Integrated drug response prediction models pinpoint repurposed drugs with effectiveness against rhabdomyosarcoma
- PMID: 38277404
- PMCID: PMC10817174
- DOI: 10.1371/journal.pone.0295629
Integrated drug response prediction models pinpoint repurposed drugs with effectiveness against rhabdomyosarcoma
Abstract
Targeted therapies for inhibiting the growth of cancer cells or inducing apoptosis are urgently needed for effective rhabdomyosarcoma (RMS) treatment. However, identifying cancer-targeting compounds with few side effects, among the many potential compounds, is expensive and time-consuming. A computational approach to reduce the number of potential candidate drugs can facilitate the discovery of attractive lead compounds. To address this and obtain reliable predictions of novel cell-line-specific drugs, we apply prediction models that have the potential to improve drug discovery approaches for RMS treatment. The results of two prediction models were ensemble and validated via in vitro experiments. The computational models were trained using data extracted from the Genomics of Drug Sensitivity in Cancer database and tested on two RMS cell lines to select potential RMS drug candidates. Among 235 candidate drugs, 22 were selected following the result of the computational approach, and three candidate drugs were identified (NSC207895, vorinostat, and belinostat) that showed selective effectiveness in RMS cell lines in vitro via the induction of apoptosis. Our in vitro experiments have demonstrated that our proposed methods can effectively identify and repurpose drugs for treating RMS.
Copyright: © 2024 Baek et al. This is an open access article distributed under the terms of the Creative Commons Attribution License, which permits unrestricted use, distribution, and reproduction in any medium, provided the original author and source are credited.
Conflict of interest statement
The authors have declared that no competing interests exist.
Figures
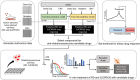
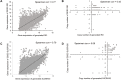
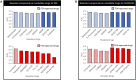

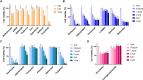
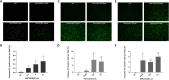
Similar articles
-
In vitro effects and ex vivo binding of an EGFR-specific immunotoxin on rhabdomyosarcoma cells.J Cancer Res Clin Oncol. 2015 Jun;141(6):1049-61. doi: 10.1007/s00432-014-1884-z. Epub 2014 Nov 30. J Cancer Res Clin Oncol. 2015. PMID: 25433506
-
Arsenic trioxide induces Noxa-dependent apoptosis in rhabdomyosarcoma cells and synergizes with antimicrotubule drugs.Cancer Lett. 2016 Oct 28;381(2):287-95. doi: 10.1016/j.canlet.2016.07.007. Epub 2016 Aug 10. Cancer Lett. 2016. PMID: 27521572
-
Rapid liposomal formulation for nucleolin targeting to rhabdomyosarcoma cells.Eur J Pharm Biopharm. 2024 Jan;194:49-61. doi: 10.1016/j.ejpb.2023.11.020. Epub 2023 Nov 27. Eur J Pharm Biopharm. 2024. PMID: 38029941
-
Engaging Cell Death Pathways for the Treatment of Rhabdomyosarcoma.Crit Rev Oncog. 2016;21(3-4):221-239. doi: 10.1615/CritRevOncog.2016016996. Crit Rev Oncog. 2016. PMID: 27915973 Review.
-
Therapeutic Approaches Targeting PAX3-FOXO1 and Its Regulatory and Transcriptional Pathways in Rhabdomyosarcoma.Molecules. 2018 Oct 28;23(11):2798. doi: 10.3390/molecules23112798. Molecules. 2018. PMID: 30373318 Free PMC article. Review.
Cited by
-
Crossfeat: a transformer-based cross-feature learning model for predicting drug side effect frequency.BMC Bioinformatics. 2024 Oct 8;25(1):324. doi: 10.1186/s12859-024-05915-2. BMC Bioinformatics. 2024. PMID: 39379821 Free PMC article.
References
-
- Peng W, Chen T, Dai W. Predicting drug response based on multi-omics fusion and graph convolution. IEEE Journal of Biomedical and Health Informatics. 2021;26(3):1384–93. - PubMed
MeSH terms
Grants and funding
LinkOut - more resources
Full Text Sources