Clinical Implications and Molecular Features of Extracellular Matrix Networks in Soft Tissue Sarcomas
- PMID: 38810090
- PMCID: PMC11292195
- DOI: 10.1158/1078-0432.CCR-23-3960
Clinical Implications and Molecular Features of Extracellular Matrix Networks in Soft Tissue Sarcomas
Abstract
Purpose: The landscape of extracellular matrix (ECM) alterations in soft tissue sarcomas (STS) remains poorly characterized. We aimed to investigate the tumor ECM and adhesion signaling networks present in STS and their clinical implications.
Experimental design: Proteomic and clinical data from 321 patients across 11 histological subtypes were analyzed to define ECM and integrin adhesion networks. Subgroup analysis was performed in leiomyosarcomas (LMS), dedifferentiated liposarcomas (DDLPS), and undifferentiated pleomorphic sarcomas (UPS).
Results: This analysis defined subtype-specific ECM profiles including enrichment of basement membrane proteins in LMS and ECM proteases in UPS. Across the cohort, we identified three distinct coregulated ECM networks which are associated with tumor malignancy grade and histological subtype. Comparative analysis of LMS cell line and patient proteomic data identified the lymphocyte cytosolic protein 1 cytoskeletal protein as a prognostic factor in LMS. Characterization of ECM network events in DDLPS revealed three subtypes with distinct oncogenic signaling pathways and survival outcomes. Evaluation of the DDLPS subtype with the poorest prognosis nominates ECM remodeling proteins as candidate antistromal therapeutic targets. Finally, we define a proteoglycan signature that is an independent prognostic factor for overall survival in DDLPS and UPS.
Conclusions: STS comprise heterogeneous ECM signaling networks and matrix-specific features that have utility for risk stratification and therapy selection, which could in future guide precision medicine in these rare cancers.
©2024 The Authors; Published by the American Association for Cancer Research.
Conflict of interest statement
A.T.J. Lee reports personal fees from Alexion, AstraZeneca, and Deciphera outside the submitted work. P. Chudasama reports grants and other support from the German Research Foundation and German Federal Ministry of Education and Research during the conduct of the study. R.L. Jones reports personal fees from Adaptimmune, Astex, Athenex, Bayer, BI, Blueprint, Clinigen, Eisai, Epizyme, Daichii, Deciphera, Immunedesign, Immunicum, Karma Oncology, Lilly, Merck, Mundipharma, Pharmamar, Springworks, SynOx, Tracon, and UpToDate outside the submitted work. P.H. Huang reports grants from Sarcoma UK, Cancer Research UK, Sarcoma Foundation of America, National Institute for Health Research, and Bristol Care Homes during the conduct of the study. No disclosures were reported by the other authors.
Figures
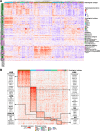
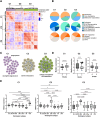
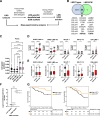
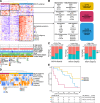
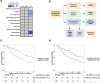
Similar articles
-
The proteomic landscape of soft tissue sarcomas.Nat Commun. 2023 Jun 29;14(1):3834. doi: 10.1038/s41467-023-39486-2. Nat Commun. 2023. PMID: 37386008 Free PMC article.
-
Gene expression profiling in leiomyosarcomas and undifferentiated pleomorphic sarcomas: SRC as a new diagnostic marker.PLoS One. 2014 Jul 16;9(7):e102281. doi: 10.1371/journal.pone.0102281. eCollection 2014. PLoS One. 2014. PMID: 25028927 Free PMC article.
-
Are peripheral purely undifferentiated pleomorphic sarcomas with MDM2 amplification dedifferentiated liposarcomas?Am J Surg Pathol. 2014 Mar;38(3):293-304. doi: 10.1097/PAS.0000000000000131. Am J Surg Pathol. 2014. PMID: 24525499
-
Establishing biomarkers for soft tissue sarcomas.Expert Rev Anticancer Ther. 2024 Jun;24(6):407-421. doi: 10.1080/14737140.2024.2346187. Epub 2024 Apr 30. Expert Rev Anticancer Ther. 2024. PMID: 38682679 Review.
-
Recent advances in the molecular pathology of soft tissue sarcoma: implications for diagnosis, patient prognosis, and molecular target therapy in the future.Cancer Sci. 2009 Feb;100(2):200-8. doi: 10.1111/j.1349-7006.2008.01024.x. Cancer Sci. 2009. PMID: 19076980 Free PMC article. Review.
Cited by
-
Extracellular matrix regulation of cell spheroid invasion in a 3D bioprinted solid tumor-on-a-chip.Acta Biomater. 2024 Sep 15;186:156-166. doi: 10.1016/j.actbio.2024.07.040. Epub 2024 Aug 7. Acta Biomater. 2024. PMID: 39097123
References
-
- Stiller CA, Trama A, Serraino D, Rossi S, Navarro C, Chirlaque MD, et al. . Descriptive epidemiology of sarcomas in Europe: report from the RARECARE project. Eur J Cancer 2013;49:684–95. - PubMed
-
- Winograd-Katz SE, Fassler R, Geiger B, Legate KR. The integrin adhesome: from genes and proteins to human disease. Nat Rev Mol Cell Biol 2014;15:273–88. - PubMed
MeSH terms
Substances
Grants and funding
LinkOut - more resources
Full Text Sources
Medical
Research Materials