Targeting ion channels with ultra-large library screening for hit discovery
- PMID: 38249296
- PMCID: PMC10796734
- DOI: 10.3389/fnmol.2023.1336004
Targeting ion channels with ultra-large library screening for hit discovery
Abstract
Ion channels play a crucial role in a variety of physiological and pathological processes, making them attractive targets for drug development in diseases such as diabetes, epilepsy, hypertension, cancer, and chronic pain. Despite the importance of ion channels in drug discovery, the vastness of chemical space and the complexity of ion channels pose significant challenges for identifying drug candidates. The use of in silico methods in drug discovery has dramatically reduced the time and cost of drug development and has the potential to revolutionize the field of medicine. Recent advances in computer hardware and software have enabled the screening of ultra-large compound libraries. Integration of different methods at various scales and dimensions is becoming an inevitable trend in drug development. In this review, we provide an overview of current state-of-the-art computational chemistry methodologies for ultra-large compound library screening and their application to ion channel drug discovery research. We discuss the advantages and limitations of various in silico techniques, including virtual screening, molecular mechanics/dynamics simulations, and machine learning-based approaches. We also highlight several successful applications of computational chemistry methodologies in ion channel drug discovery and provide insights into future directions and challenges in this field.
Keywords: chemical library; deep learning; drug design; ion channels; virtual screening.
Copyright © 2024 Melancon, Pliushcheuskaya, Meiler and Künze.
Conflict of interest statement
The authors declare that the research was conducted in the absence of any commercial or financial relationships that could be construed as a potential conflict of interest.
Figures
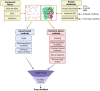
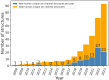
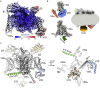
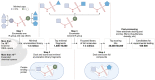
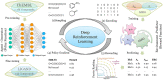
Similar articles
-
Ionic channels as targets for drug design: a review on computational methods.Pharmaceutics. 2011 Dec 9;3(4):932-53. doi: 10.3390/pharmaceutics3040932. Pharmaceutics. 2011. PMID: 24309315 Free PMC article.
-
Recent applications of deep learning and machine intelligence on in silico drug discovery: methods, tools and databases.Brief Bioinform. 2019 Sep 27;20(5):1878-1912. doi: 10.1093/bib/bby061. Brief Bioinform. 2019. PMID: 30084866 Free PMC article. Review.
-
Computational Ion Channel Research: from the Application of Artificial Intelligence to Molecular Dynamics Simulations.Cell Physiol Biochem. 2021 Mar 3;55(S3):14-45. doi: 10.33594/000000336. Cell Physiol Biochem. 2021. PMID: 33656309 Review.
-
From bench to bedside, via desktop. Recent advances in the application of cutting-edge in silico tools in the research of drugs targeting bromodomain modules.Biochem Pharmacol. 2019 Jan;159:40-51. doi: 10.1016/j.bcp.2018.11.007. Epub 2018 Nov 9. Biochem Pharmacol. 2019. PMID: 30414936 Review.
-
Advances in the discovery of new chemotypes through ultra-large library docking.Expert Opin Drug Discov. 2023 Mar;18(3):303-313. doi: 10.1080/17460441.2023.2171984. Epub 2023 Feb 2. Expert Opin Drug Discov. 2023. PMID: 36714919 Review.
References
-
- AurSCOPE Ion Channel Database (n.d.) . Available online at: https://www.aureus-pharma.com (accessed October 27, 2023)
Publication types
Grants and funding
LinkOut - more resources
Full Text Sources