The genomic landscape of 2,023 colorectal cancers
- PMID: 39112709
- PMCID: PMC11374690
- DOI: 10.1038/s41586-024-07747-9
The genomic landscape of 2,023 colorectal cancers
Abstract
Colorectal carcinoma (CRC) is a common cause of mortality1, but a comprehensive description of its genomic landscape is lacking2-9. Here we perform whole-genome sequencing of 2,023 CRC samples from participants in the UK 100,000 Genomes Project, thereby providing a highly detailed somatic mutational landscape of this cancer. Integrated analyses identify more than 250 putative CRC driver genes, many not previously implicated in CRC or other cancers, including several recurrent changes outside the coding genome. We extend the molecular pathways involved in CRC development, define four new common subgroups of microsatellite-stable CRC based on genomic features and show that these groups have independent prognostic associations. We also characterize several rare molecular CRC subgroups, some with potential clinical relevance, including cancers with both microsatellite and chromosomal instability. We demonstrate a spectrum of mutational profiles across the colorectum, which reflect aetiological differences. These include the role of Escherichia colipks+ colibactin in rectal cancers10 and the importance of the SBS93 signature11-13, which suggests that diet or smoking is a risk factor. Immune-escape driver mutations14 are near-ubiquitous in hypermutant tumours and occur in about half of microsatellite-stable CRCs, often in the form of HLA copy number changes. Many driver mutations are actionable, including those associated with rare subgroups (for example, BRCA1 and IDH1), highlighting the role of whole-genome sequencing in optimizing patient care.
© 2024. The Author(s).
Conflict of interest statement
L.B.A. is a compensated consultant and has equity interest in io9. His spouse is an employee of Biotheranostics. L.B.A. is also an inventor of a US Patent 10,776,718 for source identification by non-negative matrix factorization. L.B.A. declares US provisional applications with the following serial numbers: 63/289,601; 63/269,033; 63/366,392; 63/367,846; 63/412,835. A.J.C. is an employee of Owkin UK Ltd. All other authors declare they have no known competing financial interests or personal relationships that could have appeared to influence the work reported in this paper.
Figures
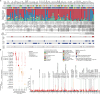
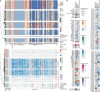
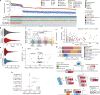
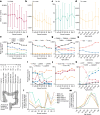
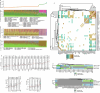
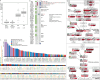
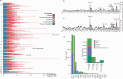
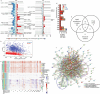
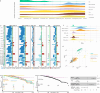
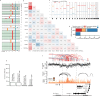
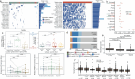
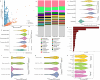
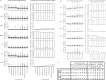
Similar articles
-
Can a Liquid Biopsy Detect Circulating Tumor DNA With Low-passage Whole-genome Sequencing in Patients With a Sarcoma? A Pilot Evaluation.Clin Orthop Relat Res. 2025 Jan 1;483(1):39-48. doi: 10.1097/CORR.0000000000003161. Epub 2024 Jun 21. Clin Orthop Relat Res. 2025. PMID: 38905450
-
Depressing time: Waiting, melancholia, and the psychoanalytic practice of care.In: Kirtsoglou E, Simpson B, editors. The Time of Anthropology: Studies of Contemporary Chronopolitics. Abingdon: Routledge; 2020. Chapter 5. In: Kirtsoglou E, Simpson B, editors. The Time of Anthropology: Studies of Contemporary Chronopolitics. Abingdon: Routledge; 2020. Chapter 5. PMID: 36137063 Free Books & Documents. Review.
-
Exploring the impact of housing insecurity on the health and well-being of children and young people: a systematic review.Public Health Res (Southampt). 2023 Dec;11(13):1-71. doi: 10.3310/TWWL4501. Public Health Res (Southampt). 2023. PMID: 39789922
-
Defining the optimum strategy for identifying adults and children with coeliac disease: systematic review and economic modelling.Health Technol Assess. 2022 Oct;26(44):1-310. doi: 10.3310/ZUCE8371. Health Technol Assess. 2022. PMID: 36321689 Free PMC article.
-
Impact of residual disease as a prognostic factor for survival in women with advanced epithelial ovarian cancer after primary surgery.Cochrane Database Syst Rev. 2022 Sep 26;9(9):CD015048. doi: 10.1002/14651858.CD015048.pub2. Cochrane Database Syst Rev. 2022. PMID: 36161421 Free PMC article. Review.
Cited by
-
Two Decades of Progress in Personalized Medicine of Colorectal Cancer in Serbia-Insights from the Institute for Oncology and Radiology of Serbia.Biomedicines. 2024 Oct 8;12(10):2278. doi: 10.3390/biomedicines12102278. Biomedicines. 2024. PMID: 39457591 Free PMC article.
-
A comprehensive comparison of tools for fitting mutational signatures.Nat Commun. 2024 Nov 2;15(1):9467. doi: 10.1038/s41467-024-53711-6. Nat Commun. 2024. PMID: 39487150 Free PMC article.
-
Characterizing the genomic landscape of colorectal cancer.Nat Rev Gastroenterol Hepatol. 2024 Oct;21(10):667. doi: 10.1038/s41575-024-00988-z. Nat Rev Gastroenterol Hepatol. 2024. PMID: 39251707 No abstract available.
-
Mutational Signatures in Colorectal Cancer: Translational Insights, Clinical Applications, and Limitations.Cancers (Basel). 2024 Aug 24;16(17):2956. doi: 10.3390/cancers16172956. Cancers (Basel). 2024. PMID: 39272814 Free PMC article. Review.
-
The ATOM-Seq sequence capture panel can accurately predict microsatellite instability status in formalin-fixed tumour samples, alongside routine gene mutation testing.Sci Rep. 2024 Sep 19;14(1):21870. doi: 10.1038/s41598-024-72419-7. Sci Rep. 2024. PMID: 39300198 Free PMC article.
References
-
- Grasso, C. S. et al. Genetic mechanisms of immune evasion in colorectal cancer. Cancer Discov.8, 730–749 (2018). 10.1158/2159-8290.CD-17-1327 - DOI - PMC - PubMed
MeSH terms
Substances
Grants and funding
LinkOut - more resources
Full Text Sources
Medical
Research Materials
Miscellaneous