Single-cell transcriptomics of the immune system in ME/CFS at baseline and following symptom provocation
- PMID: 38232699
- PMCID: PMC10829790
- DOI: 10.1016/j.xcrm.2023.101373
Single-cell transcriptomics of the immune system in ME/CFS at baseline and following symptom provocation
Abstract
Myalgic encephalomyelitis/chronic fatigue syndrome (ME/CFS) is a serious and poorly understood disease. To understand immune dysregulation in ME/CFS, we use single-cell RNA sequencing (scRNA-seq) to examine immune cells in patient and control cohorts. Postexertional malaise (PEM), an exacerbation of symptoms following strenuous exercise, is a characteristic symptom of ME/CFS. To detect changes coincident with PEM, we applied scRNA-seq on the same cohorts following exercise. At baseline, ME/CFS patients display classical monocyte dysregulation suggestive of inappropriate differentiation and migration to tissue. We identify both diseased and more normal monocytes within patients, and the fraction of diseased cells correlates with disease severity. Comparing the transcriptome at baseline and postexercise challenge, we discover patterns indicative of improper platelet activation in patients, with minimal changes elsewhere in the immune system. Taken together, these data identify immunological defects present at baseline in patients and an additional layer of dysregulation in platelets.
Keywords: CPET; exercise challenge; long COVID; monocytes; myalgic encephalomyelitis/chronic fatigue syndrome (ME/CFS); platelets; scRNA-seq.
Copyright © 2023 The Authors. Published by Elsevier Inc. All rights reserved.
Conflict of interest statement
Declaration of interests The authors declare no competing interests.
Figures
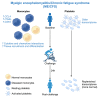
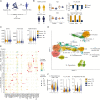
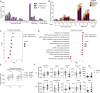
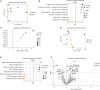
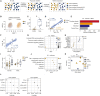
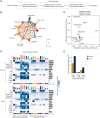
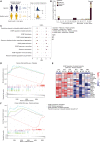
Similar articles
-
Whole blood human transcriptome and virome analysis of ME/CFS patients experiencing post-exertional malaise following cardiopulmonary exercise testing.PLoS One. 2019 Mar 21;14(3):e0212193. doi: 10.1371/journal.pone.0212193. eCollection 2019. PLoS One. 2019. PMID: 30897114 Free PMC article.
-
Recovery from Exercise in Persons with Myalgic Encephalomyelitis/Chronic Fatigue Syndrome (ME/CFS).Medicina (Kaunas). 2023 Mar 15;59(3):571. doi: 10.3390/medicina59030571. Medicina (Kaunas). 2023. PMID: 36984572 Free PMC article.
-
Stress-Induced Transcriptomic Changes in Females with Myalgic Encephalomyelitis/Chronic Fatigue Syndrome Reveal Disrupted Immune Signatures.Int J Mol Sci. 2023 Jan 31;24(3):2698. doi: 10.3390/ijms24032698. Int J Mol Sci. 2023. PMID: 36769022 Free PMC article.
-
Exercise Pathophysiology in Myalgic Encephalomyelitis/Chronic Fatigue Syndrome and Postacute Sequelae of SARS-CoV-2: More in Common Than Not?Chest. 2023 Sep;164(3):717-726. doi: 10.1016/j.chest.2023.03.049. Epub 2023 Apr 11. Chest. 2023. PMID: 37054777 Free PMC article. Review.
-
The Prospects of the Two-Day Cardiopulmonary Exercise Test (CPET) in ME/CFS Patients: A Meta-Analysis.J Clin Med. 2020 Dec 14;9(12):4040. doi: 10.3390/jcm9124040. J Clin Med. 2020. PMID: 33327624 Free PMC article. Review.
Cited by
-
Data-independent LC-MS/MS analysis of ME/CFS plasma reveals a dysregulated coagulation system, endothelial dysfunction, downregulation of complement machinery.Cardiovasc Diabetol. 2024 Jul 16;23(1):254. doi: 10.1186/s12933-024-02315-x. Cardiovasc Diabetol. 2024. PMID: 39014464 Free PMC article.
-
Thromboinflammation in long COVID-the elusive key to postinfection sequelae?J Thromb Haemost. 2023 Aug;21(8):2020-2031. doi: 10.1016/j.jtha.2023.04.039. Epub 2023 May 11. J Thromb Haemost. 2023. PMID: 37178769 Free PMC article. Review.
-
Dysregulation of extracellular vesicle protein cargo in female myalgic encephalomyelitis/chronic fatigue syndrome cases and sedentary controls in response to maximal exercise.J Extracell Vesicles. 2024 Jan;13(1):e12403. doi: 10.1002/jev2.12403. J Extracell Vesicles. 2024. PMID: 38173127 Free PMC article.
-
Herpesvirus Infection of Endothelial Cells as a Systemic Pathological Axis in Myalgic Encephalomyelitis/Chronic Fatigue Syndrome.Viruses. 2024 Apr 8;16(4):572. doi: 10.3390/v16040572. Viruses. 2024. PMID: 38675914 Free PMC article. Review.
-
Proteomics and cytokine analyses distinguish myalgic encephalomyelitis/chronic fatigue syndrome cases from controls.J Transl Med. 2023 May 13;21(1):322. doi: 10.1186/s12967-023-04179-3. J Transl Med. 2023. PMID: 37179299 Free PMC article.
References
Publication types
MeSH terms
Grants and funding
LinkOut - more resources
Full Text Sources
Medical
Molecular Biology Databases
Research Materials