Automatic Annotation Diagnostic Framework for Nasopharyngeal Carcinoma via Pathology-Fidelity GAN and Prior-Driven Classification
- PMID: 39061821
- PMCID: PMC11273917
- DOI: 10.3390/bioengineering11070739
Automatic Annotation Diagnostic Framework for Nasopharyngeal Carcinoma via Pathology-Fidelity GAN and Prior-Driven Classification
Abstract
Non-keratinizing carcinoma is the most common subtype of nasopharyngeal carcinoma (NPC). Its poorly differentiated tumor cells and complex microenvironment present challenges to pathological diagnosis. AI-based pathological models have demonstrated potential in diagnosing NPC, but the reliance on costly manual annotation hinders development. To address the challenges, this paper proposes a deep learning-based framework for diagnosing NPC without manual annotation. The framework includes a novel unpaired generative network and a prior-driven image classification system. With pathology-fidelity constraints, the generative network achieves accurate digital staining from H&E to EBER images. The classification system leverages staining specificity and pathological prior knowledge to annotate training data automatically and to classify images for NPC diagnosis. This work used 232 cases for study. The experimental results show that the classification system reached a 99.59% accuracy in classifying EBER images, which closely matched the diagnostic results of pathologists. Utilizing PF-GAN as the backbone of the framework, the system attained a specificity of 0.8826 in generating EBER images, markedly outperforming that of other GANs (0.6137, 0.5815). Furthermore, the F1-Score of the framework for patch level diagnosis was 0.9143, exceeding those of fully supervised models (0.9103, 0.8777). To further validate its clinical efficacy, the framework was compared with experienced pathologists at the WSI level, showing comparable NPC diagnosis performance. This low-cost and precise diagnostic framework optimizes the early pathological diagnosis method for NPC and provides an innovative strategic direction for AI-based cancer diagnosis.
Keywords: deep learning; digital staining; nasopharyngeal carcinoma; pathological diagnosis framework; pathology–fidelity.
Conflict of interest statement
Qiang Huang is currently employed by Shenzhen Shengqiang Technology Co., Ltd. and was employed by Shenzhen Shengqiang Technology Co., Ltd. while contributing to this manuscript. His contributions to this work and manuscript were made independently without any requirement, guidance or input by my employer. He received no financial compensation from any source for the contributions he made to this scientific work and manuscript.
Figures
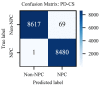
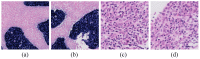
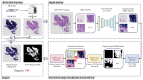
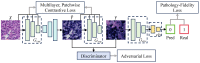
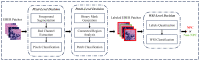
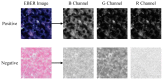
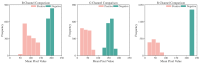
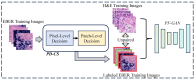
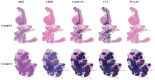
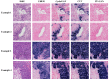
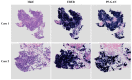
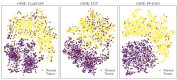
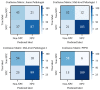
Similar articles
-
The role of unpaired image-to-image translation for stain color normalization in colorectal cancer histology classification.Comput Methods Programs Biomed. 2023 Jun;234:107511. doi: 10.1016/j.cmpb.2023.107511. Epub 2023 Mar 26. Comput Methods Programs Biomed. 2023. PMID: 37011426
-
Immunohistochemical Stain-Aided Annotation Accelerates Machine Learning and Deep Learning Model Development in the Pathologic Diagnosis of Nasopharyngeal Carcinoma.Diagnostics (Basel). 2023 Dec 18;13(24):3685. doi: 10.3390/diagnostics13243685. Diagnostics (Basel). 2023. PMID: 38132269 Free PMC article.
-
Recognizing basal cell carcinoma on smartphone-captured digital histopathology images with a deep neural network.Br J Dermatol. 2020 Mar;182(3):754-762. doi: 10.1111/bjd.18026. Epub 2019 Aug 22. Br J Dermatol. 2020. PMID: 31017653
-
Application of Artificial Intelligence to the Diagnosis and Therapy of Nasopharyngeal Carcinoma.J Clin Med. 2023 Apr 24;12(9):3077. doi: 10.3390/jcm12093077. J Clin Med. 2023. PMID: 37176518 Free PMC article. Review.
-
Generative Adversarial Networks in Digital Histopathology: Current Applications, Limitations, Ethical Considerations, and Future Directions.Mod Pathol. 2024 Jan;37(1):100369. doi: 10.1016/j.modpat.2023.100369. Epub 2023 Oct 27. Mod Pathol. 2024. PMID: 37890670 Review.
References
-
- Jen C.W., Tsai Y.C., Wu J.S., Chen P.L., Yen J.H., Chuang W.K., Cheng S.H. Prognostic classification for patients with nasopharyngeal carcinoma based on American Joint Committee on Cancer staging system T and N categories. Ther. Radiol. Oncol. 2020;4:2. doi: 10.21037/tro.2020.02.01. - DOI
-
- Yoshizaki T., Kondo S., Dochi H., Kobayashi E., Mizokami H., Komura S., Endo K. Recent Advances in Assessing the Clinical Implications of Epstein-Barr Virus Infection and Their Application to the Diagnosis and Treatment of Nasopharyngeal Carcinoma. Microorganisms. 2023;12:14. doi: 10.3390/microorganisms12010014. - DOI - PMC - PubMed
Grants and funding
LinkOut - more resources
Full Text Sources