A Primer for Utilizing Deep Learning and Abdominal MRI Imaging Features to Monitor Autosomal Dominant Polycystic Kidney Disease Progression
- PMID: 38791095
- PMCID: PMC11118119
- DOI: 10.3390/biomedicines12051133
A Primer for Utilizing Deep Learning and Abdominal MRI Imaging Features to Monitor Autosomal Dominant Polycystic Kidney Disease Progression
Abstract
Abdominal imaging of autosomal dominant polycystic kidney disease (ADPKD) has historically focused on detecting complications such as cyst rupture, cyst infection, obstructing renal calculi, and pyelonephritis; discriminating complex cysts from renal cell carcinoma; and identifying sources of abdominal pain. Many imaging features of ADPKD are incompletely evaluated or not deemed to be clinically significant, and because of this, treatment options are limited. However, total kidney volume (TKV) measurement has become important for assessing the risk of disease progression (i.e., Mayo Imaging Classification) and predicting tolvaptan treatment's efficacy. Deep learning for segmenting the kidneys has improved these measurements' speed, accuracy, and reproducibility. Deep learning models can also segment other organs and tissues, extracting additional biomarkers to characterize the extent to which extrarenal manifestations complicate ADPKD. In this concept paper, we demonstrate how deep learning may be applied to measure the TKV and how it can be extended to measure additional features of this disease.
Keywords: autosomal dominant polycystic kidney disease; radiological report; semantic segmentation.
Conflict of interest statement
J.D.B. receives research support from Vertex Pharmaceuticals. D.S. reports consulting fees from Accordant and speaker fees from Otsuka. All other authors declare no conflicts of interest.
Figures
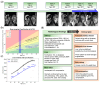
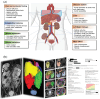
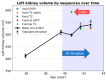
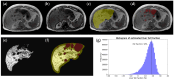
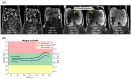
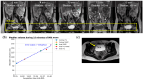
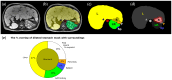
Similar articles
-
Assessing Risk of Rapid Progression in Autosomal Dominant Polycystic Kidney Disease and Special Considerations for Disease-Modifying Therapy.Am J Kidney Dis. 2021 Aug;78(2):282-292. doi: 10.1053/j.ajkd.2020.12.020. Epub 2021 Mar 8. Am J Kidney Dis. 2021. PMID: 33705818 Review.
-
Hemorrhagic Cysts and Other MR Biomarkers for Predicting Renal Dysfunction Progression in Autosomal Dominant Polycystic Kidney Disease.J Magn Reson Imaging. 2021 Feb;53(2):564-576. doi: 10.1002/jmri.27360. Epub 2020 Sep 23. J Magn Reson Imaging. 2021. PMID: 32969110
-
Deep learning-based segmentation of kidneys and renal cysts on T2-weighted MRI from patients with autosomal dominant polycystic kidney disease.Eur Radiol Exp. 2024 Oct 30;8(1):122. doi: 10.1186/s41747-024-00520-7. Eur Radiol Exp. 2024. PMID: 39477840 Free PMC article.
-
A Deep Learning Approach for Automated Segmentation of Kidneys and Exophytic Cysts in Individuals with Autosomal Dominant Polycystic Kidney Disease.J Am Soc Nephrol. 2022 Aug;33(8):1581-1589. doi: 10.1681/ASN.2021111400. Epub 2022 Jun 29. J Am Soc Nephrol. 2022. PMID: 35768178 Free PMC article.
-
Total Kidney Volume in Autosomal Dominant Polycystic Kidney Disease: A Biomarker of Disease Progression and Therapeutic Efficacy.Am J Kidney Dis. 2015 Oct;66(4):564-76. doi: 10.1053/j.ajkd.2015.01.030. Epub 2015 May 7. Am J Kidney Dis. 2015. PMID: 25960302 Review.
References
-
- Taylor J., Thomas R., Metherall P., van Gastel M., Cornec-Le Gall E., Caroli A., Furlano M., Demoulin N., Devuyst O., Winterbottom J., et al. An Artificial Intelligence Generated Automated Algorithm to Measure Total Kidney Volume in ADPKD. Kidney Int. Rep. 2024;9:249–256. doi: 10.1016/j.ekir.2023.10.029. - DOI - PMC - PubMed
Publication types
Grants and funding
LinkOut - more resources
Full Text Sources