Learning epistatic polygenic phenotypes with Boolean interactions
- PMID: 38625909
- PMCID: PMC11020961
- DOI: 10.1371/journal.pone.0298906
Learning epistatic polygenic phenotypes with Boolean interactions
Abstract
Detecting epistatic drivers of human phenotypes is a considerable challenge. Traditional approaches use regression to sequentially test multiplicative interaction terms involving pairs of genetic variants. For higher-order interactions and genome-wide large-scale data, this strategy is computationally intractable. Moreover, multiplicative terms used in regression modeling may not capture the form of biological interactions. Building on the Predictability, Computability, Stability (PCS) framework, we introduce the epiTree pipeline to extract higher-order interactions from genomic data using tree-based models. The epiTree pipeline first selects a set of variants derived from tissue-specific estimates of gene expression. Next, it uses iterative random forests (iRF) to search training data for candidate Boolean interactions (pairwise and higher-order). We derive significance tests for interactions, based on a stabilized likelihood ratio test, by simulating Boolean tree-structured null (no epistasis) and alternative (epistasis) distributions on hold-out test data. Finally, our pipeline computes PCS epistasis p-values that probabilisticly quantify improvement in prediction accuracy via bootstrap sampling on the test set. We validate the epiTree pipeline in two case studies using data from the UK Biobank: predicting red hair and multiple sclerosis (MS). In the case of predicting red hair, epiTree recovers known epistatic interactions surrounding MC1R and novel interactions, representing non-linearities not captured by logistic regression models. In the case of predicting MS, a more complex phenotype than red hair, epiTree rankings prioritize novel interactions surrounding HLA-DRB1, a variant previously associated with MS in several populations. Taken together, these results highlight the potential for epiTree rankings to help reduce the design space for follow up experiments.
Copyright: © 2024 Behr et al. This is an open access article distributed under the terms of the Creative Commons Attribution License, which permits unrestricted use, distribution, and reproduction in any medium, provided the original author and source are credited.
Conflict of interest statement
The authors have declared that no competing interests exist.
Figures
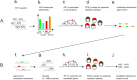
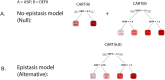
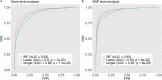
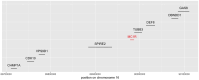
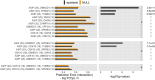
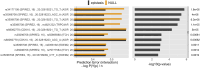
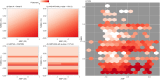
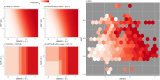
Similar articles
-
Gene, pathway and network frameworks to identify epistatic interactions of single nucleotide polymorphisms derived from GWAS data.BMC Syst Biol. 2012;6 Suppl 3(Suppl 3):S15. doi: 10.1186/1752-0509-6-S3-S15. Epub 2012 Dec 17. BMC Syst Biol. 2012. PMID: 23281810 Free PMC article.
-
Epi-MEIF: detecting higher order epistatic interactions for complex traits using mixed effect conditional inference forests.Nucleic Acids Res. 2022 Oct 28;50(19):e114. doi: 10.1093/nar/gkac715. Nucleic Acids Res. 2022. PMID: 36107776 Free PMC article.
-
Detecting epistasis with the marginal epistasis test in genetic mapping studies of quantitative traits.PLoS Genet. 2017 Jul 26;13(7):e1006869. doi: 10.1371/journal.pgen.1006869. eCollection 2017 Jul. PLoS Genet. 2017. PMID: 28746338 Free PMC article.
-
Genetics of Alzheimer's Disease: the Importance of Polygenic and Epistatic Components.Curr Neurol Neurosci Rep. 2017 Aug 21;17(10):78. doi: 10.1007/s11910-017-0787-1. Curr Neurol Neurosci Rep. 2017. PMID: 28825204 Free PMC article. Review.
-
Analysis pipeline for the epistasis search - statistical versus biological filtering.Front Genet. 2014 Apr 30;5:106. doi: 10.3389/fgene.2014.00106. eCollection 2014. Front Genet. 2014. PMID: 24817878 Free PMC article. Review.
Cited by
-
A blood-based metabolomic signature predictive of risk for pancreatic cancer.Cell Rep Med. 2023 Sep 19;4(9):101194. doi: 10.1016/j.xcrm.2023.101194. Cell Rep Med. 2023. PMID: 37729870 Free PMC article.
-
Detecting gene-gene interactions from GWAS using diffusion kernel principal components.BMC Bioinformatics. 2022 Feb 1;23(1):57. doi: 10.1186/s12859-022-04580-7. BMC Bioinformatics. 2022. PMID: 35105309 Free PMC article.
-
Patterns of Fitness and Gene Expression Epistasis Generated by Beneficial Mutations in the rho and rpoB Genes of Escherichia coli during High-Temperature Adaptation.Mol Biol Evol. 2024 Sep 4;41(9):msae187. doi: 10.1093/molbev/msae187. Mol Biol Evol. 2024. PMID: 39235107 Free PMC article.
References
-
- Bateson W. Mendel’s Principles of Heredity. Cambridge Univ. Press; 1909.
-
- Ritchie MD. Finding the Epistasis Needles in the Genome-Wide Haystack. In: Epistasis. Methods in Molecular Biology (Methods and Protocols). vol. 1253. New York: Humana Press; 2015. p. 19–33. - PubMed