital 859 human serum samples from lung adenocarcinoma, benign lung nodules, and healthy individuals, DeepMSProfiler successfully differentiates the metabolomic profiles of different groups (AUC 0.99) and detects early-stage lung adenocarcinoma (accuracy 0.961). Model flow and ablation experiments demonstrate that DeepMSProfiler overcomes inter-hospital variability and effects of unknown metabolites signals. Our ensemble strategy removes background-category phenomena in multi-classification deep-learning models, and the novel interpretability enables direct access to disease-related metabolite-protein networks. Further applying to lipid metabolomic data unveils correlations of important metabolites and proteins. Overall, DeepMSProfiler offers a straightforward and reliable method for disease diagnosis and mechanism discovery, enhancing its broad applicability.
© 2024. The Author(s).
Conflict of interest statement
All authors declare the following competing interests. All authors have filed patents for both the technology and the use of the technology to analyse metabolomic data.
Figures
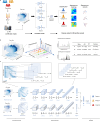
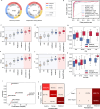
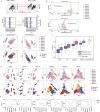
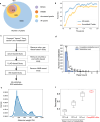
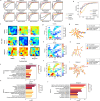
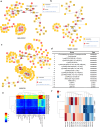
Similar articles
-
Metabolomic differentiation of benign vs malignant pulmonary nodules with high specificity via high-resolution mass spectrometry analysis of patient sera.Nat Commun. 2023 Apr 24;14(1):2339. doi: 10.1038/s41467-023-37875-1. Nat Commun. 2023. PMID: 37095081 Free PMC article.
-
Translational Metabolomics of Head Injury: Exploring Dysfunctional Cerebral Metabolism with Ex Vivo NMR Spectroscopy-Based Metabolite Quantification.In: Kobeissy FH, editor. Brain Neurotrauma: Molecular, Neuropsychological, and Rehabilitation Aspects. Boca Raton (FL): CRC Press/Taylor & Francis; 2015. Chapter 25. In: Kobeissy FH, editor. Brain Neurotrauma: Molecular, Neuropsychological, and Rehabilitation Aspects. Boca Raton (FL): CRC Press/Taylor & Francis; 2015. Chapter 25. PMID: 26269925 Free Books & Documents. Review.
-
Exploratory investigation of plasma metabolomics in human lung adenocarcinoma.Mol Biosyst. 2013 Sep;9(9):2370-8. doi: 10.1039/c3mb70138g. Mol Biosyst. 2013. PMID: 23857124
-
Serum metabolic profiling study of lung cancer using ultra high performance liquid chromatography/quadrupole time-of-flight mass spectrometry.J Chromatogr B Analyt Technol Biomed Life Sci. 2014 Sep 1;966:147-53. doi: 10.1016/j.jchromb.2014.04.047. Epub 2014 May 2. J Chromatogr B Analyt Technol Biomed Life Sci. 2014. PMID: 24856296
-
Direct infusion mass spectrometry for metabolomic phenotyping of diseases.Bioanalysis. 2017 Jan;9(1):131-148. doi: 10.4155/bio-2016-0202. Bioanalysis. 2017. PMID: 27921460 Review.
References
MeSH terms
LinkOut - more resources
Full Text Sources
Medical