This is a preprint.
De Novo Atomic Protein Structure Modeling for Cryo-EM Density Maps Using 3D Transformer and Hidden Markov Model
- PMID: 38260535
- PMCID: PMC10802328
- DOI: 10.1101/2024.01.02.573943
De Novo Atomic Protein Structure Modeling for Cryo-EM Density Maps Using 3D Transformer and Hidden Markov Model
Update in
-
De novo atomic protein structure modeling for cryoEM density maps using 3D transformer and HMM.Nat Commun. 2024 Jun 29;15(1):5511. doi: 10.1038/s41467-024-49647-6. Nat Commun. 2024. PMID: 38951555 Free PMC article.
Abstract
Accurately building three-dimensional (3D) atomic structures from 3D cryo-electron microscopy (cryo-EM) density maps is a crucial step in the cryo-EM-based determination of the structures of protein complexes. Despite improvements in the resolution of 3D cryo-EM density maps, the de novo conversion of density maps into 3D atomic structures for protein complexes that do not have accurate homologous or predicted structures to be used as templates remains a significant challenge. Here, we introduce Cryo2Struct, a fully automated ab initio cryo-EM structure modeling method that utilizes a 3D transformer to identify atoms and amino acid types in cryo-EM density maps first, and then employs a novel Hidden Markov Model (HMM) to connect predicted atoms to build backbone structures of proteins. Tested on a standard test dataset of 128 cryo-EM density maps with varying resolutions (2.1 - 5.6 °A) and different numbers of residues (730 - 8,416), Cryo2Struct built substantially more accurate and complete protein structural models than the widely used ab initio method - Phenix in terms of multiple evaluation metrics. Moreover, on a new test dataset of 500 recently released density maps with varying resolutions (1.9 - 4.0 °A) and different numbers of residues (234 - 8,828), it built more accurate models than on the standard dataset. And its performance is rather robust against the change of the resolution of density maps and the size of protein structures.
Keywords: Hidden Markov Model; atomic protein structure modeling; cryo-EM; deep learning; transformer.
Conflict of interest statement
Conflict of interest. The authors declare no conflict of interest.
Figures
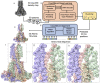
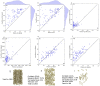
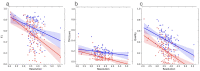
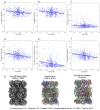
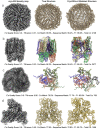
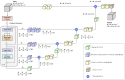
Similar articles
-
De novo atomic protein structure modeling for cryoEM density maps using 3D transformer and HMM.Nat Commun. 2024 Jun 29;15(1):5511. doi: 10.1038/s41467-024-49647-6. Nat Commun. 2024. PMID: 38951555 Free PMC article.
-
Deep Learning for Validating and Estimating Resolution of Cryo-Electron Microscopy Density Maps †.Molecules. 2019 Mar 26;24(6):1181. doi: 10.3390/molecules24061181. Molecules. 2019. PMID: 30917528 Free PMC article.
-
Full-length de novo protein structure determination from cryo-EM maps using deep learning.Bioinformatics. 2021 Oct 25;37(20):3480-3490. doi: 10.1093/bioinformatics/btab357. Bioinformatics. 2021. PMID: 33978686
-
Determining the Crystal Structure of TRPV6.In: Kozak JA, Putney JW Jr, editors. Calcium Entry Channels in Non-Excitable Cells. Boca Raton (FL): CRC Press/Taylor & Francis; 2018. Chapter 14. In: Kozak JA, Putney JW Jr, editors. Calcium Entry Channels in Non-Excitable Cells. Boca Raton (FL): CRC Press/Taylor & Francis; 2018. Chapter 14. PMID: 30299652 Free Books & Documents. Review.
-
Deep learning for reconstructing protein structures from cryo-EM density maps: Recent advances and future directions.Curr Opin Struct Biol. 2023 Apr;79:102536. doi: 10.1016/j.sbi.2023.102536. Epub 2023 Feb 9. Curr Opin Struct Biol. 2023. PMID: 36773336 Free PMC article. Review.
References
-
- Soltanikazemi Elham, Roy Raj S, Qua dir Farhan, Giri Nabin, Morehead Alex, and Cheng Jianlin. Drlcomplex: Reconstruction of protein quaternary structures using deep reinforcement learning. arXiv preprint arXiv:2205.13594, 2022.
-
- Bai Xiao-Chen, McMullan Greg, and Scheres Sjors HW. How cryo-em is revolutionizing structural biology. Trends in biochemical sciences, 40(1):49–57, 2015. - PubMed
Publication types
Grants and funding
LinkOut - more resources
Full Text Sources