Patient-specific computational models predict prognosis in B cell lymphoma by quantifying pro-proliferative and anti-apoptotic signatures from genetic sequencing data
- PMID: 38965209
- PMCID: PMC11224250
- DOI: 10.1038/s41408-024-01090-y
Patient-specific computational models predict prognosis in B cell lymphoma by quantifying pro-proliferative and anti-apoptotic signatures from genetic sequencing data
Abstract
Genetic heterogeneity and co-occurring driver mutations impact clinical outcomes in blood cancers, but predicting the emergent effect of co-occurring mutations that impact multiple complex and interacting signalling networks is challenging. Here, we used mathematical models to predict the impact of co-occurring mutations on cellular signalling and cell fates in diffuse large B cell lymphoma and multiple myeloma. Simulations predicted adverse impact on clinical prognosis when combinations of mutations induced both anti-apoptotic (AA) and pro-proliferative (PP) signalling. We integrated patient-specific mutational profiles into personalised lymphoma models, and identified patients characterised by simultaneous upregulation of anti-apoptotic and pro-proliferative (AAPP) signalling in all genomic and cell-of-origin classifications (8-25% of patients). In a discovery cohort and two validation cohorts, patients with upregulation of neither, one (AA or PP), or both (AAPP) signalling states had good, intermediate and poor prognosis respectively. Combining AAPP signalling with genetic or clinical prognostic predictors reliably stratified patients into striking prognostic categories. AAPP patients in poor prognosis genetic clusters had 7.8 months median overall survival, while patients lacking both features had 90% overall survival at 120 months in a validation cohort. Personalised computational models enable identification of novel risk-stratified patient subgroups, providing a valuable tool for future risk-adapted clinical trials.
© 2024. The Author(s).
Conflict of interest statement
The authors declare no competing interests.
Figures
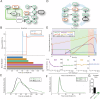
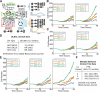
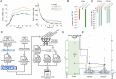
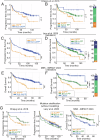
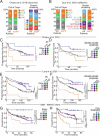
Similar articles
-
Impact of residual disease as a prognostic factor for survival in women with advanced epithelial ovarian cancer after primary surgery.Cochrane Database Syst Rev. 2022 Sep 26;9(9):CD015048. doi: 10.1002/14651858.CD015048.pub2. Cochrane Database Syst Rev. 2022. PMID: 36161421 Free PMC article. Review.
-
Comparison of Two Modern Survival Prediction Tools, SORG-MLA and METSSS, in Patients With Symptomatic Long-bone Metastases Who Underwent Local Treatment With Surgery Followed by Radiotherapy and With Radiotherapy Alone.Clin Orthop Relat Res. 2024 Dec 1;482(12):2193-2208. doi: 10.1097/CORR.0000000000003185. Epub 2024 Jul 23. Clin Orthop Relat Res. 2024. PMID: 39051924
-
Defining the optimum strategy for identifying adults and children with coeliac disease: systematic review and economic modelling.Health Technol Assess. 2022 Oct;26(44):1-310. doi: 10.3310/ZUCE8371. Health Technol Assess. 2022. PMID: 36321689 Free PMC article.
-
Depressing time: Waiting, melancholia, and the psychoanalytic practice of care.In: Kirtsoglou E, Simpson B, editors. The Time of Anthropology: Studies of Contemporary Chronopolitics. Abingdon: Routledge; 2020. Chapter 5. In: Kirtsoglou E, Simpson B, editors. The Time of Anthropology: Studies of Contemporary Chronopolitics. Abingdon: Routledge; 2020. Chapter 5. PMID: 36137063 Free Books & Documents. Review.
-
Falls prevention interventions for community-dwelling older adults: systematic review and meta-analysis of benefits, harms, and patient values and preferences.Syst Rev. 2024 Nov 26;13(1):289. doi: 10.1186/s13643-024-02681-3. Syst Rev. 2024. PMID: 39593159 Free PMC article.
References
-
- Mosquera Orgueira A, Ferreiro Ferro R, Díaz Arias J, Aliste Santos C, Antelo Rodríguez B, Bao Pérez L, et al. Detection of new drivers of frequent B-cell lymphoid neoplasms using an integrated analysis of whole genomes. PLoS One. 2021;16:e0248886. doi: 10.1371/journal.pone.0248886. - DOI - PMC - PubMed
Publication types
MeSH terms
Grants and funding
LinkOut - more resources
Full Text Sources
Miscellaneous