This is a preprint.
A functional genomic framework to elucidate novel causal non-alcoholic fatty liver disease genes
- PMID: 38352379
- PMCID: PMC10863038
- DOI: 10.1101/2024.02.03.24302258
A functional genomic framework to elucidate novel causal non-alcoholic fatty liver disease genes
Update in
-
A functional genomic framework to elucidate novel causal metabolic dysfunction-associated fatty liver disease genes.Hepatology. 2024 Aug 27. doi: 10.1097/HEP.0000000000001066. Online ahead of print. Hepatology. 2024. PMID: 39190705
Abstract
Background & aims: Non-alcoholic fatty liver disease (NAFLD) is the most prevalent chronic liver pathology in western countries, with serious public health consequences. Efforts to identify causal genes for NAFLD have been hampered by the relative paucity of human data from gold-standard magnetic resonance quantification of hepatic fat. To overcome insufficient sample size, genome-wide association studies using NAFLD surrogate phenotypes have been used, but only a small number of loci have been identified to date. In this study, we combined GWAS of NAFLD composite surrogate phenotypes with genetic colocalization studies followed by functional in vitro screens to identify bona fide causal genes for NAFLD.
Approach & results: We used the UK Biobank to explore the associations of our novel NAFLD score, and genetic colocalization to prioritize putative causal genes for in vitro validation. We created a functional genomic framework to study NAFLD genes in vitro using CRISPRi. Our data identify VKORC1, TNKS, LYPLAL1 and GPAM as regulators of lipid accumulation in hepatocytes and suggest the involvement of VKORC1 in the lipid storage related to the development of NAFLD.
Conclusions: Complementary genetic and genomic approaches are useful for the identification of NAFLD genes. Our data supports VKORC1 as a bona fide NAFLD gene. We have established a functional genomic framework to study at scale putative novel NAFLD genes from human genetic association studies.
Keywords: CRISPR interference; HepaRG cells; NAFLD; Perturb-seq; genetic epidemiology.
Figures
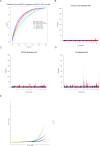
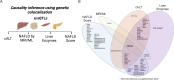
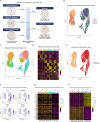
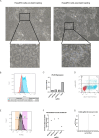
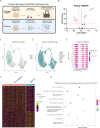
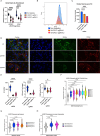
Similar articles
-
A functional genomic framework to elucidate novel causal metabolic dysfunction-associated fatty liver disease genes.Hepatology. 2024 Aug 27. doi: 10.1097/HEP.0000000000001066. Online ahead of print. Hepatology. 2024. PMID: 39190705
-
The hepato-ovarian axis: genetic evidence for a causal association between non-alcoholic fatty liver disease and polycystic ovary syndrome.BMC Med. 2023 Feb 20;21(1):62. doi: 10.1186/s12916-023-02775-0. BMC Med. 2023. PMID: 36800955 Free PMC article.
-
Disease-specific eQTL screening reveals an anti-fibrotic effect of AGXT2 in non-alcoholic fatty liver disease.J Hepatol. 2021 Sep;75(3):514-523. doi: 10.1016/j.jhep.2021.04.011. Epub 2021 Apr 20. J Hepatol. 2021. PMID: 33892010
-
From sugar to liver fat and public health: systems biology driven studies in understanding non-alcoholic fatty liver disease pathogenesis.Proc Nutr Soc. 2019 Aug;78(3):290-304. doi: 10.1017/S0029665119000570. Epub 2019 Mar 29. Proc Nutr Soc. 2019. PMID: 30924429 Review.
-
Genetic and epigenetic disease modifiers: non-alcoholic fatty liver disease (NAFLD) and alcoholic liver disease (ALD).Transl Gastroenterol Hepatol. 2021 Jan 5;6:2. doi: 10.21037/tgh.2019.09.06. eCollection 2021. Transl Gastroenterol Hepatol. 2021. PMID: 33409397 Free PMC article. Review.
References
-
- Duell PB, Welty FK, Miller M, Chait A, Hammond G, Ahmad Z, et al. Nonalcoholic Fatty Liver Disease and Cardiovascular Risk: A Scientific Statement From the American Heart Association. Arterioscler Thromb Vasc Biol. 2022;42:e168–e185. - PubMed
Publication types
Grants and funding
LinkOut - more resources
Full Text Sources
Research Materials