Artificial intelligence and database for NGS-based diagnosis in rare disease
- PMID: 38371307
- PMCID: PMC10870236
- DOI: 10.3389/fgene.2023.1258083
Artificial intelligence and database for NGS-based diagnosis in rare disease
Abstract
Rare diseases (RDs) are rare complex genetic diseases affecting a conservative estimate of 300 million people worldwide. Recent Next-Generation Sequencing (NGS) studies are unraveling the underlying genetic heterogeneity of this group of diseases. NGS-based methods used in RDs studies have improved the diagnosis and management of RDs. Concomitantly, a suite of bioinformatics tools has been developed to sort through big data generated by NGS to understand RDs better. However, there are concerns regarding the lack of consistency among different methods, primarily linked to factors such as the lack of uniformity in input and output formats, the absence of a standardized measure for predictive accuracy, and the regularity of updates to the annotation database. Today, artificial intelligence (AI), particularly deep learning, is widely used in a variety of biological contexts, changing the healthcare system. AI has demonstrated promising capabilities in boosting variant calling precision, refining variant prediction, and enhancing the user-friendliness of electronic health record (EHR) systems in NGS-based diagnostics. This paper reviews the state of the art of AI in NGS-based genetics, and its future directions and challenges. It also compare several rare disease databases.
Keywords: artificial intelligence; data science; diagnosis; machine learning; next-generation sequencing; rare disease.
Copyright © 2024 Choon, Choon, Nasarudin, Al Jasmi, Remli, Alkayali and Mohamad.
Conflict of interest statement
The authors declare that the research was conducted in the absence of any commercial or financial relationships that could be construed as a potential conflict of interest.
Figures
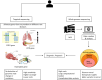
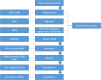
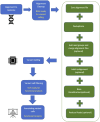
Similar articles
-
Artificial intelligence (AI) and big data in cancer and precision oncology.Comput Struct Biotechnol J. 2020 Aug 28;18:2300-2311. doi: 10.1016/j.csbj.2020.08.019. eCollection 2020. Comput Struct Biotechnol J. 2020. PMID: 32994889 Free PMC article. Review.
-
Machine learning random forest for predicting oncosomatic variant NGS analysis.Sci Rep. 2021 Nov 8;11(1):21820. doi: 10.1038/s41598-021-01253-y. Sci Rep. 2021. PMID: 34750410 Free PMC article.
-
Diagnosis of a Single-Nucleotide Variant in Whole-Exome Sequencing Data for Patients With Inherited Diseases: Machine Learning Study Using Artificial Intelligence Variant Prioritization.JMIR Bioinform Biotechnol. 2022 Sep 15;3(1):e37701. doi: 10.2196/37701. JMIR Bioinform Biotechnol. 2022. PMID: 38935959 Free PMC article.
-
Artificial Intelligence in Epigenetic Studies: Shedding Light on Rare Diseases.Front Mol Biosci. 2021 May 5;8:648012. doi: 10.3389/fmolb.2021.648012. eCollection 2021. Front Mol Biosci. 2021. PMID: 34026829 Free PMC article. Review.
-
Artificial Intelligence (AI) in Rare Diseases: Is the Future Brighter?Genes (Basel). 2019 Nov 27;10(12):978. doi: 10.3390/genes10120978. Genes (Basel). 2019. PMID: 31783696 Free PMC article. Review.
References
-
- Amorim B. R., Santos P. A. C. D., Lima C. L. D., Andia D. C., Mazzeu J. F., Acevedo A. C. (2019). “Protocols for genetic and epigenetic studies of rare diseases affecting dental tissues,” in Odontogenesis (New York, NY: Humana Press; ), 453–492. - PubMed
Publication types
Grants and funding
LinkOut - more resources
Full Text Sources