Analysis and experimental validation of IL-17 pathway and key genes as central roles associated with inflammation in hepatic ischemia-reperfusion injury
- PMID: 38494504
- PMCID: PMC10944831
- DOI: 10.1038/s41598-024-57139-2
Analysis and experimental validation of IL-17 pathway and key genes as central roles associated with inflammation in hepatic ischemia-reperfusion injury
Abstract
Hepatic ischemia-reperfusion injury (HIRI) elicits an immune-inflammatory response that may result in hepatocyte necrosis and apoptosis, ultimately culminating in postoperative hepatic dysfunction and hepatic failure. The precise mechanisms governing the pathophysiology of HIRI remain incompletely understood, necessitating further investigation into key molecules and pathways implicated in disease progression to guide drug discovery and potential therapeutic interventions. Gene microarray data was downloaded from the GEO expression profile database. Integrated bioinformatic analyses were performed to identify HIRI signature genes, which were subsequently validated for expression levels and diagnostic efficacy. Finally, the gene expression was verified in an experimental HIRI model and the effect of anti-IL17A antibody intervention in three time points (including pre-ischemic, post-ischemic, and at 1 h of reperfusion) on HIRI and the expression of these genes was investigated. Bioinformatic analyses of the screened characterized genes revealed that inflammation, immune response, and cell death modulation were significantly associated with HIRI pathophysiology. CCL2, BTG2, GADD45A, FOS, CXCL10, TNFRSF12A, and IL-17 pathway were identified as key components involved in the HIRI. Serum and liver IL-17A expression were significantly upregulated during the initial phase of HIRI. Pretreatment with anti-IL-17A antibody effectively alleviated the damage of liver tissue, suppressed inflammatory factors, and serum transaminase levels, and downregulated the mRNA expression of CCL2, GADD45A, FOS, CXCL10, and TNFRSF12A. Injection of anti-IL17A antibody after ischemia and at 1 h of reperfusion failed to demonstrate anti-inflammatory and attenuating HIRI benefits relative to earlier intervention. Our study reveals that the IL-17 pathway and related genes may be involved in the proinflammatory mechanism of HIRI, which may provide a new perspective and theoretical basis for the prevention and treatment of HIRI.
Keywords: Bioinformatics; Chemokines; Cytokines; Hepatic ischemia–reperfusion injury; IL-17; Inflammation.
© 2024. The Author(s).
Conflict of interest statement
The authors declare no competing interests.
Figures
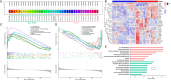
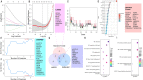
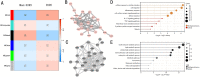
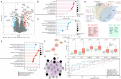
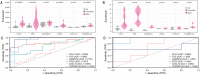
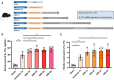
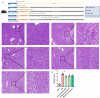
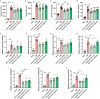
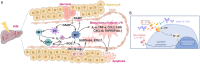
Similar articles
-
New progress in roles of nitric oxide during hepatic ischemia reperfusion injury.World J Gastroenterol. 2017 Apr 14;23(14):2505-2510. doi: 10.3748/wjg.v23.i14.2505. World J Gastroenterol. 2017. PMID: 28465634 Free PMC article. Review.
-
Exosome-related gene identification and diagnostic model construction in hepatic ischemia-reperfusion injury.Sci Rep. 2024 Sep 28;14(1):22450. doi: 10.1038/s41598-024-73441-5. Sci Rep. 2024. PMID: 39341981 Free PMC article.
-
MCTR1 inhibits ferroptosis by promoting NRF2 expression to attenuate hepatic ischemia-reperfusion injury.Am J Physiol Gastrointest Liver Physiol. 2022 Sep 1;323(3):G283-G293. doi: 10.1152/ajpgi.00354.2021. Epub 2022 Aug 2. Am J Physiol Gastrointest Liver Physiol. 2022. PMID: 35916424
-
Isoflurane upregulates microRNA-9-3p to protect rats from hepatic ischemia-reperfusion injury through inhibiting fibronectin type III domain containing 3B.Cell Cycle. 2021 Aug;20(16):1527-1539. doi: 10.1080/15384101.2021.1947548. Epub 2021 Jul 25. Cell Cycle. 2021. PMID: 34308776 Free PMC article.
-
Targeting NF-κB in Hepatic Ischemia-Reperfusion Alleviation: from Signaling Networks to Therapeutic Targeting.Mol Neurobiol. 2024 Jun;61(6):3409-3426. doi: 10.1007/s12035-023-03787-w. Epub 2023 Nov 22. Mol Neurobiol. 2024. PMID: 37991700 Review.
References
MeSH terms
Substances
Grants and funding
LinkOut - more resources
Full Text Sources
Medical