Accelerating computer vision-based human identification through the integration of deep learning-based age estimation from 2 to 89 years
- PMID: 38379027
- PMCID: PMC10879188
- DOI: 10.1038/s41598-024-54877-1
Accelerating computer vision-based human identification through the integration of deep learning-based age estimation from 2 to 89 years
Abstract
Computer Vision (CV)-based human identification using orthopantomograms (OPGs) has the potential to identify unknown deceased individuals by comparing postmortem OPGs with a comprehensive antemortem CV database. However, the growing size of the CV database leads to longer processing times. This study aims to develop a standardized and reliable Convolutional Neural Network (CNN) for age estimation using OPGs and integrate it into the CV-based human identification process. The CNN was trained on 50,000 OPGs, each labeled with ages ranging from 2 to 89 years. Testing included three postmortem OPGs, 10,779 antemortem OPGs, and an additional set of 70 OPGs within the context of CV-based human identification. Integrating the CNN for age estimation into CV-based human identification process resulted in a substantial reduction of up to 96% in processing time for a CV database containing 105,251 entries. Age estimation accuracy varied between postmortem and antemortem OPGs, with a mean absolute error (MAE) of 2.76 ± 2.67 years and 3.26 ± 3.06 years across all ages, as well as 3.69 ± 3.14 years for an additional 70 OPGs. In conclusion, the incorporation of a CNN for age estimation in the CV-based human identification process significantly reduces processing time while delivering reliable results.
© 2024. The Author(s).
Conflict of interest statement
The author declares no competing interests.
Figures
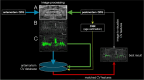
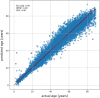
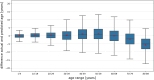
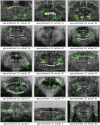
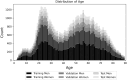
Similar articles
-
Semi-supervised automatic dental age and sex estimation using a hybrid transformer model.Int J Legal Med. 2023 May;137(3):721-731. doi: 10.1007/s00414-023-02956-9. Epub 2023 Jan 31. Int J Legal Med. 2023. PMID: 36717384
-
OPG-based dental age estimation using a data-technical exploration of deep learning techniques.J Forensic Sci. 2024 May;69(3):919-931. doi: 10.1111/1556-4029.15473. Epub 2024 Jan 30. J Forensic Sci. 2024. PMID: 38291770
-
Forensic Odontology: Automatic Identification of Persons Comparing Antemortem and Postmortem Panoramic Radiographs Using Computer Vision.Rofo. 2018 Dec;190(12):1152-1158. doi: 10.1055/a-0632-4744. Epub 2018 Jun 18. Rofo. 2018. PMID: 29913524 English.
-
Image-based laparoscopic tool detection and tracking using convolutional neural networks: a review of the literature.Comput Assist Surg (Abingdon). 2020 Dec;25(1):15-28. doi: 10.1080/24699322.2020.1801842. Comput Assist Surg (Abingdon). 2020. PMID: 32886540 Review.
-
3D fully convolutional networks for subcortical segmentation in MRI: A large-scale study.Neuroimage. 2018 Apr 15;170:456-470. doi: 10.1016/j.neuroimage.2017.04.039. Epub 2017 Apr 24. Neuroimage. 2018. PMID: 28450139 Review.
Cited by
-
Reply to Letter to the Editor: "Can single CT slices revolutionize personal identification in emergency settings?".Eur Radiol. 2024 Oct 28. doi: 10.1007/s00330-024-11131-6. Online ahead of print. Eur Radiol. 2024. PMID: 39466396 No abstract available.
-
Automatic personal identification using a single CT image.Eur Radiol. 2024 Aug 22. doi: 10.1007/s00330-024-11013-x. Online ahead of print. Eur Radiol. 2024. PMID: 39172245
References
-
- INTERPOL. Disaster victim identification guide 2018. (Diakses).
-
- Rötzscher K. Forensische Zahnmedizin: Forensische Odonto-Stomatologie. Springer-Verlag; 2013.
-
- Aliyev, R., Arslanoglu, E., Yasa, Y. & Oktay, A. B. Age Estimation from Pediatric Panoramic Dental Images with CNNs and LightGBM. 2022 Medical Technologies Congress (TIPTEKNO), 1–4 (2022).
MeSH terms
Grants and funding
LinkOut - more resources
Full Text Sources