Revolutionary Point-of-Care Wearable Diagnostics for Early Disease Detection and Biomarker Discovery through Intelligent Technologies
- PMID: 38958517
- PMCID: PMC11423253
- DOI: 10.1002/advs.202400595
Revolutionary Point-of-Care Wearable Diagnostics for Early Disease Detection and Biomarker Discovery through Intelligent Technologies
Abstract
Early-stage disease detection, particularly in Point-Of-Care (POC) wearable formats, assumes pivotal role in advancing healthcare services and precision-medicine. Public benefits of early detection extend beyond cost-effectively promoting healthcare outcomes, to also include reducing the risk of comorbid diseases. Technological advancements enabling POC biomarker recognition empower discovery of new markers for various health conditions. Integration of POC wearables for biomarker detection with intelligent frameworks represents ground-breaking innovations enabling automation of operations, conducting advanced large-scale data analysis, generating predictive models, and facilitating remote and guided clinical decision-making. These advancements substantially alleviate socioeconomic burdens, creating a paradigm shift in diagnostics, and revolutionizing medical assessments and technology development. This review explores critical topics and recent progress in development of 1) POC systems and wearable solutions for early disease detection and physiological monitoring, as well as 2) discussing current trends in adoption of smart technologies within clinical settings and in developing biological assays, and ultimately 3) exploring utilities of POC systems and smart platforms for biomarker discovery. Additionally, the review explores technology translation from research labs to broader applications. It also addresses associated risks, biases, and challenges of widespread Artificial Intelligence (AI) integration in diagnostics systems, while systematically outlining potential prospects, current challenges, and opportunities.
Keywords: AI‐assisted biomarker discovery; high‐throughput omics and clinical study; intelligent diagnostics; point‐of‐care biomarker detection platforms; precision medicine; remote disease detection; wearable health monitoring.
© 2024 The Author(s). Advanced Science published by Wiley‐VCH GmbH.
Conflict of interest statement
The authors declare no conflict of interest.
Figures
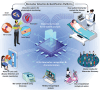
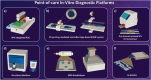
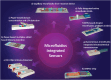
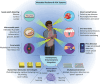
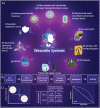
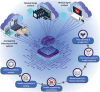
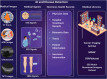
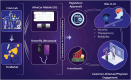
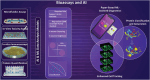
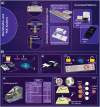
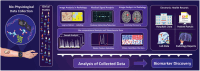
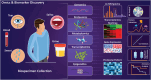
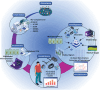
Similar articles
-
AI-Assisted Detection of Biomarkers by Sensors and Biosensors for Early Diagnosis and Monitoring.Biosensors (Basel). 2024 Jul 22;14(7):356. doi: 10.3390/bios14070356. Biosensors (Basel). 2024. PMID: 39056632 Free PMC article. Review.
-
Metal Organic Frameworks Based Wearable and Point-of-Care Electrochemical Sensors for Healthcare Monitoring.Biosensors (Basel). 2024 Oct 10;14(10):492. doi: 10.3390/bios14100492. Biosensors (Basel). 2024. PMID: 39451704 Free PMC article. Review.
-
Microfluidic Point-of-Care Devices: New Trends and Future Prospects for eHealth Diagnostics.Sensors (Basel). 2020 Mar 31;20(7):1951. doi: 10.3390/s20071951. Sensors (Basel). 2020. PMID: 32244343 Free PMC article.
-
A Comprehensive Review on Advancements in Wearable Technologies: Revolutionizing Cardiovascular Medicine.Cureus. 2024 May 29;16(5):e61312. doi: 10.7759/cureus.61312. eCollection 2024 May. Cureus. 2024. PMID: 38947726 Free PMC article. Review.
-
Wearable Technologies and AI at the Far Edge for Chronic Heart Failure Prevention and Management: A Systematic Review and Prospects.Sensors (Basel). 2023 Aug 3;23(15):6896. doi: 10.3390/s23156896. Sensors (Basel). 2023. PMID: 37571678 Free PMC article. Review.
References
-
- Balogh E. P., Miller B. T., Ball J. R., Improving Diagnosis in Health Care, National Academies Press (US), Washington (DC) 2015. - PubMed
-
- Black G. B., Lyratzopoulos G., Vincent C. A., Fulop N. J., Nicholson B. D., BMJ 2023, 380, 071225.
-
- Vinood P., Victor P., Textbook of Cancer Epidemiology, Springer, Dordrecht, The Netherlands: 2015.
Publication types
MeSH terms
Substances
Grants and funding
LinkOut - more resources
Full Text Sources