SVDF: enhancing structural variation detect from long-read sequencing via automatic filtering strategies
- PMID: 38980375
- PMCID: PMC11232458
- DOI: 10.1093/bib/bbae336
SVDF: enhancing structural variation detect from long-read sequencing via automatic filtering strategies
Abstract
Structural variation (SV) is an important form of genomic variation that influences gene function and expression by altering the structure of the genome. Although long-read data have been proven to better characterize SVs, SVs detected from noisy long-read data still include a considerable portion of false-positive calls. To accurately detect SVs in long-read data, we present SVDF, a method that employs a learning-based noise filtering strategy and an SV signature-adaptive clustering algorithm, for effectively reducing the likelihood of false-positive events. Benchmarking results from multiple orthogonal experiments demonstrate that, across different sequencing platforms and depths, SVDF achieves higher calling accuracy for each sample compared to several existing general SV calling tools. We believe that, with its meticulous and sensitive SV detection capability, SVDF can bring new opportunities and advancements to cutting-edge genomic research.
Keywords: deep learning; false-positives; long-read sequencing; structural variation detection.
© The Author(s) 2024. Published by Oxford University Press.
Figures
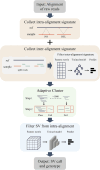
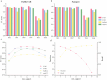
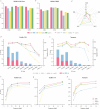
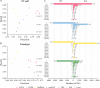
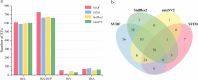
Similar articles
-
Comparison of multiple algorithms to reliably detect structural variants in pears.BMC Genomics. 2020 Jan 20;21(1):61. doi: 10.1186/s12864-020-6455-x. BMC Genomics. 2020. PMID: 31959124 Free PMC article.
-
A Comparison of Structural Variant Calling from Short-Read and Nanopore-Based Whole-Genome Sequencing Using Optical Genome Mapping as a Benchmark.Genes (Basel). 2024 Jul 16;15(7):925. doi: 10.3390/genes15070925. Genes (Basel). 2024. PMID: 39062704 Free PMC article.
-
rMFilter: acceleration of long read-based structure variation calling by chimeric read filtering.Bioinformatics. 2017 Sep 1;33(17):2750-2752. doi: 10.1093/bioinformatics/btx279. Bioinformatics. 2017. PMID: 28482046
-
A survey of algorithms for the detection of genomic structural variants from long-read sequencing data.Nat Methods. 2023 Aug;20(8):1143-1158. doi: 10.1038/s41592-023-01932-w. Epub 2023 Jun 29. Nat Methods. 2023. PMID: 37386186 Free PMC article. Review.
-
Overview of structural variation calling: Simulation, identification, and visualization.Comput Biol Med. 2022 Jun;145:105534. doi: 10.1016/j.compbiomed.2022.105534. Epub 2022 Apr 15. Comput Biol Med. 2022. PMID: 35585730 Review.