Characterization of the genetic determinants of context-specific DNA methylation in primary monocytes
- PMID: 38663408
- PMCID: PMC11099345
- DOI: 10.1016/j.xgen.2024.100541
Characterization of the genetic determinants of context-specific DNA methylation in primary monocytes
Abstract
To better understand inter-individual variation in sensitivity of DNA methylation (DNAm) to immune activity, we characterized effects of inflammatory stimuli on primary monocyte DNAm (n = 190). We find that monocyte DNAm is site-dependently sensitive to lipopolysaccharide (LPS), with LPS-induced demethylation occurring following hydroxymethylation. We identify 7,359 high-confidence immune-modulated CpGs (imCpGs) that differ in genomic localization and transcription factor usage according to whether they represent a gain or loss in DNAm. Demethylated imCpGs are profoundly enriched for enhancers and colocalize to genes enriched for disease associations, especially cancer. DNAm is age associated, and we find that 24-h LPS exposure triggers approximately 6 months of gain in epigenetic age, directly linking epigenetic aging with innate immune activity. By integrating LPS-induced changes in DNAm with genetic variation, we identify 234 imCpGs under local genetic control. Exploring shared causal loci between LPS-induced DNAm responses and human disease traits highlights examples of disease-associated loci that modulate imCpG formation.
Keywords: DNA methylation; LPS; cancer; epigenetic aging; genetics; innate immune activation; mQTL; monocytes.
Copyright © 2024 The Authors. Published by Elsevier Inc. All rights reserved.
Conflict of interest statement
Declaration of interests The authors declare no competing interests.
Figures
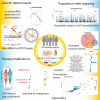
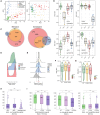
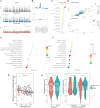
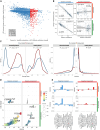
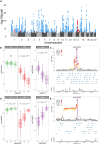
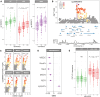
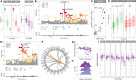
Similar articles
-
Epigenome-wide change and variation in DNA methylation in childhood: trajectories from birth to late adolescence.Hum Mol Genet. 2021 Mar 25;30(1):119-134. doi: 10.1093/hmg/ddaa280. Hum Mol Genet. 2021. PMID: 33450751 Free PMC article.
-
Systematic evaluation of DNA methylation age estimation with common preprocessing methods and the Infinium MethylationEPIC BeadChip array.Clin Epigenetics. 2018 Oct 16;10(1):123. doi: 10.1186/s13148-018-0556-2. Clin Epigenetics. 2018. PMID: 30326963 Free PMC article.
-
Evaluating DNA methylation age on the Illumina MethylationEPIC Bead Chip.PLoS One. 2019 Apr 19;14(4):e0207834. doi: 10.1371/journal.pone.0207834. eCollection 2019. PLoS One. 2019. PMID: 31002714 Free PMC article.
-
Common DNA methylation alterations of Alzheimer's disease and aging in peripheral whole blood.Oncotarget. 2016 Apr 12;7(15):19089-98. doi: 10.18632/oncotarget.7862. Oncotarget. 2016. PMID: 26943045 Free PMC article.
-
Smoking is Associated to DNA Methylation in Atherosclerotic Carotid Lesions.Circ Genom Precis Med. 2018 Sep;11(9):e002030. doi: 10.1161/CIRCGEN.117.002030. Circ Genom Precis Med. 2018. PMID: 30354327
References
-
- Kim-Hellmuth S., Bechheim M., Pütz B., Mohammadi P., Nédélec Y., Giangreco N., Becker J., Kaiser V., Fricker N., Beier E., et al. Genetic regulatory effects modified by immune activation contribute to autoimmune disease associations. Nat. Commun. 2017;8:266. doi: 10.1038/s41467-017-00366-1. - DOI - PMC - PubMed
Publication types
MeSH terms
Substances
LinkOut - more resources
Full Text Sources