De novo generation of SARS-CoV-2 antibody CDRH3 with a pre-trained generative large language model
- PMID: 39127753
- PMCID: PMC11316817
- DOI: 10.1038/s41467-024-50903-y
De novo generation of SARS-CoV-2 antibody CDRH3 with a pre-trained generative large language model
Abstract
Artificial Intelligence (AI) techniques have made great advances in assisting antibody design. However, antibody design still heavily relies on isolating antigen-specific antibodies from serum, which is a resource-intensive and time-consuming process. To address this issue, we propose a Pre-trained Antibody generative large Language Model (PALM-H3) for the de novo generation of artificial antibodies heavy chain complementarity-determining region 3 (CDRH3) with desired antigen-binding specificity, reducing the reliance on natural antibodies. We also build a high-precision model antigen-antibody binder (A2binder) that pairs antigen epitope sequences with antibody sequences to predict binding specificity and affinity. PALM-H3-generated antibodies exhibit binding ability to SARS-CoV-2 antigens, including the emerging XBB variant, as confirmed through in-silico analysis and in-vitro assays. The in-vitro assays validate that PALM-H3-generated antibodies achieve high binding affinity and potent neutralization capability against spike proteins of SARS-CoV-2 wild-type, Alpha, Delta, and the emerging XBB variant. Meanwhile, A2binder demonstrates exceptional predictive performance on binding specificity for various epitopes and variants. Furthermore, by incorporating the attention mechanism inherent in the Roformer architecture into the PALM-H3 model, we improve its interpretability, providing crucial insights into the fundamental principles of antibody design.
© 2024. The Author(s).
Conflict of interest statement
H.H., B.H., L.G., Y.Z., T.L., and J.Y. are inventors on patent applications related to this work filed by Tencent Technology (Shenzhen) Company Ltd. (Chinese patent applications nos. 202310562554.X, 202311665813.8 and 202311682516.4). H.H., B.H., Y.Z., F.J., and J.Y. are employees of Tencent AI Lab. The authors declare no other competing interests.
Figures
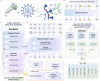
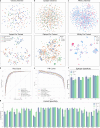
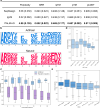
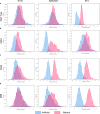
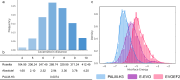
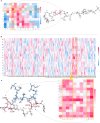
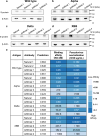
Similar articles
-
Structure-guided mutations in CDRs for enhancing the affinity of neutralizing SARS-CoV-2 nanobody.Biochem Biophys Res Commun. 2024 Nov 19;734:150746. doi: 10.1016/j.bbrc.2024.150746. Epub 2024 Sep 26. Biochem Biophys Res Commun. 2024. PMID: 39366179
-
Neutralizing Monoclonal Anti-SARS-CoV-2 Antibodies Isolated from Immunized Rabbits Define Novel Vulnerable Spike-Protein Epitope.Viruses. 2021 Mar 26;13(4):566. doi: 10.3390/v13040566. Viruses. 2021. PMID: 33810465 Free PMC article.
-
Genetic and structural basis for SARS-CoV-2 variant neutralization by a two-antibody cocktail.Nat Microbiol. 2021 Oct;6(10):1233-1244. doi: 10.1038/s41564-021-00972-2. Epub 2021 Sep 21. Nat Microbiol. 2021. PMID: 34548634 Free PMC article.
-
Analysis of the molecular mechanism of SARS-CoV-2 antibodies.Biochem Biophys Res Commun. 2021 Aug 20;566:45-52. doi: 10.1016/j.bbrc.2021.06.001. Epub 2021 Jun 5. Biochem Biophys Res Commun. 2021. PMID: 34116356 Free PMC article. Review.
-
Comprehensive Overview of Broadly Neutralizing Antibodies against SARS-CoV-2 Variants.Viruses. 2024 Jun 1;16(6):900. doi: 10.3390/v16060900. Viruses. 2024. PMID: 38932192 Free PMC article. Review.
Cited by
-
A deep drug prediction framework for viral infectious diseases using an optimizer-based ensemble of convolutional neural network: COVID-19 as a case study.Mol Divers. 2024 Oct 9. doi: 10.1007/s11030-024-11003-7. Online ahead of print. Mol Divers. 2024. PMID: 39379663
-
Advancements in mammalian display technology for therapeutic antibody development and beyond: current landscape, challenges, and future prospects.Front Immunol. 2024 Sep 24;15:1469329. doi: 10.3389/fimmu.2024.1469329. eCollection 2024. Front Immunol. 2024. PMID: 39381002 Free PMC article. Review.
-
PAbFold: Linear Antibody Epitope Prediction using AlphaFold2.bioRxiv [Preprint]. 2024 Dec 20:2024.04.19.590298. doi: 10.1101/2024.04.19.590298. bioRxiv. 2024. PMID: 38659833 Free PMC article. Preprint.
References
MeSH terms
Substances
Supplementary concepts
LinkOut - more resources
Full Text Sources
Medical
Miscellaneous