Network propagation for GWAS analysis: a practical guide to leveraging molecular networks for disease gene discovery
- PMID: 38340090
- PMCID: PMC10858647
- DOI: 10.1093/bib/bbae014
Network propagation for GWAS analysis: a practical guide to leveraging molecular networks for disease gene discovery
Abstract
Motivation: Genome-wide association studies (GWAS) have enabled large-scale analysis of the role of genetic variants in human disease. Despite impressive methodological advances, subsequent clinical interpretation and application remains challenging when GWAS suffer from a lack of statistical power. In recent years, however, the use of information diffusion algorithms with molecular networks has led to fruitful insights on disease genes.
Results: We present an overview of the design choices and pitfalls that prove crucial in the application of network propagation methods to GWAS summary statistics. We highlight general trends from the literature, and present benchmark experiments to expand on these insights selecting as case study three diseases and five molecular networks. We verify that the use of gene-level scores based on GWAS P-values offers advantages over the selection of a set of 'seed' disease genes not weighted by the associated P-values if the GWAS summary statistics are of sufficient quality. Beyond that, the size and the density of the networks prove to be important factors for consideration. Finally, we explore several ensemble methods and show that combining multiple networks may improve the network propagation approach.
Keywords: GWAS; disease gene; molecular network; network propagation.
© The Author(s) 2024. Published by Oxford University Press.
Figures
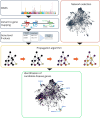
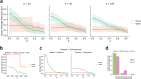
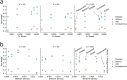
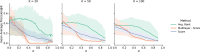
Similar articles
-
Leveraging multiple gene networks to prioritize GWAS candidate genes via network representation learning.Methods. 2018 Aug 1;145:41-50. doi: 10.1016/j.ymeth.2018.06.002. Epub 2018 Jun 3. Methods. 2018. PMID: 29874547
-
Identification of susceptibility modules for coronary artery disease using a genome wide integrated network analysis.Gene. 2013 Dec 1;531(2):347-54. doi: 10.1016/j.gene.2013.08.059. Epub 2013 Aug 29. Gene. 2013. PMID: 23994195
-
Prioritizing candidate disease genes by network-based boosting of genome-wide association data.Genome Res. 2011 Jul;21(7):1109-21. doi: 10.1101/gr.118992.110. Epub 2011 May 2. Genome Res. 2011. PMID: 21536720 Free PMC article.
-
Genetic study of complex diseases in the post-GWAS era.J Genet Genomics. 2015 Mar 20;42(3):87-98. doi: 10.1016/j.jgg.2015.02.001. Epub 2015 Feb 13. J Genet Genomics. 2015. PMID: 25819085 Review.
-
Genetics of coronary artery disease in the post-GWAS era.J Intern Med. 2021 Nov;290(5):980-992. doi: 10.1111/joim.13362. Epub 2021 Aug 23. J Intern Med. 2021. PMID: 34237186 Review.
References
-
- Ghosh R, Tabrizi SJ. Clinical features of Huntington’s disease. Adv Exp Med Biol 2018; 1049:1–28. - PubMed
-
- Hirschhorn JN, Daly MJ. Genome-wide association studies for common diseases and complex traits. Nat Rev Genet 2005; 6(2): 95–108. - PubMed
-
- Hirschhorn JN. Genetic approaches to studying common diseases and complex traits. Pediatr Res 2005; 57:74R–7. - PubMed