Identification and validation of immunity- and disulfidptosis-related genes signature for predicting prognosis in ovarian cancer
- PMID: 38952356
- PMCID: PMC11215265
- DOI: 10.1016/j.heliyon.2024.e32273
Identification and validation of immunity- and disulfidptosis-related genes signature for predicting prognosis in ovarian cancer
Abstract
Background: Ovarian cancer (OC) ranks as the fifth most prevalent neoplasm in women and exhibits an unfavorable prognosis. To improve the OC patient's prognosis, a pioneering risk signature was formulated by amalgamating disulfidptosis-related genes.
Methods: A comparative analysis of OC tissues and normal tissues was carried out, and differentially expressed disulfidptosis-related genes (DRGs) were found using the criteria of |log2 (fold change) | > 0.585 and adjusted P-value <0.05. Subsequently, the TCGA training set was utilized to create a prognostic risk signature, which was validated by employing both the TCGA testing set and the GEO dataset. Moreover, the immune cell infiltration, mutational load, response to chemotherapy, and response to immunotherapy were analyzed. To further validate these findings, QRT-PCR analysis was conducted on ovarian tumor cell lines.
Results: A risk signature was created using fourteen differentially expressed genes (DEGs) associated with disulfidptosis, enabling the classification of ovarian cancer (OC) patients into high-risk group (HRG) and low-risk group (LRG). The HRG exhibited a lower overall survival (OS) compared to the LRG. In addition, the risk score remained an independent predictor even after incorporating clinical factors. Furthermore, the LRG displayed lower stromal, immune, and estimated scores compared to the HRG, suggesting a possible connection between the risk signature, immune cell infiltration, and mutational load. Finally, the QRT-PCR experiments revealed that eight genes were upregulated in the human OC cell line SKOV3 compared with the human normal OC line IOSE80, while six genes were down-regulated.
Conclusions: A fourteen-biomarker signature composed of disulfidptosis-related genes could serve as a valuable risk stratification tool in OC, facilitating the identification of patients who may benefit from individualized treatment and follow-up management.
Keywords: Bioinformatics; Disulfidptosis; Disulfidptosis-related genes (DRGs); Immunity; Ovarian cancer.
© 2024 The Authors. Published by Elsevier Ltd.
Conflict of interest statement
The authors declare that they have no known competing financial interests or personal relationships that could have appeared to influence the work reported in this paper.
Figures
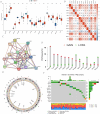
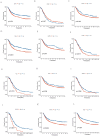
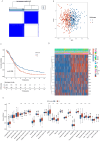
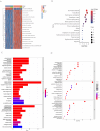
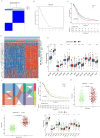
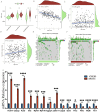
Similar articles
-
Bioinformatics-based analysis of the relationship between disulfidptosis and prognosis and treatment response in pancreatic cancer.Sci Rep. 2023 Dec 14;13(1):22218. doi: 10.1038/s41598-023-49752-4. Sci Rep. 2023. PMID: 38097783 Free PMC article. Clinical Trial.
-
Identification of Disulfidptosis-Related LncRNA Subtypes, Establishment of a Prognostic Signature, and Characterization of Immune Infiltration in Ovarian Cancer.Comb Chem High Throughput Screen. 2024 Oct 3. doi: 10.2174/0113862073326170240923061119. Online ahead of print. Comb Chem High Throughput Screen. 2024. PMID: 39364865
-
Disulfidptosis characterizes the tumor microenvironment and predicts immunotherapy sensitivity and prognosis in bladder cancer.Heliyon. 2024 Feb 5;10(3):e25573. doi: 10.1016/j.heliyon.2024.e25573. eCollection 2024 Feb 15. Heliyon. 2024. PMID: 38356551 Free PMC article.
-
Identification of immunity- and ferroptosis-related genes for predicting the prognosis of serous ovarian cancer.Gene. 2022 Sep 5;838:146701. doi: 10.1016/j.gene.2022.146701. Epub 2022 Jun 28. Gene. 2022. PMID: 35777713
-
Identification of disulfidptosis-related clusters and construction of a disulfidptosis-related gene prognostic signature in triple-negative breast cancer.Heliyon. 2024 Jun 14;10(12):e33092. doi: 10.1016/j.heliyon.2024.e33092. eCollection 2024 Jun 30. Heliyon. 2024. PMID: 38994057 Free PMC article.
Cited by
-
The role of molecular subtypes and immune infiltration characteristics based on disulfidptosis-related genes in ovarian cancer.Discov Oncol. 2024 Oct 28;15(1):596. doi: 10.1007/s12672-024-01489-w. Discov Oncol. 2024. PMID: 39467928 Free PMC article.
References
LinkOut - more resources
Full Text Sources
Miscellaneous