De novo protein design-From new structures to programmable functions
- PMID: 38306980
- PMCID: PMC10990048
- DOI: 10.1016/j.cell.2023.12.028
De novo protein design-From new structures to programmable functions
Abstract
Methods from artificial intelligence (AI) trained on large datasets of sequences and structures can now "write" proteins with new shapes and molecular functions de novo, without starting from proteins found in nature. In this Perspective, I will discuss the state of the field of de novo protein design at the juncture of physics-based modeling approaches and AI. New protein folds and higher-order assemblies can be designed with considerable experimental success rates, and difficult problems requiring tunable control over protein conformations and precise shape complementarity for molecular recognition are coming into reach. Emerging approaches incorporate engineering principles-tunability, controllability, and modularity-into the design process from the beginning. Exciting frontiers lie in deconstructing cellular functions with de novo proteins and, conversely, constructing synthetic cellular signaling from the ground up. As methods improve, many more challenges are unsolved.
Keywords: artificial intelligence; de novo protein design; deep learning; synthetic signaling.
Copyright © 2024 The Author. Published by Elsevier Inc. All rights reserved.
Conflict of interest statement
Declaration of interests The author declares no competing interests.
Figures
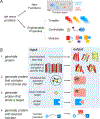
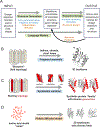
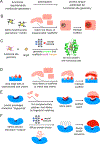
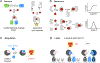
Similar articles
-
Generative artificial intelligence for de novo protein design.Curr Opin Struct Biol. 2024 Jun;86:102794. doi: 10.1016/j.sbi.2024.102794. Epub 2024 Apr 24. Curr Opin Struct Biol. 2024. PMID: 38663170 Review.
-
De Novo Design of Polyhedral Protein Assemblies: Before and After the AI Revolution.Chembiochem. 2023 Aug 1;24(15):e202300117. doi: 10.1002/cbic.202300117. Epub 2023 Jul 12. Chembiochem. 2023. PMID: 37014094 Review.
-
Revolutionizing Molecular Design for Innovative Therapeutic Applications through Artificial Intelligence.Molecules. 2024 Sep 29;29(19):4626. doi: 10.3390/molecules29194626. Molecules. 2024. PMID: 39407556 Free PMC article. Review.
-
Artificial Intelligence in De novo Drug Design: Are We Still There?Curr Top Med Chem. 2022;22(30):2483-2492. doi: 10.2174/1568026623666221017143244. Curr Top Med Chem. 2022. PMID: 36263480 Review.
-
Deep Learning Applied to Ligand-Based De Novo Drug Design.Methods Mol Biol. 2022;2390:273-299. doi: 10.1007/978-1-0716-1787-8_12. Methods Mol Biol. 2022. PMID: 34731474 Review.
Cited by
-
Rationally seeded computational protein design of ɑ-helical barrels.Nat Chem Biol. 2024 Aug;20(8):991-999. doi: 10.1038/s41589-024-01642-0. Epub 2024 Jun 20. Nat Chem Biol. 2024. PMID: 38902458 Free PMC article.
-
Applications of cell free protein synthesis in protein design.Protein Sci. 2024 Sep;33(9):e5148. doi: 10.1002/pro.5148. Protein Sci. 2024. PMID: 39180484 Free PMC article. Review.
-
Folds from fold: Exploring topological isoforms of a single-domain protein.Proc Natl Acad Sci U S A. 2024 Oct 22;121(43):e2407355121. doi: 10.1073/pnas.2407355121. Epub 2024 Oct 15. Proc Natl Acad Sci U S A. 2024. PMID: 39405345
-
SCUBA-D: a freshly trained diffusion model generates high-quality protein structures.Nat Methods. 2024 Nov;21(11):1990-1991. doi: 10.1038/s41592-024-02465-6. Nat Methods. 2024. PMID: 39468213 No abstract available.
-
An integrative approach to protein sequence design through multiobjective optimization.PLoS Comput Biol. 2024 Jul 11;20(7):e1011953. doi: 10.1371/journal.pcbi.1011953. eCollection 2024 Jul. PLoS Comput Biol. 2024. PMID: 38991035 Free PMC article.
References
Publication types
MeSH terms
Substances
Grants and funding
LinkOut - more resources
Full Text Sources
Research Materials