Geometric epitope and paratope prediction
- PMID: 38984742
- PMCID: PMC11245313
- DOI: 10.1093/bioinformatics/btae405
Geometric epitope and paratope prediction
Abstract
Motivation: Identifying the binding sites of antibodies is essential for developing vaccines and synthetic antibodies. In this article, we investigate the optimal representation for predicting the binding sites in the two molecules and emphasize the importance of geometric information.
Results: Specifically, we compare different geometric deep learning methods applied to proteins' inner (I-GEP) and outer (O-GEP) structures. We incorporate 3D coordinates and spectral geometric descriptors as input features to fully leverage the geometric information. Our research suggests that different geometrical representation information is useful for different tasks. Surface-based models are more efficient in predicting the binding of the epitope, while graph models are better in paratope prediction, both achieving significant performance improvements. Moreover, we analyze the impact of structural changes in antibodies and antigens resulting from conformational rearrangements or reconstruction errors. Through this investigation, we showcase the robustness of geometric deep learning methods and spectral geometric descriptors to such perturbations.
Availability and implementation: The python code for the models, together with the data and the processing pipeline, is open-source and available at https://github.com/Marco-Peg/GEP.
© The Author(s) 2024. Published by Oxford University Press.
Conflict of interest statement
None declared.
Figures
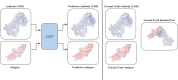
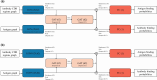
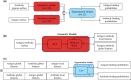
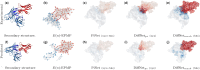
Similar articles
-
Machine-learning-based structural analysis of interactions between antibodies and antigens.Biosystems. 2024 Sep;243:105264. doi: 10.1016/j.biosystems.2024.105264. Epub 2024 Jul 2. Biosystems. 2024. PMID: 38964652
-
A spatial-temporal gated attention module for molecular property prediction based on molecular geometry.Brief Bioinform. 2021 Sep 2;22(5):bbab078. doi: 10.1093/bib/bbab078. Brief Bioinform. 2021. PMID: 33822856
-
Parapred: antibody paratope prediction using convolutional and recurrent neural networks.Bioinformatics. 2018 Sep 1;34(17):2944-2950. doi: 10.1093/bioinformatics/bty305. Bioinformatics. 2018. PMID: 29672675
-
Prediction of Paratope-Epitope Pairs Using Convolutional Neural Networks.Int J Mol Sci. 2024 May 16;25(10):5434. doi: 10.3390/ijms25105434. Int J Mol Sci. 2024. PMID: 38791470 Free PMC article.
-
A compact vocabulary of paratope-epitope interactions enables predictability of antibody-antigen binding.Cell Rep. 2021 Mar 16;34(11):108856. doi: 10.1016/j.celrep.2021.108856. Cell Rep. 2021. PMID: 33730590
References
-
- Cia G, Pucci F, Rooman M.. Critical review of conformational b-cell epitope prediction methods. Brief Bioinform 2023;24:bbac567. - PubMed
-
- da Silva BM, Myung Y, Ascher DB. et al. epitope3d: a machine learning method for conformational b-cell epitope prediction. Brief Bioinform 2022;23:bbab423. - PubMed
-
- Deac A, Veličković P, Sormanni P.. Attentive cross-modal paratope prediction. J Comput Biol 2019;26:536–45. - PubMed
-
- Del Vecchio A, Deac A, Liò P. et al. Neural message passing for joint paratope-epitope prediction. ICML Workshop on Computational Biology 2022.
Publication types
MeSH terms
Substances
Grants and funding
LinkOut - more resources
Full Text Sources
Miscellaneous