Predicting the clinical subcutaneous absorption rate constant of monoclonal antibodies using only the primary sequence: a machine learning approach
- PMID: 38745390
- PMCID: PMC11110684
- DOI: 10.1080/19420862.2024.2352887
Predicting the clinical subcutaneous absorption rate constant of monoclonal antibodies using only the primary sequence: a machine learning approach
Abstract
Subcutaneous injections are an increasingly prevalent route of administration for delivering biological therapies including monoclonal antibodies (mAbs). Compared with intravenous delivery, subcutaneous injections reduce administration costs, shorten the administration time, and are strongly preferred from a patient experience point of view. An understanding of the absorption process of a mAb from the injection site to the systemic circulation is critical to the process of subcutaneous mAb formulation development. In this study, we built a model to predict the absorption rate constant (ka), which denotes how fast a mAb is absorbed from the site of administration. Once trained, our model (enabled by the XGBoost algorithm in machine learning) can predict the ka of a mAb following a subcutaneous injection using in silico molecular properties alone (generated from the primary sequence). Our model does not need clinically observed plasma concentration-time data; this is a novel capability not previously achieved in predictive pharmacokinetic models. The model also showed improved performance when benchmarked against a recently reported mechanistic model that relied on clinical data to predict subcutaneous absorption of mAbs. We further interpreted the model to understand which molecular properties affect the absorption rate and showed that our findings are consistent with previous studies evaluating subcutaneous absorption through direct experimentation. Taken altogether, this study reports the development, validation, benchmarking, and interpretation of a model that can predict the clinical ka of a mAb using its primary sequence as the only input.
Keywords: Machine learning; Xgboost; monoclonal antibody; rate constant; subcutaneous absorption.
Conflict of interest statement
No potential conflict of interest was reported by the author(s).
Figures
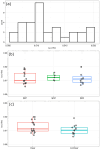
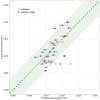
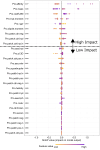
Similar articles
-
Influence of physiochemical properties on the subcutaneous absorption and bioavailability of monoclonal antibodies.MAbs. 2020 Jan 1;12(1):1770028. doi: 10.1080/19420862.2020.1770028. MAbs. 2020. PMID: 32486889 Free PMC article.
-
Predicting monoclonal antibody pharmacokinetics following subcutaneous administration via whole-body physiologically-based modeling.J Pharmacokinet Pharmacodyn. 2020 Oct;47(5):385-409. doi: 10.1007/s10928-020-09691-3. Epub 2020 Jun 4. J Pharmacokinet Pharmacodyn. 2020. PMID: 32500362 Free PMC article.
-
Subcutaneous delivery of monoclonal antibodies: How do we get there?J Control Release. 2018 Sep 28;286:301-314. doi: 10.1016/j.jconrel.2018.08.001. Epub 2018 Aug 2. J Control Release. 2018. PMID: 30077735 Review.
-
Simple Approach to Accurately Predict Pharmacokinetics of Therapeutic Monoclonal Antibodies after Subcutaneous Injection in Humans.Clin Pharmacokinet. 2021 Jan;60(1):111-120. doi: 10.1007/s40262-020-00917-8. Clin Pharmacokinet. 2021. PMID: 32779124
-
Subcutaneous Administration of Monoclonal Antibodies: Pharmacology, Delivery, Immunogenicity, and Learnings From Applications to Clinical Development.Clin Pharmacol Ther. 2024 Mar;115(3):422-439. doi: 10.1002/cpt.3150. Epub 2024 Jan 10. Clin Pharmacol Ther. 2024. PMID: 38093583 Review.
References
-
- Gabrielsson J, Weiner D, Weiner D. Pharmacokinetic & pharmacodynamic data analysis: concepts and applications. In: Gabrielsson J, editors. Expanded.; PK/PD data analysis: concepts and applications; Apotekarsocieteten, Swedish Acedemy of Pharmaceutical Sciences. Stockholm: Swedish Pharmaceutical Press; 2006. p. 43.
MeSH terms
Grants and funding
LinkOut - more resources
Full Text Sources