Potential inhibitors of VEGFR1, VEGFR2, and VEGFR3 developed through Deep Learning for the treatment of Cervical Cancer
- PMID: 38858458
- PMCID: PMC11164920
- DOI: 10.1038/s41598-024-63762-w
Potential inhibitors of VEGFR1, VEGFR2, and VEGFR3 developed through Deep Learning for the treatment of Cervical Cancer
Abstract
Cervical cancer stands as a prevalent gynaecologic malignancy affecting women globally, often linked to persistent human papillomavirus infection. Biomarkers associated with cervical cancer, including VEGF-A, VEGF-B, VEGF-C, VEGF-D, and VEGF-E, show upregulation and are linked to angiogenesis and lymphangiogenesis. This research aims to employ in-silico methods to target tyrosine kinase receptor proteins-VEGFR-1, VEGFR-2, and VEGFR-3, and identify novel inhibitors for Vascular Endothelial Growth Factors receptors (VEGFRs). A comprehensive literary study was conducted which identified 26 established inhibitors for VEGFR-1, VEGFR-2, and VEGFR-3 receptor proteins. Compounds with high-affinity scores, including PubChem ID-25102847, 369976, and 208908 were chosen from pre-existing compounds for creating Deep Learning-based models. RD-Kit, a Deep learning algorithm, was used to generate 43 million compounds for VEGFR-1, VEGFR-2, and VEGFR-3 targets. Molecular docking studies were conducted on the top 10 molecules for each target to validate the receptor-ligand binding affinity. The results of Molecular Docking indicated that PubChem IDs-71465,645 and 11152946 exhibited strong affinity, designating them as the most efficient molecules. To further investigate their potential, a Molecular Dynamics Simulation was performed to assess conformational stability, and a pharmacophore analysis was also conducted for indoctrinating interactions.
Keywords: ADMET studies; Deep learning; Machine-learning; Molecular docking; Molecular dynamics simulation; Python; R programming; VEGFR inhibitors.
© 2024. The Author(s).
Conflict of interest statement
The authors declare no competing interests.
Figures
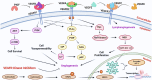
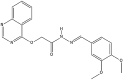
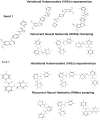
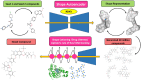
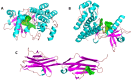
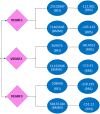
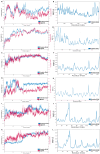
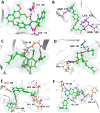
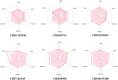
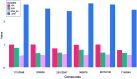
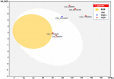
Similar articles
-
Discovery of fruquintinib, a potent and highly selective small molecule inhibitor of VEGFR 1, 2, 3 tyrosine kinases for cancer therapy.Cancer Biol Ther. 2014;15(12):1635-45. doi: 10.4161/15384047.2014.964087. Cancer Biol Ther. 2014. PMID: 25482937 Free PMC article.
-
Computational Investigation of 1, 3, 4 Oxadiazole Derivatives as Lead Inhibitors of VEGFR 2 in Comparison with EGFR: Density Functional Theory, Molecular Docking and Molecular Dynamics Simulation Studies.Biomolecules. 2022 Nov 1;12(11):1612. doi: 10.3390/biom12111612. Biomolecules. 2022. PMID: 36358960 Free PMC article.
-
Sunitinib inhibits lymphatic endothelial cell functions and lymph node metastasis in a breast cancer model through inhibition of vascular endothelial growth factor receptor 3.Breast Cancer Res. 2011 Jun 21;13(3):R66. doi: 10.1186/bcr2903. Breast Cancer Res. 2011. PMID: 21693010 Free PMC article.
-
Vascular endothelial growth factor and its receptor system: physiological functions in angiogenesis and pathological roles in various diseases.J Biochem. 2013 Jan;153(1):13-9. doi: 10.1093/jb/mvs136. Epub 2012 Nov 21. J Biochem. 2013. PMID: 23172303 Free PMC article. Review.
-
Therapeutic implication of vascular endothelial growth factor receptor-1 (VEGFR-1) targeting in cancer cells and tumor microenvironment by competitive and non-competitive inhibitors.Pharmacol Res. 2018 Oct;136:97-107. doi: 10.1016/j.phrs.2018.08.023. Epub 2018 Aug 28. Pharmacol Res. 2018. PMID: 30170190 Review.
Cited by
-
VEGF-Virus Interactions: Pathogenic Mechanisms and Therapeutic Applications.Cells. 2024 Nov 4;13(21):1815. doi: 10.3390/cells13211815. Cells. 2024. PMID: 39513922 Free PMC article. Review.
References
MeSH terms
Substances
LinkOut - more resources
Full Text Sources
Medical
Miscellaneous