Combining machine learning with structure-based protein design to predict and engineer post-translational modifications of proteins
- PMID: 38484014
- PMCID: PMC10965067
- DOI: 10.1371/journal.pcbi.1011939
Combining machine learning with structure-based protein design to predict and engineer post-translational modifications of proteins
Abstract
Post-translational modifications (PTMs) of proteins play a vital role in their function and stability. These modifications influence protein folding, signaling, protein-protein interactions, enzyme activity, binding affinity, aggregation, degradation, and much more. To date, over 400 types of PTMs have been described, representing chemical diversity well beyond the genetically encoded amino acids. Such modifications pose a challenge to the successful design of proteins, but also represent a major opportunity to diversify the protein engineering toolbox. To this end, we first trained artificial neural networks (ANNs) to predict eighteen of the most abundant PTMs, including protein glycosylation, phosphorylation, methylation, and deamidation. In a second step, these models were implemented inside the computational protein modeling suite Rosetta, which allows flexible combination with existing protocols to model the modified sites and understand their impact on protein stability as well as function. Lastly, we developed a new design protocol that either maximizes or minimizes the predicted probability of a particular site being modified. We find that this combination of ANN prediction and structure-based design can enable the modification of existing, as well as the introduction of novel, PTMs. The potential applications of our work include, but are not limited to, glycan masking of epitopes, strengthening protein-protein interactions through phosphorylation, as well as protecting proteins from deamidation liabilities. These applications are especially important for the design of new protein therapeutics where PTMs can drastically change the therapeutic properties of a protein. Our work adds novel tools to Rosetta's protein engineering toolbox that allow for the rational design of PTMs.
Copyright: © 2024 Ertelt et al. This is an open access article distributed under the terms of the Creative Commons Attribution License, which permits unrestricted use, distribution, and reproduction in any medium, provided the original author and source are credited.
Conflict of interest statement
The authors have declared that no competing interests exist.
Figures
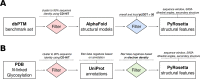
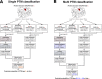
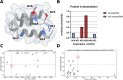
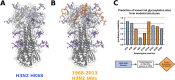
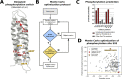
Similar articles
-
A machine learning strategy for predicting localization of post-translational modification sites in protein-protein interacting regions.BMC Bioinformatics. 2016 Aug 17;17(1):307. doi: 10.1186/s12859-016-1165-8. BMC Bioinformatics. 2016. PMID: 27534850 Free PMC article.
-
A Text Mining and Machine Learning Protocol for Extracting Posttranslational Modifications of Proteins from PubMed: A Special Focus on Glycosylation, Acetylation, Methylation, Hydroxylation, and Ubiquitination.Methods Mol Biol. 2022;2496:179-202. doi: 10.1007/978-1-0716-2305-3_10. Methods Mol Biol. 2022. PMID: 35713865
-
Current status of PTMs structural databases: applications, limitations and prospects.Amino Acids. 2022 Apr;54(4):575-590. doi: 10.1007/s00726-021-03119-z. Epub 2022 Jan 12. Amino Acids. 2022. PMID: 35020020 Review.
-
Novel Post-translational Modifications in Human Serum Albumin.Protein Pept Lett. 2022;29(5):473-484. doi: 10.2174/0929866529666220318152509. Protein Pept Lett. 2022. PMID: 35306981
-
Post-translational modifications in the Protein Data Bank.Acta Crystallogr D Struct Biol. 2024 Sep 1;80(Pt 9):647-660. doi: 10.1107/S2059798324007794. Epub 2024 Aug 29. Acta Crystallogr D Struct Biol. 2024. PMID: 39207896 Free PMC article. Review.
Cited by
-
Combining Rosetta Sequence Design with Protein Language Model Predictions Using Evolutionary Scale Modeling (ESM) as Restraint.ACS Synth Biol. 2024 Apr 19;13(4):1085-1092. doi: 10.1021/acssynbio.3c00753. Epub 2024 Apr 3. ACS Synth Biol. 2024. PMID: 38568188 Free PMC article.
-
Current computational tools for protein lysine acylation site prediction.Brief Bioinform. 2024 Sep 23;25(6):bbae469. doi: 10.1093/bib/bbae469. Brief Bioinform. 2024. PMID: 39316944
References
-
- Schwarz F, Aebi M. Mechanisms and principles of N-linked protein glycosylation. Current Opinion in Structural Biology 2011;21:576–582. - PubMed
-
- Hart GW, Haltiwanger RS, Holt GD, Kelly WG. Nucleoplasmic and cytoplasmic glycoproteins. In Ciba Foundation Symposium 145-Carbohydrate Recognition in Cellular Function: Carbohydrate Recognition in Cellular Function: Ciba Foundation Symposium 145; 2007, 102–18. - PubMed
MeSH terms
Substances
Grants and funding
LinkOut - more resources
Full Text Sources
Miscellaneous