Integrative temporal multi-omics reveals uncoupling of transcriptome and proteome during human T cell activation
- PMID: 38418561
- PMCID: PMC10901835
- DOI: 10.1038/s41540-024-00346-4
Integrative temporal multi-omics reveals uncoupling of transcriptome and proteome during human T cell activation
Abstract
Engagement of the T cell receptor (TCR) triggers molecular reprogramming leading to the acquisition of specialized effector functions by CD4 helper and CD8 cytotoxic T cells. While transcription factors, chemokines, and cytokines are known drivers in this process, the temporal proteomic and transcriptomic changes that regulate different stages of human primary T cell activation remain to be elucidated. Here, we report an integrative temporal proteomic and transcriptomic analysis of primary human CD4 and CD8 T cells following ex vivo stimulation with anti-CD3/CD28 beads, which revealed major transcriptome-proteome uncoupling. The early activation phase in both CD4 and CD8 T cells was associated with transient downregulation of the mRNA transcripts and protein of the central glucose transport GLUT1. In the proliferation phase, CD4 and CD8 T cells became transcriptionally more divergent while their proteome became more similar. In addition to the kinetics of proteome-transcriptome correlation, this study unveils selective transcriptional and translational metabolic reprogramming governing CD4 and CD8 T cell responses to TCR stimulation. This temporal transcriptome/proteome map of human T cell activation provides a reference map exploitable for future discovery of biomarkers and candidates targeting T cell responses.
© 2024. The Author(s).
Conflict of interest statement
The authors declare no competing interests.
Figures
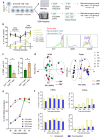
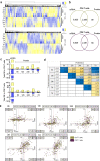
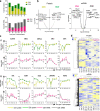
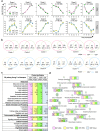
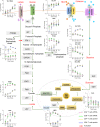
Similar articles
-
Extensive proteomic and transcriptomic changes quench the TCR/CD3 activation signal of latently HIV-1 infected T cells.PLoS Pathog. 2021 Jan 19;17(1):e1008748. doi: 10.1371/journal.ppat.1008748. eCollection 2021 Jan. PLoS Pathog. 2021. PMID: 33465149 Free PMC article.
-
NSOM/QD-based direct visualization of CD3-induced and CD28-enhanced nanospatial coclustering of TCR and coreceptor in nanodomains in T cell activation.PLoS One. 2009 Jun 17;4(6):e5945. doi: 10.1371/journal.pone.0005945. PLoS One. 2009. PMID: 19536289 Free PMC article.
-
4-1BB ligand induces cell division, sustains survival, and enhances effector function of CD4 and CD8 T cells with similar efficacy.J Immunol. 2001 Aug 1;167(3):1313-24. doi: 10.4049/jimmunol.167.3.1313. J Immunol. 2001. PMID: 11466348
-
Interplay between the TCR/CD3 complex and CD4 or CD8 in the activation of cytotoxic T lymphocytes.Immunol Rev. 1989 Jun;109:119-41. doi: 10.1111/j.1600-065x.1989.tb00022.x. Immunol Rev. 1989. PMID: 2527803 Review.
-
Antigen-specific suppressor T lymphocytes in man.Clin Immunol Immunopathol. 1989 Nov;53(2 Pt 2):S17-24. doi: 10.1016/0090-1229(89)90066-4. Clin Immunol Immunopathol. 1989. PMID: 2551552 Review.
References
MeSH terms
Substances
Grants and funding
LinkOut - more resources
Full Text Sources
Research Materials
Miscellaneous