Using XGBoost for Predicting In-Stent Restenosis Post-DES Implantation: Role of Lymphocyte-to-Monocyte Ratio and Residual Cholesterol
- PMID: 39139709
- PMCID: PMC11321347
- DOI: 10.2147/IJGM.S477053
Using XGBoost for Predicting In-Stent Restenosis Post-DES Implantation: Role of Lymphocyte-to-Monocyte Ratio and Residual Cholesterol
Abstract
Objective: This study aims to investigate their correlation and predictive utility for in-stent restenosis (ISR) in patients with acute coronary syndrome (ACS) following percutaneous coronary intervention (PCI).
Methods: We collected medical records of 668 patients who underwent PCI treatment from January 2022 to December 2022. Based on follow-up results (ISR defined as luminal narrowing ≥ 50% on angiography), all participants were divided into ISR and non-ISR groups. The XGBoost machine learning (ML) model was employed to identify the optimal predictive variables from a set of 31 variables. Discriminatory ability was evaluated using the area under the receiver operating characteristic (ROC) curve (AUC), while calibration and performance of the prediction models were assessed using the Hosmer-Lemeshow (HL) test and calibration plots. Clinical utility of each model was evaluated using decision curve analysis (DCA).
Results: In the XGBoost importance ranking of predictive factors, LMR and RC ranked first and fourth, respectively. The AUC of the entire XGBoost ML model was 0.8098, whereas the model using traditional stepwise backward regression, comprising five predictive factors, had an AUC of 0.706. The XGBoost model showed superior predictive performance with a higher AUC, indicating better discrimination and predictive accuracy for ISR compared to traditional methods.
Conclusion: LMR and RC are identified as cost-effective and reliable biomarkers for predicting ISR risk in ACS patients following drug-eluting stent (DES) implantation. LMR and RC represent cost-effective and reliable biomarkers for predicting ISR risk in ACS patients following drug-eluting stent implantation. Enhances the accuracy and clinical utility of ISR prediction models, offering clinicians a robust tool for risk stratification and personalized patient management.
Keywords: Lymphocyte-to-monocyte ratio; XGBoost; drug-eluting stent; in-stent restenosis; machine learning; residual cholesterol.
© 2024 Hou et al.
Conflict of interest statement
The authors have no conflicts of interest to declare in this work.
Figures
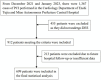
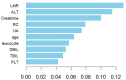
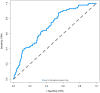
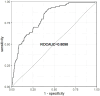
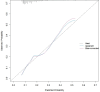
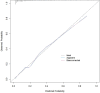
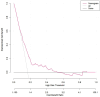
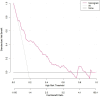
Similar articles
-
A Nomogram for Predicting In-Stent Restenosis Risk in Patients Undergoing Percutaneous Coronary Intervention: A Population-Based Analysis.Int J Gen Med. 2022 Mar 3;15:2451-2461. doi: 10.2147/IJGM.S357250. eCollection 2022. Int J Gen Med. 2022. PMID: 35264881 Free PMC article.
-
Risk investigation of in-stent restenosis after initial implantation of intracoronary drug-eluting stent in patients with coronary heart disease.Front Cardiovasc Med. 2023 Mar 10;10:1117915. doi: 10.3389/fcvm.2023.1117915. eCollection 2023. Front Cardiovasc Med. 2023. PMID: 36970340 Free PMC article.
-
Risk factors for revascularization and in-stent restenosis in patients with triple-vessel disease after second-generation drug-eluting stent implantation: a retrospective analysis.BMC Cardiovasc Disord. 2021 Sep 17;21(1):446. doi: 10.1186/s12872-021-02259-0. BMC Cardiovasc Disord. 2021. PMID: 34535088 Free PMC article.
-
Development and validation of a risk prediction nomogram for in-stent restenosis in patients undergoing percutaneous coronary intervention.BMC Cardiovasc Disord. 2021 Sep 14;21(1):435. doi: 10.1186/s12872-021-02255-4. BMC Cardiovasc Disord. 2021. PMID: 34521385 Free PMC article.
-
Triglyceride-glucose index is associated with in-stent restenosis in patients with acute coronary syndrome after percutaneous coronary intervention with drug-eluting stents.Cardiovasc Diabetol. 2021 Jul 8;20(1):137. doi: 10.1186/s12933-021-01332-4. Cardiovasc Diabetol. 2021. PMID: 34238294 Free PMC article.
Cited by
-
Analyzing dissemination, quality, and reliability of Chinese brain tumor-related short videos on TikTok and Bilibili: a cross-sectional study.Front Neurol. 2024 Oct 18;15:1404038. doi: 10.3389/fneur.2024.1404038. eCollection 2024. Front Neurol. 2024. PMID: 39494168 Free PMC article.
References
Grants and funding
LinkOut - more resources
Full Text Sources
Miscellaneous