Streamlining Computational Fragment-Based Drug Discovery through Evolutionary Optimization Informed by Ligand-Based Virtual Prescreening
- PMID: 38696451
- PMCID: PMC11197033
- DOI: 10.1021/acs.jcim.4c00234
Streamlining Computational Fragment-Based Drug Discovery through Evolutionary Optimization Informed by Ligand-Based Virtual Prescreening
Abstract
Recent advances in computational methods provide the promise of dramatically accelerating drug discovery. While mathematical modeling and machine learning have become vital in predicting drug-target interactions and properties, there is untapped potential in computational drug discovery due to the vast and complex chemical space. This paper builds on our recently published computational fragment-based drug discovery (FBDD) method called fragment databases from screened ligand drug discovery (FDSL-DD). FDSL-DD uses in silico screening to identify ligands from a vast library, fragmenting them while attaching specific attributes based on predicted binding affinity and interaction with the target subdomain. In this paper, we further propose a two-stage optimization method that utilizes the information from prescreening to optimize computational ligand synthesis. We hypothesize that using prescreening information for optimization shrinks the search space and focuses on promising regions, thereby improving the optimization for candidate ligands. The first optimization stage assembles these fragments into larger compounds using genetic algorithms, followed by a second stage of iterative refinement to produce compounds with enhanced bioactivity. To demonstrate broad applicability, the methodology is demonstrated on three diverse protein targets found in human solid cancers, bacterial antimicrobial resistance, and the SARS-CoV-2 virus. Combined, the proposed FDSL-DD and a two-stage optimization approach yield high-affinity ligand candidates more efficiently than other state-of-the-art computational FBDD methods. We further show that a multiobjective optimization method accounting for drug-likeness can still produce potential candidate ligands with a high binding affinity. Overall, the results demonstrate that integrating detailed chemical information with a constrained search framework can markedly optimize the initial drug discovery process, offering a more precise and efficient route to developing new therapeutics.
Conflict of interest statement
The authors declare no competing financial interest.
Figures
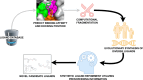
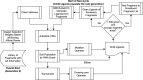
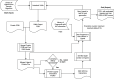
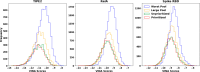
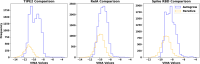
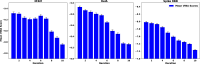
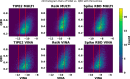
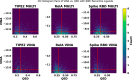
Similar articles
-
Fragment databases from screened ligands for drug discovery (FDSL-DD).J Mol Graph Model. 2024 Mar;127:108669. doi: 10.1016/j.jmgm.2023.108669. Epub 2023 Nov 22. J Mol Graph Model. 2024. PMID: 38011826
-
In silico fragment-based drug discovery: setup and validation of a fragment-to-lead computational protocol using S4MPLE.J Chem Inf Model. 2013 Apr 22;53(4):836-51. doi: 10.1021/ci4000163. Epub 2013 Apr 11. J Chem Inf Model. 2013. PMID: 23537132
-
Artificial intelligence in the prediction of protein-ligand interactions: recent advances and future directions.Brief Bioinform. 2022 Jan 17;23(1):bbab476. doi: 10.1093/bib/bbab476. Brief Bioinform. 2022. PMID: 34849575 Free PMC article. Review.
-
Machine learning in computational docking.Artif Intell Med. 2015 Mar;63(3):135-52. doi: 10.1016/j.artmed.2015.02.002. Epub 2015 Feb 16. Artif Intell Med. 2015. PMID: 25724101
-
Counting on Fragment Based Drug Design Approach for Drug Discovery.Curr Top Med Chem. 2018;18(27):2284-2293. doi: 10.2174/1568026619666181130134250. Curr Top Med Chem. 2018. PMID: 30499406 Review.
References
-
- Vieira T. F.; Sousa S. F. Comparing AutoDock and Vina in Ligand/Decoy Discrimination for Virtual Screening. Appl. Sci. 2019, 9, 4538.10.3390/app9214538. - DOI
Publication types
MeSH terms
Substances
LinkOut - more resources
Full Text Sources
Miscellaneous