Integrated Single-cell Multiomic Analysis of HIV Latency Reversal Reveals Novel Regulators of Viral Reactivation
- PMID: 38902848
- PMCID: PMC11189801
- DOI: 10.1093/gpbjnl/qzae003
Integrated Single-cell Multiomic Analysis of HIV Latency Reversal Reveals Novel Regulators of Viral Reactivation
Abstract
Despite the success of antiretroviral therapy, human immunodeficiency virus (HIV) cannot be cured because of a reservoir of latently infected cells that evades therapy. To understand the mechanisms of HIV latency, we employed an integrated single-cell RNA sequencing (scRNA-seq) and single-cell assay for transposase-accessible chromatin with sequencing (scATAC-seq) approach to simultaneously profile the transcriptomic and epigenomic characteristics of ∼ 125,000 latently infected primary CD4+ T cells after reactivation using three different latency reversing agents. Differentially expressed genes and differentially accessible motifs were used to examine transcriptional pathways and transcription factor (TF) activities across the cell population. We identified cellular transcripts and TFs whose expression/activity was correlated with viral reactivation and demonstrated that a machine learning model trained on these data was 75%-79% accurate at predicting viral reactivation. Finally, we validated the role of two candidate HIV-regulating factors, FOXP1 and GATA3, in viral transcription. These data demonstrate the power of integrated multimodal single-cell analysis to uncover novel relationships between host cell factors and HIV latency.
Keywords: HIV latency reversal; HIV-regulating factor; Machine learning; Primary CD4+ T cell; Single-cell multiomics.
© The Author(s) 2024. Published by Oxford University Press and Science Press on behalf of the Beijing Institute of Genomics, Chinese Academy of Sciences / China National Center for Bioinformation and Genetics Society of China.
Conflict of interest statement
The authors have declared no competing interests.
Figures
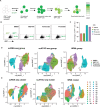
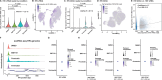
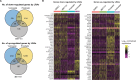
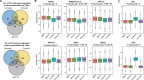
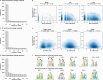
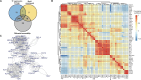
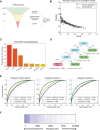
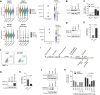
Similar articles
-
Posttranscriptional Regulation of HIV-1 Gene Expression during Replication and Reactivation from Latency by Nuclear Matrix Protein MATR3.mBio. 2018 Nov 13;9(6):e02158-18. doi: 10.1128/mBio.02158-18. mBio. 2018. PMID: 30425153 Free PMC article.
-
Epitranscriptomic m6A modifications during reactivation of HIV-1 latency in CD4+ T cells.mBio. 2024 Nov 13;15(11):e0221424. doi: 10.1128/mbio.02214-24. Epub 2024 Oct 7. mBio. 2024. PMID: 39373537 Free PMC article.
-
Naf1 Regulates HIV-1 Latency by Suppressing Viral Promoter-Driven Gene Expression in Primary CD4+ T Cells.J Virol. 2016 Dec 16;91(1):e01830-16. doi: 10.1128/JVI.01830-16. Print 2017 Jan 1. J Virol. 2016. PMID: 27795436 Free PMC article.
-
Leveraging Novel Integrated Single-Cell Analyses to Define HIV-1 Latency Reversal.Viruses. 2021 Jun 22;13(7):1197. doi: 10.3390/v13071197. Viruses. 2021. PMID: 34206546 Free PMC article. Review.
-
Underlying mechanisms of HIV-1 latency.Virus Genes. 2017 Jun;53(3):329-339. doi: 10.1007/s11262-017-1443-1. Epub 2017 Mar 3. Virus Genes. 2017. PMID: 28258391 Review.
References
-
- Finzi D, Hermankova M, Pierson T, Carruth LM, Buck C, Chaisson RE, et al.Identification of a reservoir for HIV-1 in patients on highly active antiretroviral therapy. Science 1997;278:1295–300. - PubMed
-
- Siliciano JD, Kajdas J, Finzi D, Quinn TC, Chadwick K, Margolick JB, et al.Long-term follow-up studies confirm the stability of the latent reservoir for HIV-1 in resting CD4+ T cells. Nat Med 2003;9:727–8. - PubMed
Publication types
MeSH terms
Substances
Grants and funding
LinkOut - more resources
Full Text Sources
Research Materials
Miscellaneous