Impact of genome build on RNA-seq interpretation and diagnostics
- PMID: 38834072
- PMCID: PMC11267525
- DOI: 10.1016/j.ajhg.2024.05.005
Impact of genome build on RNA-seq interpretation and diagnostics
Abstract
Transcriptomics is a powerful tool for unraveling the molecular effects of genetic variants and disease diagnosis. Prior studies have demonstrated that choice of genome build impacts variant interpretation and diagnostic yield for genomic analyses. To identify the extent genome build also impacts transcriptomics analyses, we studied the effect of the hg19, hg38, and CHM13 genome builds on expression quantification and outlier detection in 386 rare disease and familial control samples from both the Undiagnosed Diseases Network and Genomics Research to Elucidate the Genetics of Rare Disease Consortium. Across six routinely collected biospecimens, 61% of quantified genes were not influenced by genome build. However, we identified 1,492 genes with build-dependent quantification, 3,377 genes with build-exclusive expression, and 9,077 genes with annotation-specific expression across six routinely collected biospecimens, including 566 clinically relevant and 512 known OMIM genes. Further, we demonstrate that between builds for a given gene, a larger difference in quantification is well correlated with a larger change in expression outlier calling. Combined, we provide a database of genes impacted by build choice and recommend that transcriptomics-guided analyses and diagnoses are cross referenced with these data for robustness.
Keywords: RNA-seq; genome build; rare disease.
Copyright © 2024 American Society of Human Genetics. Published by Elsevier Inc. All rights reserved.
Conflict of interest statement
Declaration of interests During this project R.A.U. was employed for an internship by Vertex Pharmaceuticals. P.C.G. is a consultant for BioMarin. S.B.M. is an advisor to BioMarin, MyOme, and Tenaya Therapeutics.
Figures
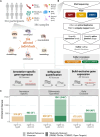
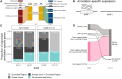
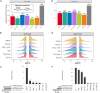
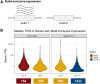
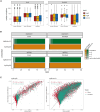
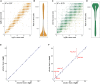
Update of
-
Impact of genome build on RNA-seq interpretation and diagnostics.medRxiv [Preprint]. 2024 Jan 12:2024.01.11.24301165. doi: 10.1101/2024.01.11.24301165. medRxiv. 2024. Update in: Am J Hum Genet. 2024 Jul 11;111(7):1282-1300. doi: 10.1016/j.ajhg.2024.05.005 PMID: 38260490 Free PMC article. Updated. Preprint.
Similar articles
-
Defining the optimum strategy for identifying adults and children with coeliac disease: systematic review and economic modelling.Health Technol Assess. 2022 Oct;26(44):1-310. doi: 10.3310/ZUCE8371. Health Technol Assess. 2022. PMID: 36321689 Free PMC article.
-
Can a Liquid Biopsy Detect Circulating Tumor DNA With Low-passage Whole-genome Sequencing in Patients With a Sarcoma? A Pilot Evaluation.Clin Orthop Relat Res. 2025 Jan 1;483(1):39-48. doi: 10.1097/CORR.0000000000003161. Epub 2024 Jun 21. Clin Orthop Relat Res. 2025. PMID: 38905450
-
Exploring the impact of housing insecurity on the health and well-being of children and young people: a systematic review.Public Health Res (Southampt). 2023 Dec;11(13):1-71. doi: 10.3310/TWWL4501. Public Health Res (Southampt). 2023. PMID: 39789922
-
Antibody tests for identification of current and past infection with SARS-CoV-2.Cochrane Database Syst Rev. 2022 Nov 17;11(11):CD013652. doi: 10.1002/14651858.CD013652.pub2. Cochrane Database Syst Rev. 2022. PMID: 36394900 Free PMC article. Review.
-
Depressing time: Waiting, melancholia, and the psychoanalytic practice of care.In: Kirtsoglou E, Simpson B, editors. The Time of Anthropology: Studies of Contemporary Chronopolitics. Abingdon: Routledge; 2020. Chapter 5. In: Kirtsoglou E, Simpson B, editors. The Time of Anthropology: Studies of Contemporary Chronopolitics. Abingdon: Routledge; 2020. Chapter 5. PMID: 36137063 Free Books & Documents. Review.
References
-
- Frankish A., Uszczynska B., Ritchie G.R.S., Gonzalez J.M., Pervouchine D., Petryszak R., Mudge J.M., Fonseca N., Brazma A., Guigo R., Harrow J. Comparison of GENCODE and RefSeq gene annotation and the impact of reference geneset on variant effect prediction. BMC Genom. 2015;16:S2. doi: 10.1186/1471-2164-16-S8-S2. - DOI - PMC - PubMed
MeSH terms
Grants and funding
LinkOut - more resources
Full Text Sources