Large-Scale Proteome Profiling Identifies Biomarkers Associated with Suspected Neurosyphilis Diagnosis
- PMID: 38380496
- PMCID: PMC11040343
- DOI: 10.1002/advs.202307744
Large-Scale Proteome Profiling Identifies Biomarkers Associated with Suspected Neurosyphilis Diagnosis
Abstract
Neurosyphilis (NS) is a central nervous system (CNS) infection caused by Treponema pallidum (T. pallidum). NS can occur at any stage of syphilis and manifests as a broad spectrum of clinical symptoms. Often referred to as "the great imitator," NS can be easily overlooked or misdiagnosed due to the absence of standard diagnostic tests, potentially leading to severe and irreversible organ dysfunction. In this study, proteomic and machine learning model techniques are used to characterize 223 cerebrospinal fluid (CSF) samples to identify diagnostic markers of NS and provide insights into the underlying mechanisms of the associated inflammatory responses. Three biomarkers (SEMA7A, SERPINA3, and ITIH4) are validated as contributors to NS diagnosis through multicenter verification of an additional 115 CSF samples. We anticipate that the identified biomarkers will become effective tools for assisting in diagnosis of NS. Our insights into NS pathogenesis in brain tissue may inform therapeutic strategies and drug discoveries for NS patients.
Keywords: cerebrospinal fluid; diagnostic biomarker; machine learning model; neurosyphilis; proteomics.
© 2024 The Authors. Advanced Science published by Wiley‐VCH GmbH.
Conflict of interest statement
The authors declare no conflict of interest.
Figures
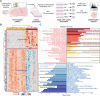
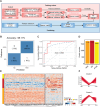
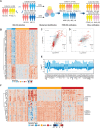
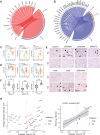
Similar articles
-
Multicentric evaluation of a specific intrathecal anti-Treponema pallidum IgG index as a diagnostic biomarker of neurosyphilis: results from a retro-prospective case-control study.Sex Transm Infect. 2024 Feb 19;100(2):63-69. doi: 10.1136/sextrans-2023-055913. Sex Transm Infect. 2024. PMID: 38071543
-
Intrathecal Synthesis Index of Specific Anti-Treponema IgG: a New Tool for the Diagnosis of Neurosyphilis.Microbiol Spectr. 2022 Feb 23;10(1):e0147721. doi: 10.1128/spectrum.01477-21. Epub 2022 Feb 9. Microbiol Spectr. 2022. PMID: 35138118 Free PMC article.
-
Clinical and Biological Characteristics of 40 Patients With Neurosyphilis and Evaluation of Treponema pallidum Nested Polymerase Chain Reaction in Cerebrospinal Fluid Samples.Clin Infect Dis. 2016 Nov 1;63(9):1180-1186. doi: 10.1093/cid/ciw499. Epub 2016 Sep 1. Clin Infect Dis. 2016. PMID: 27585981 Clinical Trial.
-
Chemokine Ligand 13 (CXCL13) in Neuroborreliosis and Neurosyphilis as Selected Spirochetal Neurological Diseases: A Review of Its Diagnostic Significance.Int J Mol Sci. 2020 Apr 22;21(8):2927. doi: 10.3390/ijms21082927. Int J Mol Sci. 2020. PMID: 32331231 Free PMC article. Review.
-
Neurosyphilis.Infect Dis Clin North Am. 1994 Dec;8(4):769-95. Infect Dis Clin North Am. 1994. PMID: 7890932 Review.
References
Publication types
MeSH terms
Substances
Grants and funding
- 82341079/National Natural Science Foundation of China
- 32270697/National Natural Science Foundation of China
- 2021YFA1301603/National Key Research Program of China
- Z231100007223009/Beijing Science and Technology Planning Project
- 2023-I2M-3-002/Chinese Academy of Medical Sciences Initiative for Innovative Medicine
- 2023-I2M-QJ-001/Chinese Academy of Medical Sciences Initiative for Innovative Medicine
- 2020-I2M-C&T-B-048/Chinese Academy of Medical Sciences Initiative for Innovative Medicine
- 2022-PUMCH-A-022/Peking Union Medical College Hospital
- 2022-PUMCH-B-092/Peking Union Medical College Hospital
- 2022-PUMCH-B-061/Peking Union Medical College Hospital
LinkOut - more resources
Full Text Sources
Miscellaneous