Data-driven probabilistic definition of the low energy conformational states of protein residues
- PMID: 38984065
- PMCID: PMC11231583
- DOI: 10.1093/nargab/lqae082
Data-driven probabilistic definition of the low energy conformational states of protein residues
Abstract
Protein dynamics and related conformational changes are essential for their function but difficult to characterise and interpret. Amino acids in a protein behave according to their local energy landscape, which is determined by their local structural context and environmental conditions. The lowest energy state for a given residue can correspond to sharply defined conformations, e.g. in a stable helix, or can cover a wide range of conformations, e.g. in intrinsically disordered regions. A good definition of such low energy states is therefore important to describe the behaviour of a residue and how it changes with its environment. We propose a data-driven probabilistic definition of six low energy conformational states typically accessible for amino acid residues in proteins. This definition is based on solution NMR information of 1322 proteins through a combined analysis of structure ensembles with interpreted chemical shifts. We further introduce a conformational state variability parameter that captures, based on an ensemble of protein structures from molecular dynamics or other methods, how often a residue moves between these conformational states. The approach enables a different perspective on the local conformational behaviour of proteins that is complementary to their static interpretation from single structure models.
© The Author(s) 2024. Published by Oxford University Press on behalf of NAR Genomics and Bioinformatics.
Conflict of interest statement
None declared.
Figures
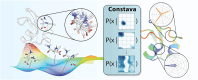
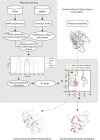
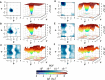
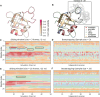
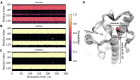
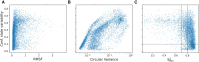
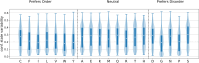
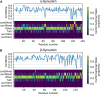
Similar articles
-
WASCO: A Wasserstein-based Statistical Tool to Compare Conformational Ensembles of Intrinsically Disordered Proteins.J Mol Biol. 2023 Jul 15;435(14):168053. doi: 10.1016/j.jmb.2023.168053. Epub 2023 Mar 18. J Mol Biol. 2023. PMID: 36934808
-
Spontaneous Switching among Conformational Ensembles in Intrinsically Disordered Proteins.Biomolecules. 2019 Mar 22;9(3):114. doi: 10.3390/biom9030114. Biomolecules. 2019. PMID: 30909517 Free PMC article. Review.
-
Measuring the hydrogen/deuterium exchange of proteins at high spatial resolution by mass spectrometry: overcoming gas-phase hydrogen/deuterium scrambling.Acc Chem Res. 2014 Oct 21;47(10):3018-27. doi: 10.1021/ar500194w. Epub 2014 Aug 29. Acc Chem Res. 2014. PMID: 25171396 Review.
-
Generating Intrinsically Disordered Protein Conformational Ensembles from a Database of Ramachandran Space Pair Residue Probabilities Using a Markov Chain.J Phys Chem B. 2018 Oct 4;122(39):9087-9101. doi: 10.1021/acs.jpcb.8b05797. Epub 2018 Sep 25. J Phys Chem B. 2018. PMID: 30204435
-
Capturing dynamic conformational shifts in protein-ligand recognition using integrative structural biology in solution.Emerg Top Life Sci. 2018 Apr 20;2(1):107-119. doi: 10.1042/ETLS20170090. Emerg Top Life Sci. 2018. PMID: 33525784
References
-
- Tompa P. Intrinsically disordered proteins: a 10-year recap. Trends Biochem. Sci. 2012; 37:509–516. - PubMed
-
- Sun Z., Liu Q., Qu G., Feng Y., Reetz M.T. Utility of B-factors in protein science: interpreting rigidity, flexibility, and internal motion and engineering thermostability. Chem. Rev. 2019; 119:1626–1665. - PubMed
LinkOut - more resources
Full Text Sources