Deep learning for protein structure prediction and design-progress and applications
- PMID: 38291232
- PMCID: PMC10912668
- DOI: 10.1038/s44320-024-00016-x
Deep learning for protein structure prediction and design-progress and applications
Abstract
Proteins are the key molecular machines that orchestrate all biological processes of the cell. Most proteins fold into three-dimensional shapes that are critical for their function. Studying the 3D shape of proteins can inform us of the mechanisms that underlie biological processes in living cells and can have practical applications in the study of disease mutations or the discovery of novel drug treatments. Here, we review the progress made in sequence-based prediction of protein structures with a focus on applications that go beyond the prediction of single monomer structures. This includes the application of deep learning methods for the prediction of structures of protein complexes, different conformations, the evolution of protein structures and the application of these methods to protein design. These developments create new opportunities for research that will have impact across many areas of biomedical research.
Keywords: AlphaFold2; Protein Conformations; Protein Design; Structural Bioinformatics; Structural Systems Biology.
© 2024. The Author(s).
Conflict of interest statement
The authors declare no competing interests.
Figures
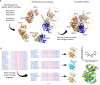
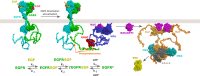
Similar articles
-
Recent Applications of Deep Learning Methods on Evolution- and Contact-Based Protein Structure Prediction.Int J Mol Sci. 2021 Jun 2;22(11):6032. doi: 10.3390/ijms22116032. Int J Mol Sci. 2021. PMID: 34199677 Free PMC article. Review.
-
Deep learning-driven insights into super protein complexes for outer membrane protein biogenesis in bacteria.Elife. 2022 Dec 28;11:e82885. doi: 10.7554/eLife.82885. Elife. 2022. PMID: 36576775 Free PMC article.
-
[Advances in the application of AlphaFold2: a protein structure prediction model].Sheng Wu Gong Cheng Xue Bao. 2024 May 25;40(5):1406-1420. doi: 10.13345/j.cjb.230677. Sheng Wu Gong Cheng Xue Bao. 2024. PMID: 38783805 Review. Chinese.
-
Single-sequence protein structure prediction using a language model and deep learning.Nat Biotechnol. 2022 Nov;40(11):1617-1623. doi: 10.1038/s41587-022-01432-w. Epub 2022 Oct 3. Nat Biotechnol. 2022. PMID: 36192636 Free PMC article.
-
Artificial intelligence in fusion protein three-dimensional structure prediction: Review and perspective.Clin Transl Med. 2024 Aug;14(8):e1789. doi: 10.1002/ctm2.1789. Clin Transl Med. 2024. PMID: 39090739 Free PMC article. Review.
Cited by
-
Allostery Illuminated: Harnessing AI and Machine Learning for Drug Discovery.ACS Med Chem Lett. 2024 Aug 30;15(9):1449-1455. doi: 10.1021/acsmedchemlett.4c00260. eCollection 2024 Sep 12. ACS Med Chem Lett. 2024. PMID: 39291033 Review.
-
Global atlas of predicted functional domains in Legionella pneumophila Dot/Icm translocated effectors.Mol Syst Biol. 2025 Jan;21(1):59-89. doi: 10.1038/s44320-024-00076-z. Epub 2024 Nov 19. Mol Syst Biol. 2025. PMID: 39562741 Free PMC article.
References
-
- Ahdritz G, Bouatta N, Kadyan S, Xia Q, Gerecke W, O’Donnell TJ, Berenberg D, Fisk I, Zanichelli N, Zhang B et al (2022) OpenFold: retraining AlphaFold2 yields new insights into its learning mechanisms and capacity for generalization. Preprint at bioRxiv 10.1101/2022.11.20.517210 - PubMed
Publication types
MeSH terms
Substances
LinkOut - more resources
Full Text Sources