This is a preprint.
Predicting the translation efficiency of messenger RNA in mammalian cells
- PMID: 39149337
- PMCID: PMC11326250
- DOI: 10.1101/2024.08.11.607362
Predicting the translation efficiency of messenger RNA in mammalian cells
Abstract
The degree to which translational control is specified by mRNA sequence is poorly understood in mammalian cells. Here, we constructed and leveraged a compendium of 3,819 ribosomal profiling datasets, distilling them into a transcriptome-wide atlas of translation efficiency (TE) measurements encompassing >140 human and mouse cell types. We subsequently developed RiboNN, a multitask deep convolutional neural network, and classic machine learning models to predict TEs in hundreds of cell types from sequence-encoded mRNA features, achieving state-of-the-art performance (r=0.79 in human and r=0.78 in mouse for mean TE across cell types). While the majority of earlier models solely considered 5' UTR sequence, RiboNN integrates contributions from the full-length mRNA sequence, learning that the 5' UTR, CDS, and 3' UTR respectively possess ~67%, 31%, and 2% per-nucleotide information density in the specification of mammalian TEs. Interpretation of RiboNN revealed that the spatial positioning of low-level di- and tri-nucleotide features (i.e., including codons) largely explain model performance, capturing mechanistic principles such as how ribosomal processivity and tRNA abundance control translational output. RiboNN is predictive of the translational behavior of base-modified therapeutic RNA, and can explain evolutionary selection pressures in human 5' UTRs. Finally, it detects a common language governing mRNA regulatory control and highlights the interconnectedness of mRNA translation, stability, and localization in mammalian organisms.
Keywords: Deep learning; Machine learning; Ribosome profiling; Translation efficiency; Translational regulation.
Conflict of interest statement
DECLARATION OF INTERESTS D.Z., J.W., F.M., and V.A. are employees of Sanofi and may hold shares and/or stock options in the company.
Figures
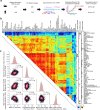
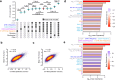
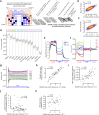
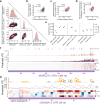
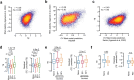
Similar articles
-
Dynamic Field Theory of Executive Function: Identifying Early Neurocognitive Markers.Monogr Soc Res Child Dev. 2024 Dec;89(3):7-109. doi: 10.1111/mono.12478. Monogr Soc Res Child Dev. 2024. PMID: 39628288 Free PMC article.
-
Enabling Systemic Identification and Functionality Profiling for Cdc42 Homeostatic Modulators.bioRxiv [Preprint]. 2024 Jan 8:2024.01.05.574351. doi: 10.1101/2024.01.05.574351. bioRxiv. 2024. Update in: Commun Chem. 2024 Nov 19;7(1):271. doi: 10.1038/s42004-024-01352-7 PMID: 38260445 Free PMC article. Updated. Preprint.
-
Depressing time: Waiting, melancholia, and the psychoanalytic practice of care.In: Kirtsoglou E, Simpson B, editors. The Time of Anthropology: Studies of Contemporary Chronopolitics. Abingdon: Routledge; 2020. Chapter 5. In: Kirtsoglou E, Simpson B, editors. The Time of Anthropology: Studies of Contemporary Chronopolitics. Abingdon: Routledge; 2020. Chapter 5. PMID: 36137063 Free Books & Documents. Review.
-
Gene and genon concept: coding versus regulation. A conceptual and information-theoretic analysis of genetic storage and expression in the light of modern molecular biology.Theory Biosci. 2007 Oct;126(2-3):65-113. doi: 10.1007/s12064-007-0012-x. Epub 2007 Sep 22. Theory Biosci. 2007. PMID: 18087760 Free PMC article.
-
Impact of residual disease as a prognostic factor for survival in women with advanced epithelial ovarian cancer after primary surgery.Cochrane Database Syst Rev. 2022 Sep 26;9(9):CD015048. doi: 10.1002/14651858.CD015048.pub2. Cochrane Database Syst Rev. 2022. PMID: 36161421 Free PMC article. Review.
References
-
- Agarwal V. & Shendure J. Predicting mRNA Abundance Directly from Genomic Sequence Using Deep Convolutional Neural Networks. Cell Rep. 31, 107663 (2020). - PubMed
Publication types
Grants and funding
LinkOut - more resources
Full Text Sources